Generalizing Tree-Level Sap Flow Across the European Continent
GEOPHYSICAL RESEARCH LETTERS(2024)
摘要
Sap flow offers key insights about transpiration dynamics and forest-climate interactions. Accurately simulating sap flow remains challenging due to measurement uncertainties and interactions between global and local environmental controls. Addressing these complexities, this study leveraged Long Short-Term Memory networks (LSTMs) with SAPFLUXNET to predict hourly tree-level sap flow across Europe. We built models with diverse training sets to assess performance under previously unseen conditions. The average Kling-Gupta Efficiency was 0.77 for models trained on 50% of time series across all forest stands, and 0.52 for models trained on 50% of the forest stands. Continental models not only matched but surpassed the performance of specialized and baselines for all genera and forest types, showcasing the capacity of LSTMs to effectively generalize across tree genera, climates, and forest ecosystems given minimal inputs. This study underscores the potential of LSTMs in generalizing state-dependent ecohydrological processes and bridging tree level measurements to continental scales. Transpiration is the dominant flux of water from the land surface to the atmosphere, yet it remains challenging to measure and estimate especially given different climates and tree types. This study shows how large-scale deep learning models can simulate sap flow, the movement of water within trees, with high precision, even in forests and conditions not previously studied. We show that these models excel when they learn from large and diverse data sets. The flexible design of our model training means that every new sap flow measurement can potentially be used to further optimize our networks. Our findings indicate that this approach of continuously updating the model with new information greatly improves its performance to simulate and predict tree-level sap flow. This work thus highlights the potential of deep learning models to generalize sap flow, thereby enhancing ecohydrological investigations from the tree to the continental scale. Long Short-Term Memory networks (LSTMs) can predict hourly sap flow for diverse trees and climates across Europe bridging tree level measurements to continental scales Training on the extensive SAPFLUXNET data set enhances the ability of the LSTMs to simulate sap flow accurately in time and space The study underscores the value of large-scale deep learning models and advocates for the expansion of data sets like SAPFLUXNET
更多查看译文
关键词
sap flow,LSTMs,deep learning,ecohydrology,transpiration,vegetation dynamics
AI 理解论文
溯源树
样例
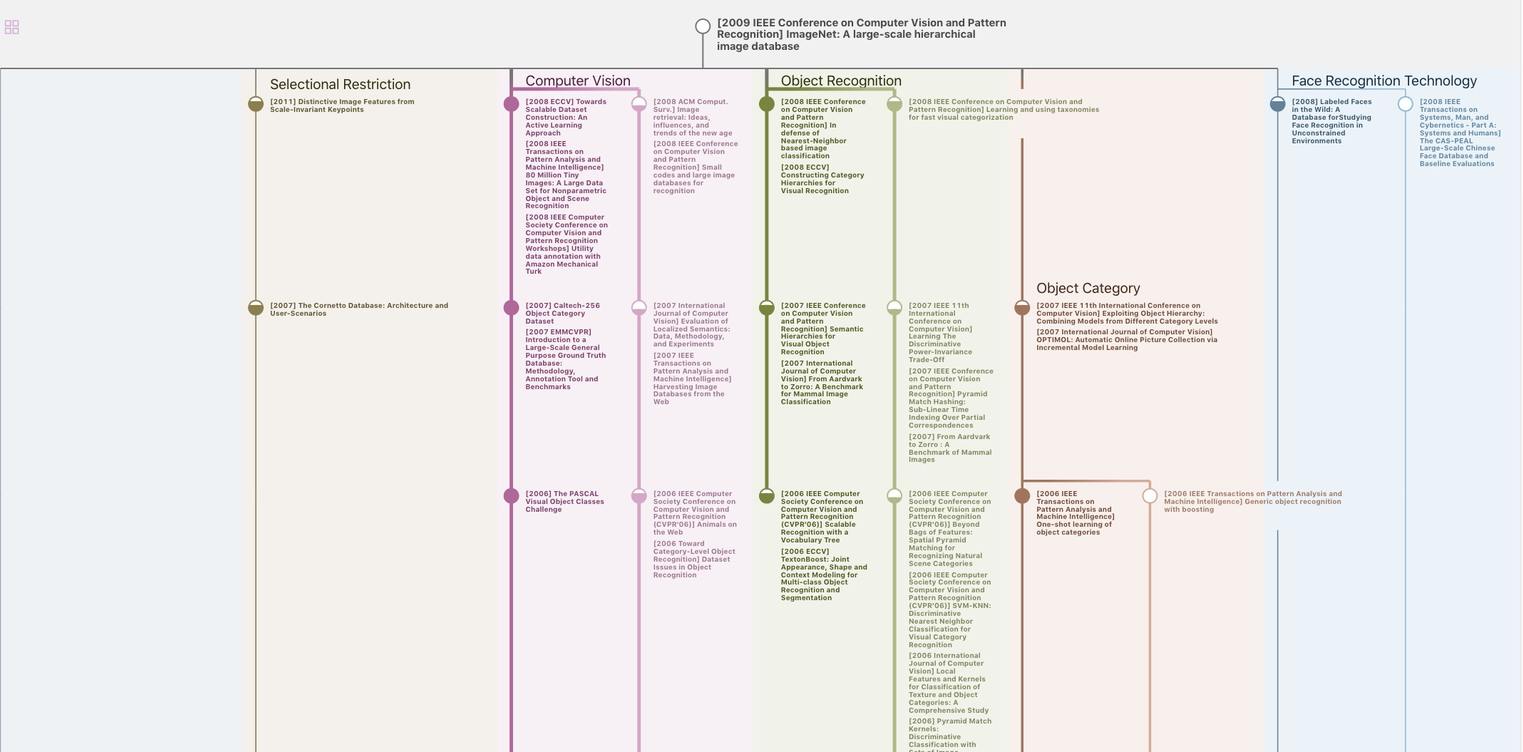
生成溯源树,研究论文发展脉络
Chat Paper
正在生成论文摘要