Robust Discriminative t-Linear Subspace Learning for Image Feature Extraction
IEEE Transactions on Circuits and Systems for Video Technology(2024)
摘要
Subspace learning has been widely applied for joint feature extraction and dimensionality reduction, demonstrating significant efficacy. Numerous subspace learning methods with diverse assumptions regarding the criteria for the target subspaces have been developed to obtain compact and interpretable data representations. However, when applied to image data, existing methods fail to fully exploit the inherent correlations within the image set. This paper proposes a Robust Discriminative t-Linear Subspace Learning model (RDtSL) to tackle this issue using t-product. The model mainly has four strengths: 1) Taking advantage of t-product, RDtSL learns the projection basis directly from the image set while fully exploiting its internal correlations; 2) Based on its energy preservation module, RDtSL retains the primary energy of samples in the learned subspace, maintaining satisfactory performance even with low subspace dimensions; 3) Class-distinctive features are effectively preserved in the learned representations due to the incorporation of the classification module; 4) Relying on its graph embedding module, RDtSL learns an affinity graph of samples adaptively to enrich the data representations with locality and similarity information. The harmonious balance maintained between the three proposed modules helps RDtSL learn discriminative and informative data representations. We also develop an iterative algorithm to solve RDtSL. Extensive experiments on benchmark databases demonstrate the superiority of the proposed model.
更多查看译文
关键词
Dimensionality reduction,image feature extraction,subspace learning,t-product
AI 理解论文
溯源树
样例
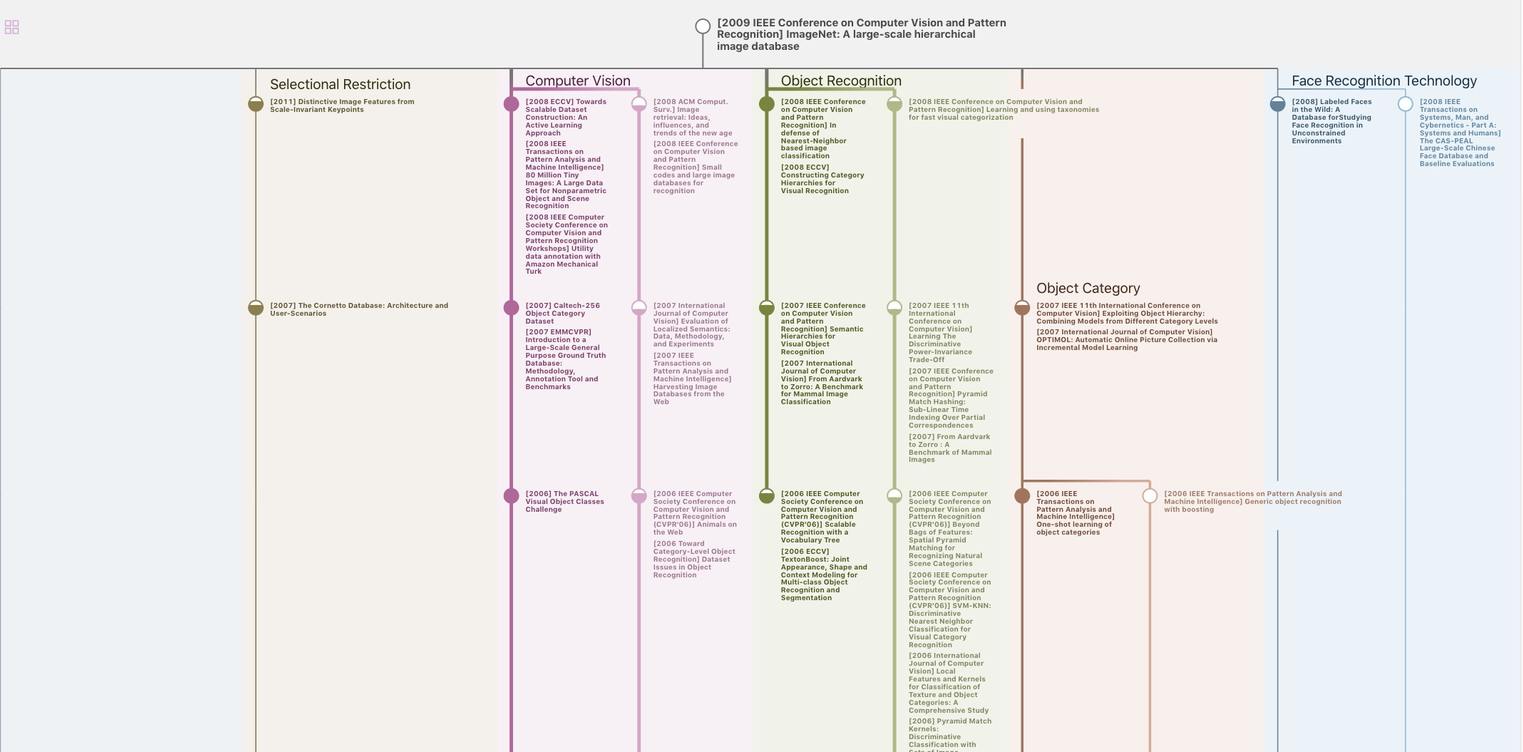
生成溯源树,研究论文发展脉络
Chat Paper
正在生成论文摘要