Photovoltaic Inverter Failure Mechanism Estimation Using Unsupervised Machine Learning and Reliability Assessment
IEEE TRANSACTIONS ON RELIABILITY(2024)
摘要
This article introduces a data-driven approach to assessing failure mechanisms and reliability degradation in outdoor photovoltaic (PV) string inverters. The manufacturer's stated PV inverter lifetime can vary due to the impact of operating site conditions. To address limitations in degradation estimation through accelerated testing, condition monitoring, or degradation modeling, we propose a machine learning (ML) oriented approach. Utilizing data from a 1.4 MW PV power plant operational since 2016, with 46 string PV inverters tied to the grid, we employ the unsupervised one-class support vector machine ML technique to analyze inverter and sensor data, capable of classifying humidity cycling and temperature fluctuations as dominant failure mechanisms. Utilizing the anomaly alert relationship and alert details specific to the inverter, the level of PV inverter output is considered as its availability or available reliability. Subsequently, a continuous Markov model is applied to six-month alert data, revealing an average stated reliability of 20% after 20 years of continuous operation. These results support recommendations for time-bound preventive measures to enhance PV inverter reliability under diverse outdoor conditions. The approach provides a nondestructive, top-down, and generalized method for analyzing any commercial PV inverter exposed to outdoor conditions, contingent on the availability of relevant data.
更多查看译文
关键词
Classification and Regression Tree (CART),correlation,degradation,inverter,micro grid,One Class Support Vector Machine (OCSVM),reliability
AI 理解论文
溯源树
样例
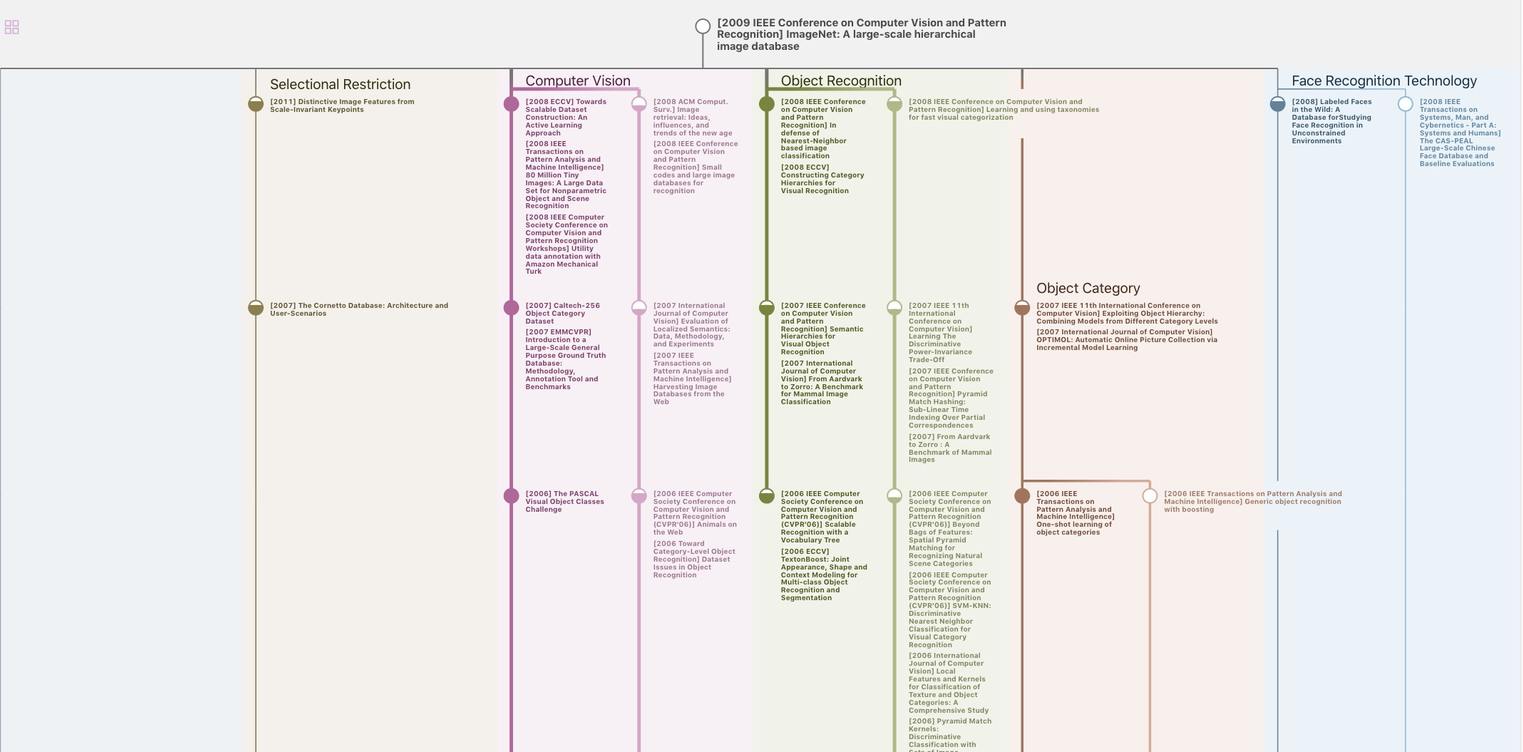
生成溯源树,研究论文发展脉络
Chat Paper
正在生成论文摘要