Boosting Robot Intelligence in Practice: Enhancing Robot Task Planning with Large Language Models
2023 8th International Conference on Robotics and Automation Engineering (ICRAE)(2023)
摘要
Task planning capabilities are crucial for intelligent robots to operate autonomously in the physical world. However, traditional Planning Domain Definition Language (PDDL) based methods often suffer from combinatorial explosion and unsat-isfactory planning time. In this paper, we propose enhancing robot task planning with large language models (LLMs) in an innovative way - using LLMs to guide the search process of PDDL planners rather than replacing PDDL planning completely. The LLMs guide the search process of PDDL planners with learned heuristics and provide constraint reasoning to reduce the search space. To address potential pitfalls of LLMs, a verification mechanism is added at the execution stage to validate plan correctness. We evaluated our method on a real scenario, end-of-life vehicle battery disassembly. Experimental results demonstrate that incorporating LLMs into the planning pipeline can significantly improve planning efficiency and scalability while maintaining plan validity. This research provides a promising direction towards integrating language models with classical approaches to boost robot intelligence for practical applications. The proposed framework makes a solid step forward in en-hancing the task planning capability of future intelligent robotic systems.
更多查看译文
关键词
robot,task planning,LLM
AI 理解论文
溯源树
样例
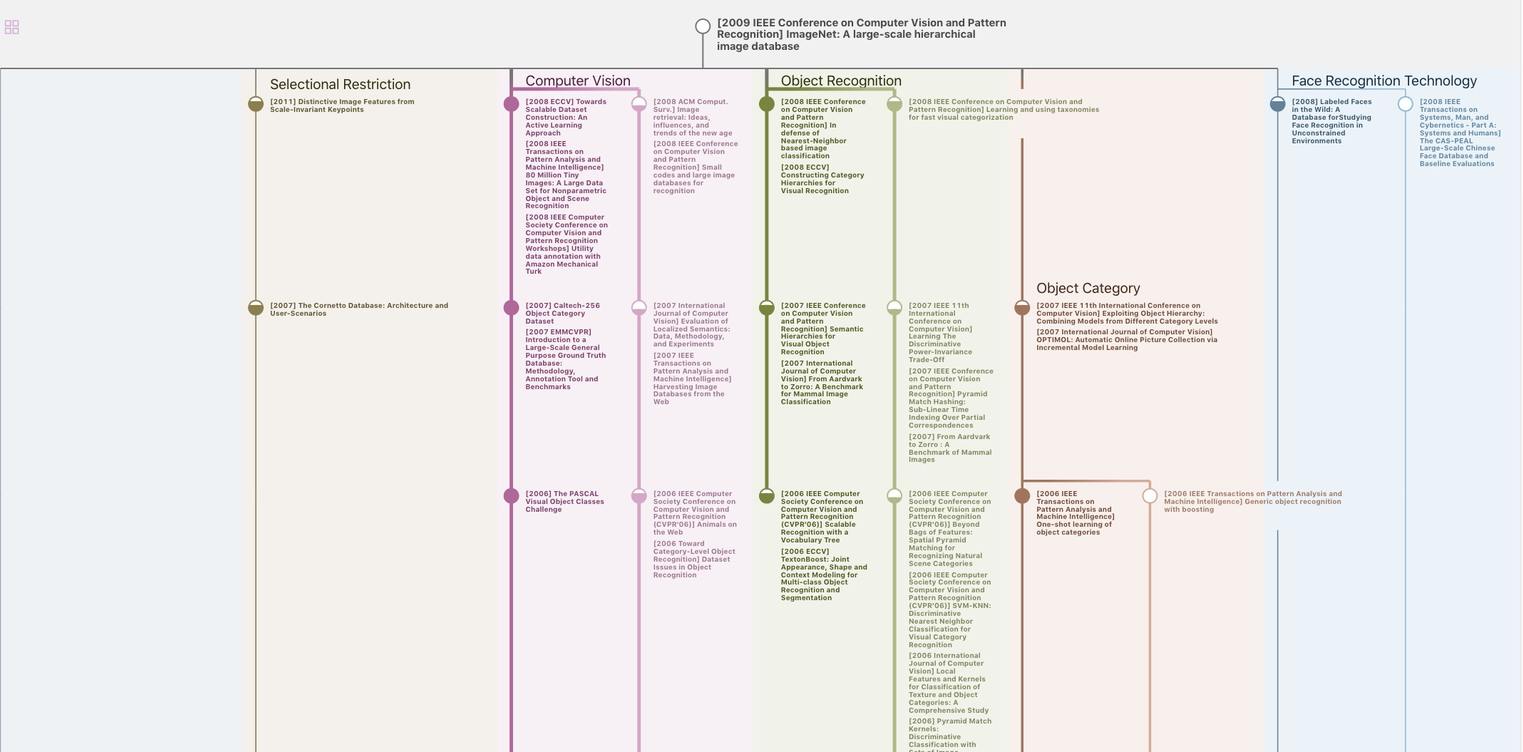
生成溯源树,研究论文发展脉络
Chat Paper
正在生成论文摘要