A New End-to-End Monitoring Framework for Nonlinear Dynamic Processes With Unknown Noise Statistics
IEEE TRANSACTIONS ON INSTRUMENTATION AND MEASUREMENT(2024)
摘要
Process monitoring (PM) plays an important role in industrial intelligent maintenance. However, existing PM methods usually assume that the noise satisfies Gaussian or non-Gaussian, making it difficult to deal with monitoring data contaminated by noise with unknown statistics in nonlinear dynamic processes. If these methods are used directly, there will be a certain number of undetectable areas. To address this issue, we propose a new end-to-end monitoring framework by integrating a recurrent neural network (RNN) with complementary dual residual generators, which to the best of our knowledge has not been investigated in PM. Technically, the pre residual generator targets to capture the potential dynamics and nonlinear relationships but may generate non-Gaussian residuals, while the post residual generator targets to complement the pre residual generator by training with a negentropy loss function. It is guaranteed that although the noise statistics are unknown, the generated outputs can be combined into a residual satisfying the Gaussian distribution. Hence, we can easily monitor processes using the T-2 test statistics. Extensive numerical examples with unknown noise statistics, including the simulated Tennessee Eastman (TE) process and a real-world marine diesel engine (DE) process, demonstrate the Gaussianity of generated residuals and verify the superiority compared with state-of-the-art methods.
更多查看译文
关键词
Generators,Monitoring,Recurrent neural networks,Nonlinear dynamical systems,Principal component analysis,Vectors,Kernel,Complementary dual residual generator,nonlinear dynamic processes,process monitoring (PM),recurrent neural network (RNN),unknown noise statistics
AI 理解论文
溯源树
样例
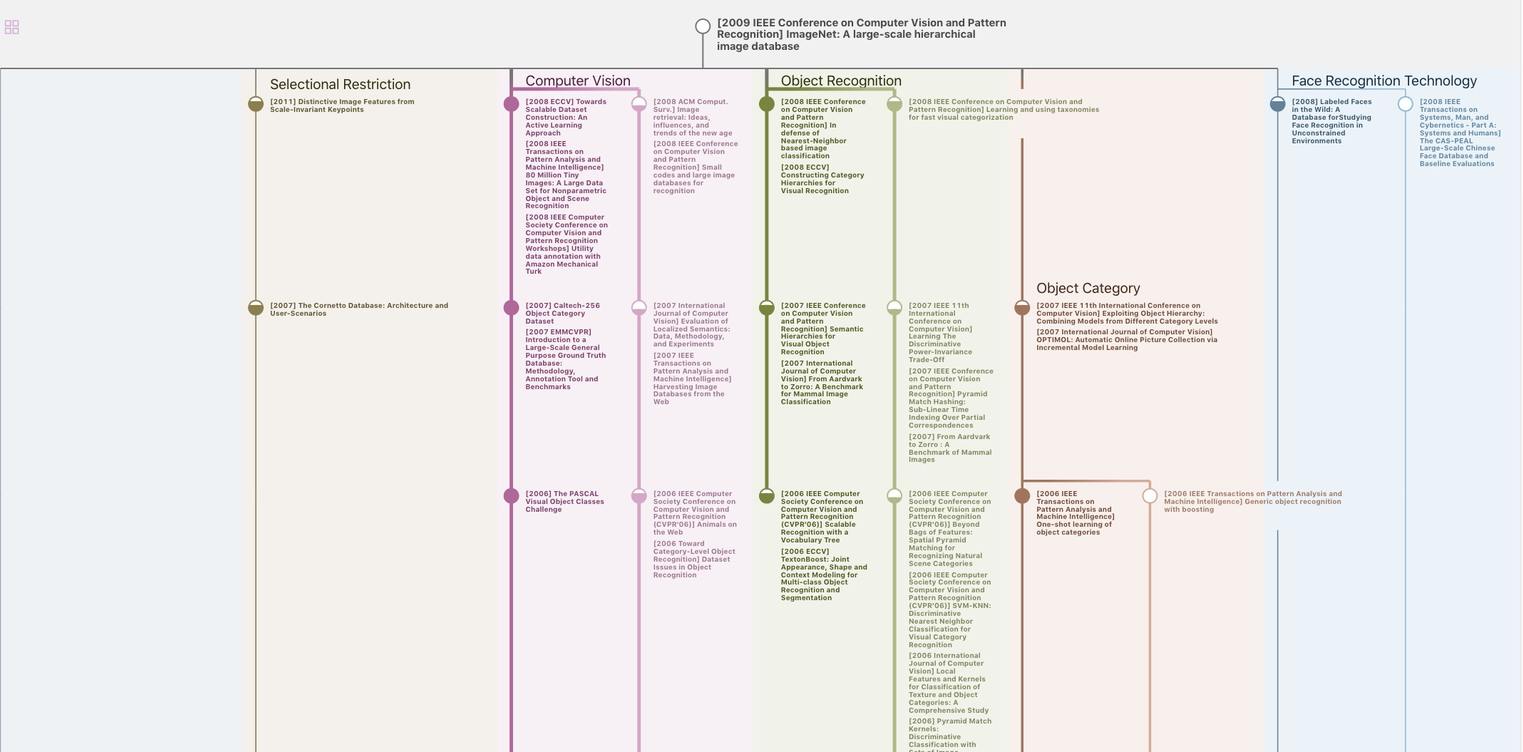
生成溯源树,研究论文发展脉络
Chat Paper
正在生成论文摘要