A study of machine learning object detection performance for phased array ultrasonic testing of carbon fibre reinforced plastics
NDT & E International(2024)
摘要
The growing adoption of Carbon Fibre Reinforced Plastics (CFRPs) in the aerospace industry has resulted in a significant reliance on Non-Destructive Evaluation (NDE) to ensure the quality and integrity of these materials. The interpretation of large amounts of data acquired from automated robotic ultrasonic scanning by expert operators is often time consuming, tedious, and prone to human error creating a bottleneck in the manufacturing process. However, with ever growing trend of computing power and digitally stored NDE data, intelligent Machine Learning (ML) algorithms have been gaining more traction than before for NDE data analysis. In this study, the performance of ML object detection models, statistical methods for defect detection, and traditional amplitude thresholding approaches for defect detection in CFRPs were compared. A novel augmentation technique was used to enhance synthetically generated datasets used for ML model training. All approaches were tested on real data obtained from an experimental setup mimicking industrial conditions, with ML models showing improvement over amplitude thresholding and statistical thresholding techniques. The advantages and limitations of all methods are reported and discussed.
更多查看译文
关键词
Automated defect detection,Machine learning,Deep learning in ultrasonic testing,Non-destructive evaluation,Carbon fibre reinforced polymers,Aerospace composites
AI 理解论文
溯源树
样例
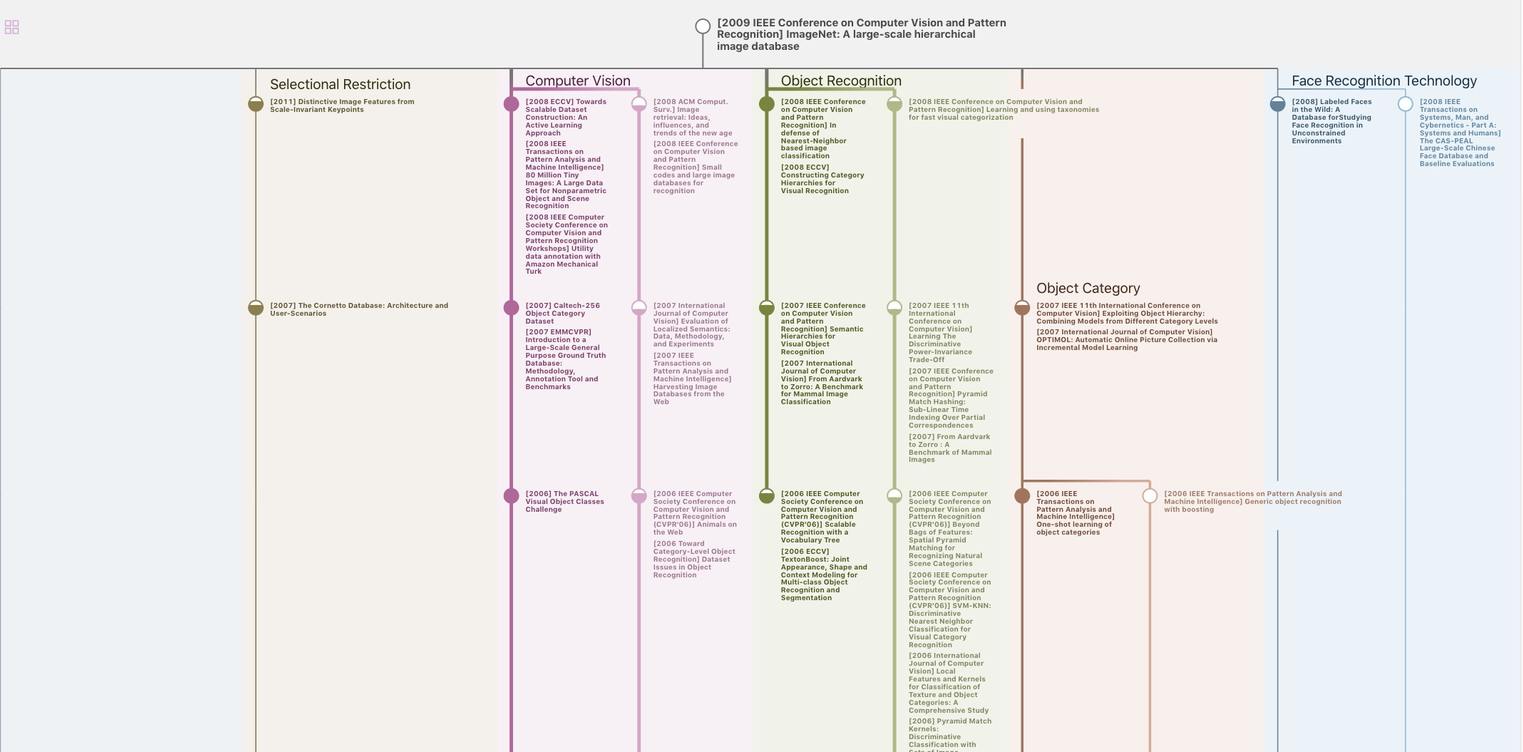
生成溯源树,研究论文发展脉络
Chat Paper
正在生成论文摘要