Intelligent Marine Survey: Lightweight Multi-Scale Attention Adaptive Segmentation Framework for Underwater Target Detection of AUV
IEEE TRANSACTIONS ON AUTOMATION SCIENCE AND ENGINEERING(2024)
摘要
Accurate and automatic underwater target recognition is a compelling challenge for autonomous underwater vehicles (AUVs) in intelligent marine surveys. This study proposed a seabed target correction model based on side-scan sonar (SSS) images and combined the navigation information of AUV to achieve pixel-level geocoding. Moreover, a lightweight multi-level attention adaptive segmentation framework (MA(2)Net) was proposed to achieve fine-grained recognition. It contains three new modules: 1) The lightweight attention network (LAN) is designed as the baseline to obtain dense feature maps and focus on interesting features based on a balanced attention mechanism. 2) the multi-scale feature pyramid (MASPP) was then constructed to capture the context of SSS images and extract rich semantic information at high levels. 3) Finally, the adaptive feature fusion module (AFF) effectively incorporates feature maps of MASPP and spatial information to improve the learned representations further. Extensive experiments are verified on six SSS categories and show the remarkable performance of the MA(2)Net compared with state-of-the-art methods. Furthermore, real sea trials were conducted by deploying MA(2)Net to the autonomous target recognition (ATR) system of AUV, which can achieve 29.7 fps and 81.23% MIoU for a (512x 512) input on a single Nvidia Jetson Xavier. Note to Practitioners-This paper aims to provide a real-time semantic segmentation model for the autonomous target detection of AUV, which is suitable for the autonomous detection of underwater targets by underwater robots (ROV, AUV, ARV, et al). This paper proposes a lightweight, multi-scale attention-adaptive segmentation framework (MA(2)Net) incorporating pixel-level seabed targets rectification methods. The algorithm has high segmentation accuracy and fast operation speed. It can identify seabed targets in high-resolution sonar images online and realize precise positioning of small seabed targets, which is conducive to improving the intelligence level of marine survey unmanned equipment. This paper details the design of MA(2)Net and the hardware structure of the autonomous target recognition system (ATR). Plenty of simulation experiments and sea trials have proved the efficiency and practicability of the method for the autonomous detection of different seabed targets (sand waves, coral reefs, metal balls, threads, and artificial reefs). Future research will verify the generalization of the algorithm in more seabed targets.
更多查看译文
关键词
Feature extraction,Sonar,Real-time systems,Surveys,Semantics,Target recognition,Semantic segmentation,Autonomous underwater vehicle,underwater targets recognition,side-scan sonar,real-time semantic segmentation
AI 理解论文
溯源树
样例
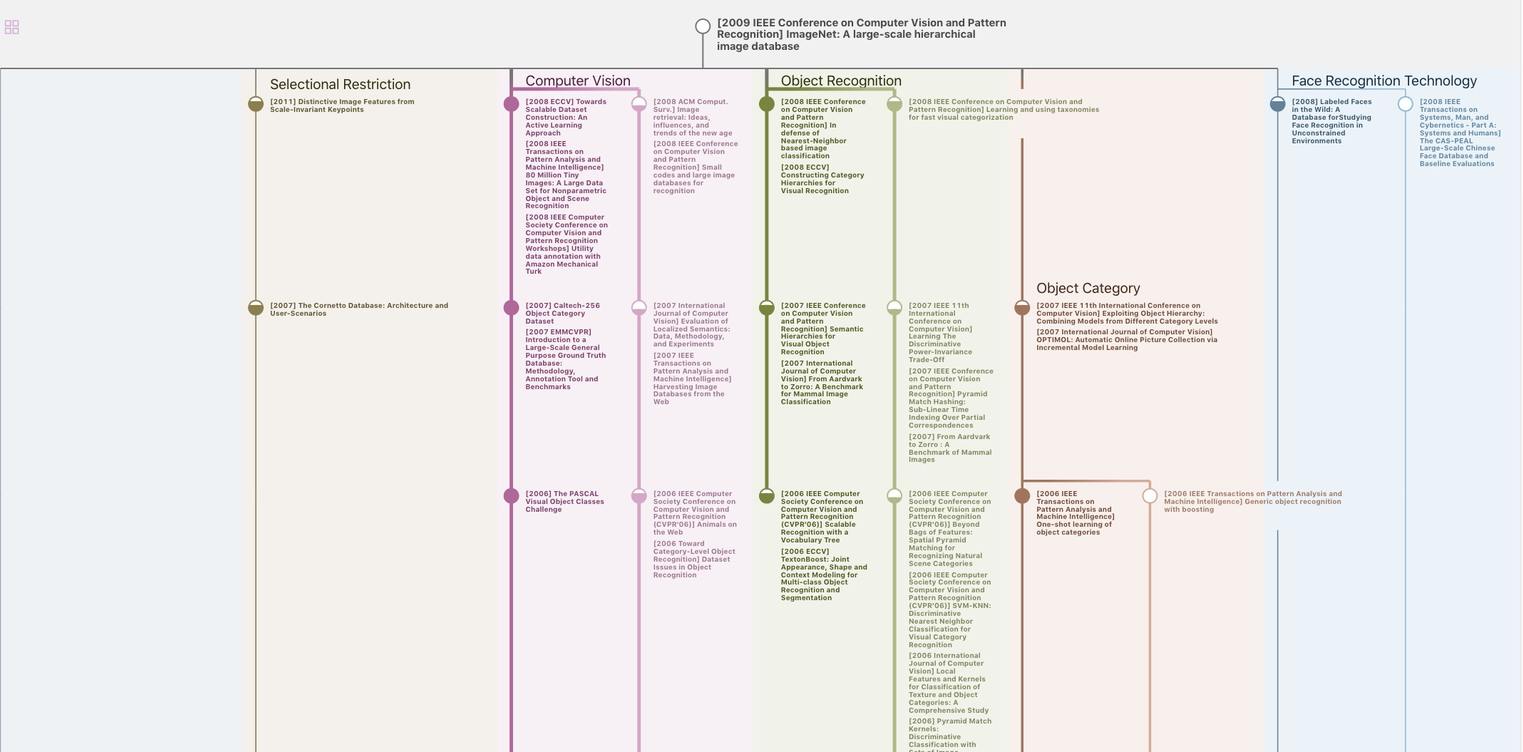
生成溯源树,研究论文发展脉络
Chat Paper
正在生成论文摘要