Thermal characterization of the spindle-bearing system under different working conditions based on a hybrid-driven framework combining data-driven and model-based methods
Journal of Manufacturing Processes(2024)
摘要
Due to the complex physical mechanism and the difficulty in deploying a large number of temperature sensors, it is challenging for the spindle-bearing system to accurately analysis thermal characteristics. In this study, a hybrid-driven framework combining data-driven and model-based methods is proposed to estimate uncertainty thermal parameters and predict thermal errors. Firstly, the temperature prediction model is built based on the thermal network method. Then SIAN and Sobol’s global sensitivity indicator are used to extracted parameters with global structural identifiability and estimability respectively as uncertainty parameters to construct the state space equation of the model. In addition, the multilayer particle filter method (MLPF) based on the particle filter and the sparse identification is proposed to improve the accuracy and robustness of the thermal parameter estimation. Finally, thermal characteristics experiments of the spindle-bearing system are conducted in different working conditions with only a few temperature sensors to verify the performance of the hybrid-drive framework. The result shows that the proposed framework enables effective, accurate and robust prediction of the temperature field at different working conditions.
更多查看译文
关键词
Hybrid-driven framework,Structural identifiability,Estimability,Multilayer particle filter,Thermal characterization,Spindle-bearing system
AI 理解论文
溯源树
样例
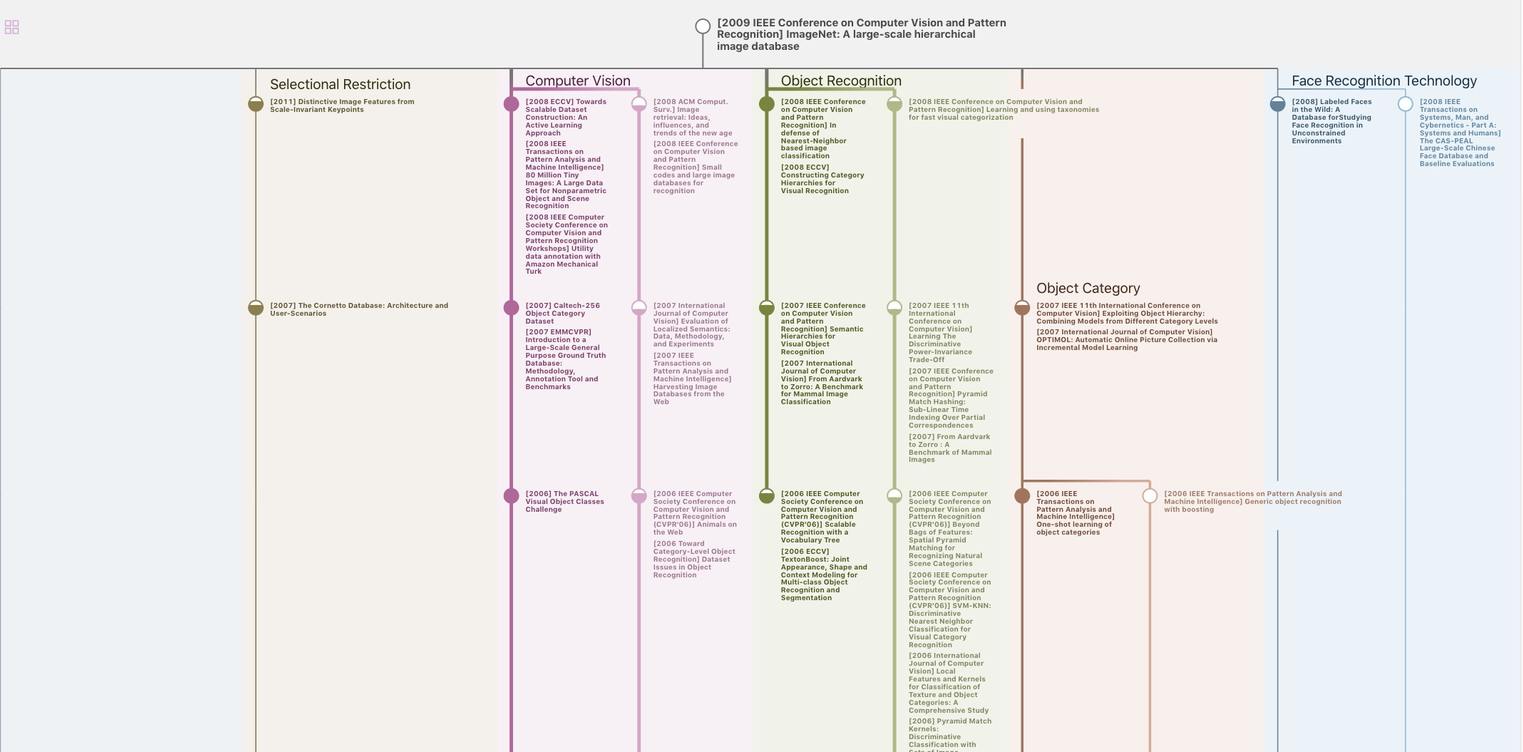
生成溯源树,研究论文发展脉络
Chat Paper
正在生成论文摘要