A simple reconstruction method to infer nonreciprocal interactions and local driving in complex systems
arxiv(2024)
摘要
Data-based inference of directed interactions in complex dynamical systems is
a problem common to many disciplines of science. In this work, we study
networks of spatially separate dynamical entities, which could represent
physical systems that interact with each other by reciprocal or nonreciprocal,
instantaneous or time-delayed interactions. We present a simple approach that
combines Markov state models with directed information-theoretical measures for
causal inference that can accurately infer the underlying interactions from
noisy time series of the dynamical system states alone. Remarkably, this is
possible despite the built-in simplification of a Markov assumption and the
choice of a very coarse discretization at the level of probability estimation.
Our test systems are an Ising chain with nonreciprocal coupling imposed by
local driving of a single spin, and a system of delay-coupled linear stochastic
processes. Stepping away from physical systems, the approach infers
cause-effect relationships, or more generally, the direction of mutual or
one-way influence. The presented method is agnostic to the number of
interacting entities and details of the dynamics, so that it is widely
applicable to problems in various fields.
更多查看译文
AI 理解论文
溯源树
样例
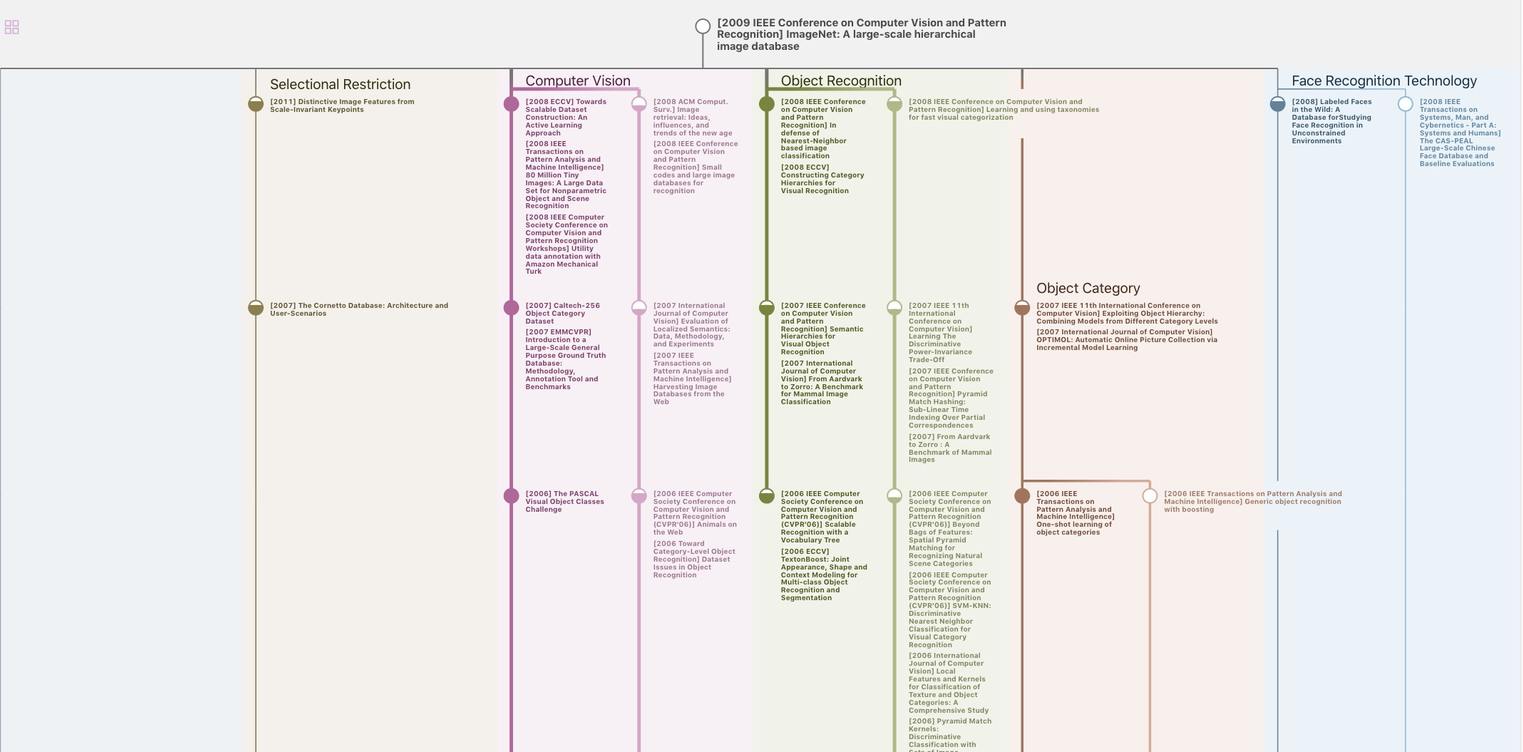
生成溯源树,研究论文发展脉络
Chat Paper
正在生成论文摘要