Method for Incomplete and Imbalanced Data Based on Multivariate Imputation by Chained Equations and Ensemble Learning.
IEEE JOURNAL OF BIOMEDICAL AND HEALTH INFORMATICS(2024)
摘要
The classification analysis of incomplete and imbalanced data is still a challenging task since these issues could negatively impact the training of classifiers, which were also found in our study on the physical fitness assessments of patients. And in fields such as healthcare, there are higher requirements for the accuracy of the generated imputation values. To train a high-performance classifier and pursue high accuracy, we attempted to resolve any potential negative impact by using a novel algorithmic approach based on the combination of multivariate imputation by chained equations and the ensemble learning method (MICEEN), which can solve the two problems simultaneously. We used multivariate imputation by chained equations to generate more accurate imputation values for the training set passed to ensemble learning to build a predictor. On the other hand, missing values were introduced into minority classes and used them to generate new samples belonging to the minority classes in order to balance the distribution of classes. On real-world datasets, we perform extensive experiments to assess our method and compare it to other state-of-the-art approaches. The advantages of the proposed method are demonstrated by experimental results for the benchmark datasets and self-collected datasets of physical fitness assessment of tumor patients with varying missing rates.
更多查看译文
关键词
Training,Mathematical models,Ensemble learning,Task analysis,Costs,Support vector machines,Data models,Data incompleteness,class imbalance,physical fitness assessment,malignant tumor patients,multivariate imputation by chained equations,ensemble learning
AI 理解论文
溯源树
样例
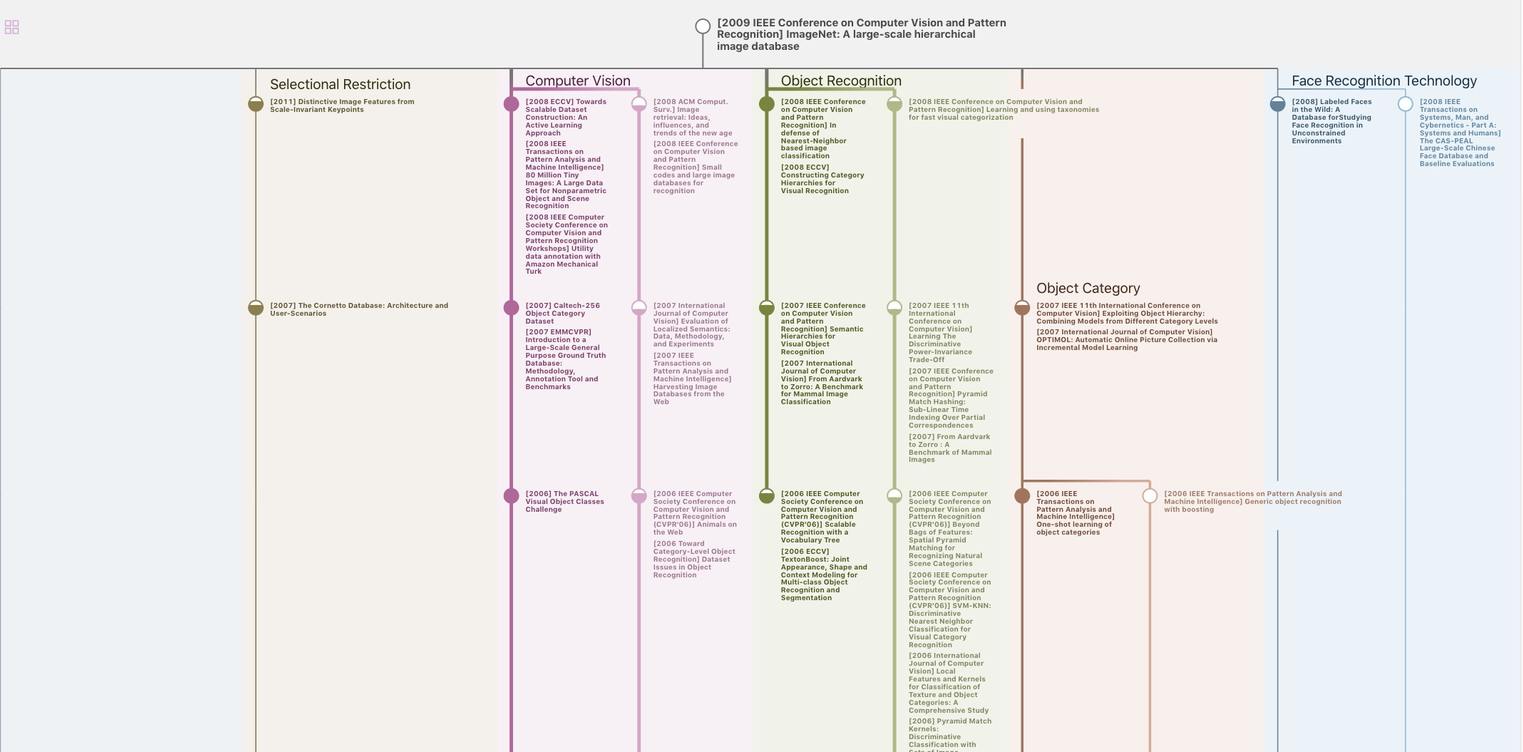
生成溯源树,研究论文发展脉络
Chat Paper
正在生成论文摘要