Next-generation non-linear and collapse prediction models for short- to long-period systems via machine learning methods
Engineering Structures(2024)
摘要
Since the 1960 s, a cornerstone of earthquake engineering has been estimating the non-linear response of structures based just on lateral strength, modal properties, and the anticipated seismic demand. Over the years, several studies have quantified this empirical relationship and integrated it within seismic design and assessment methodologies. These have been widely accepted for practical application and adopted in building codes worldwide. While these models work reasonably well, there are still areas in which improvements can be made, especially concerning their robust quantification of uncertainty. This is mainly due to the amount of data used to quantify these empirical relationships, the choice of functional forms during the fitting, the model fitting and testing process, and how the ground motion shaking intensity is characterised. This study tackles these issues via the non-linear analysis of single-degree-of-freedom oscillators to train several machine learning (ML) models. This was to examine the accuracy and applicability of such models within a seismic engineering context and explore potential gains in quantifying the non-linear response of structures via next-generation intensity measures, namely average spectral acceleration, Saavg. The results show that the Decision Tree and XGBoost models worked well across a broad range of periods, accurately predicting collapse and non-collapse responses. Appraising these with existing models showed a notable improvement all around. It indicates that the models based on data-driven ML approaches represent a positive step and can be seamlessly integrated with seismic analysis methodologies utilised worldwide. Subsequently, a Python-based library of these XGBoost ML models was developed and made available online to foster practical application.
更多查看译文
关键词
Machine learning,SDOF models,Average spectral acceleration,Non-linear dynamic analysis
AI 理解论文
溯源树
样例
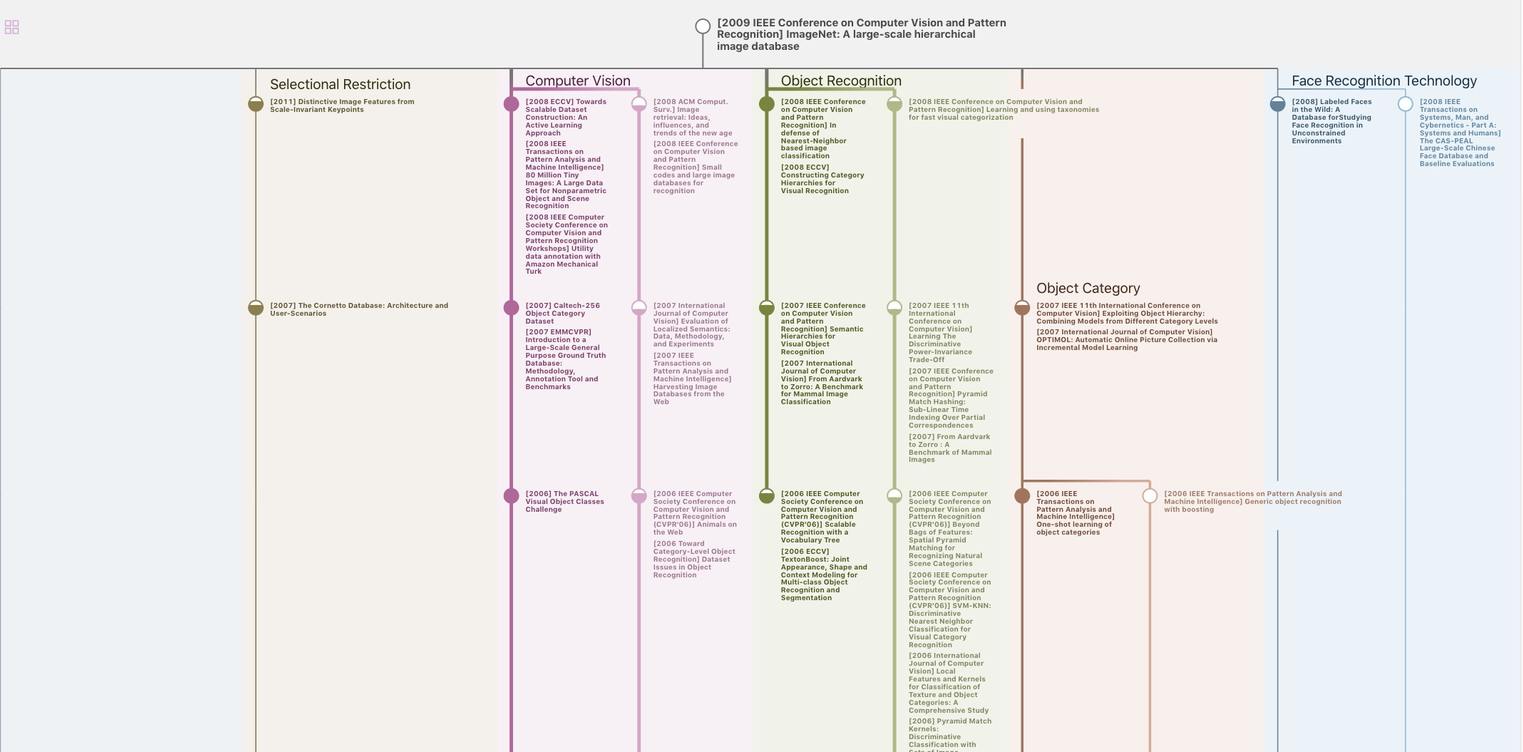
生成溯源树,研究论文发展脉络
Chat Paper
正在生成论文摘要