On sampling diluted Spin Glasses using Glauber dynamics
arxiv(2024)
摘要
Spin-glasses are Gibbs distributions that have been studied in CS for many
decades. Recently, they have gained renewed attention as they emerge naturally
in learning, inference, optimisation etc.
We consider the Edwards-Anderson (EA) spin-glass distribution at inverse
temperature β when the underlying graph is an instance of G(n,d/n).
This is the random graph on n vertices where each edge appears independently
with probability d/n and d=Θ(1). We study the problem of approximate
sampling from this distribution using Glauber dynamics.
For a range of β that depends on d and for typical instances of the
EA model on G(n,d/n), we show that the corresponding Glauber dynamics
exhibits mixing time O(n^2+3/log^2 d).
The range of β for which we obtain our rapid-mixing results correspond
to the expected influence being <1/d; we conjecture that this is the best
possible.
Unlike the mean-field spin-glasses, where the problem has been studied
before, the diluted case has not.
We utilise the well-known path-coupling technique. In the standard Glauber
dynamics on G(n,d/n), one has to deal with the so-called effect of high
degree vertices. Here, rather than considering degrees, it is more natural to
use a different measure on the vertices called aggregate influence.
We build on the block-construction approach proposed by [Dyer et al. 2006] to
circumvent the problem of high-degree vertices. Specifically, we first
establish rapid mixing for an appropriately defined block-dynamics. We design
this dynamics such that vertices of large aggregate influence are placed deep
inside their blocks. Then, we obtain rapid mixing for the Glauber dynamics
utilising a comparison argument.
更多查看译文
AI 理解论文
溯源树
样例
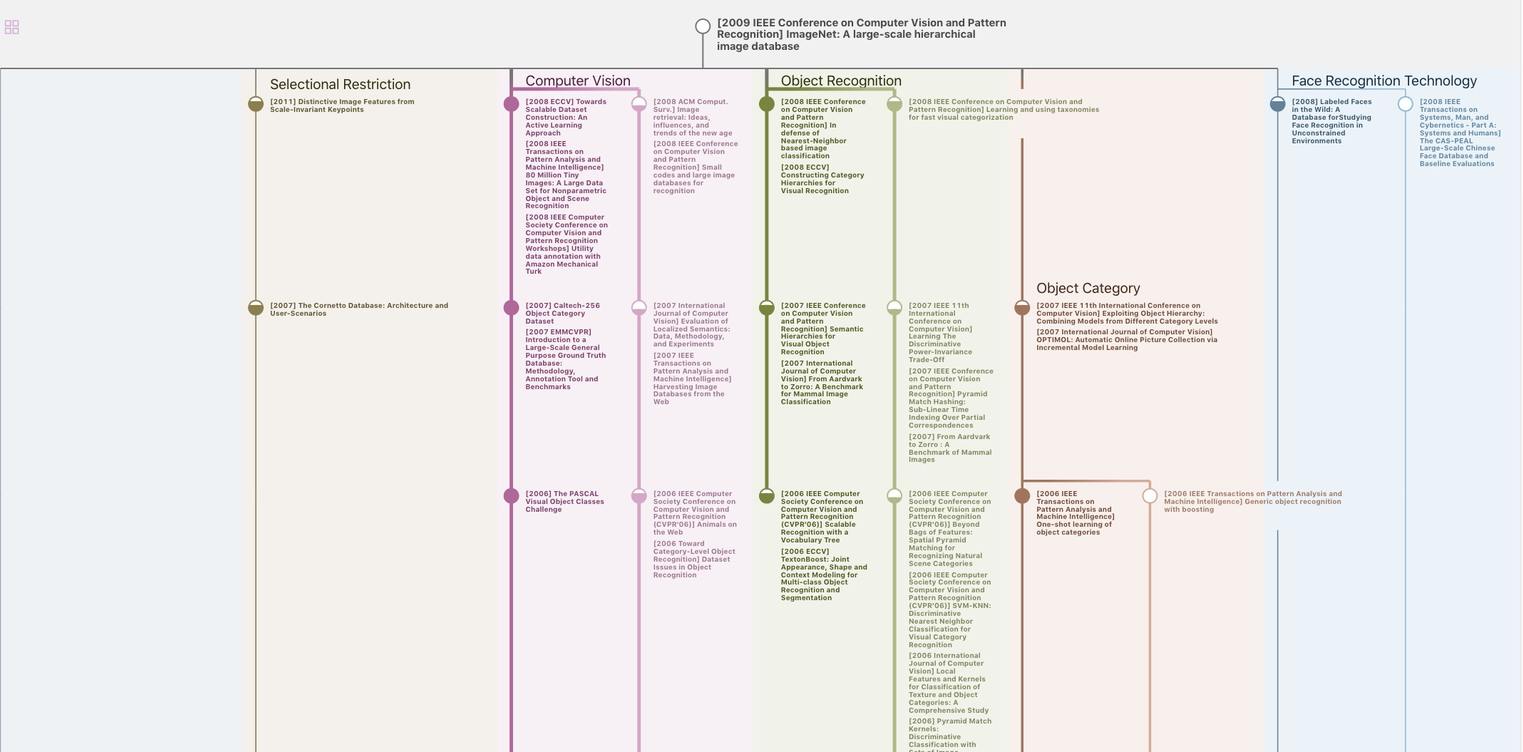
生成溯源树,研究论文发展脉络
Chat Paper
正在生成论文摘要