234 Predictive Radiomics in Glioblastoma: A Machine Learning Approach for Personalized Survival Outcomes and a Novel Web Application
Neurosurgery(2024)
摘要
INTRODUCTION: Diverse characteristics of glioblastomas (GBMs) complicate survival predictions, requiring personalized approaches. METHODS: From The Cancer Imaging Archive (TCIA) database, 611 patients from the UPENN-GBM collection and 500 patients from the UCSF-PDGM collections were included in this study. UPENN-GBM collection was used as the training set and for 5-fold internal cross-validation, whereas the UCSF-PDGM collection was used for external validation. Survival at 3, 6, 12, and 24 months were the outcomes of interest. Necrotic tumor core, enhancing tumor, and whole tumor segmentation masks for T1 with contrast, T1, T2, and FLAIR images were obtained automatically with a pre-trained Swin UNETR model. From these regions of interest, radiomic features were extracted using the PyRadiomicsLibrary. The LASSO (least absolute shrinkage and selection operator) method was employed for radiomic feature selection. The ML algorithms TabPFN, XGBoost, LightGBM, Support Vector Machine (SVM), and Random Forest (RF) were utilized to predict the survival outcomes of interest. RESULTS: The highlight of our study, our web application is available for use via the following link: https://huggingface.co/spaces/MSHSNeurosurgeryResearch/Radiomics_Based_Glioblastoma_Survival_Prediction_Tool. CONCLUSIONS: This study not only provides a radiomics pipeline for predicting the GBM survival outcomes, but it also contributes to the clinical adoption of these models by developing a fully automatic and user-friendly web application which make them available to clinicians worldwide.
更多查看译文
AI 理解论文
溯源树
样例
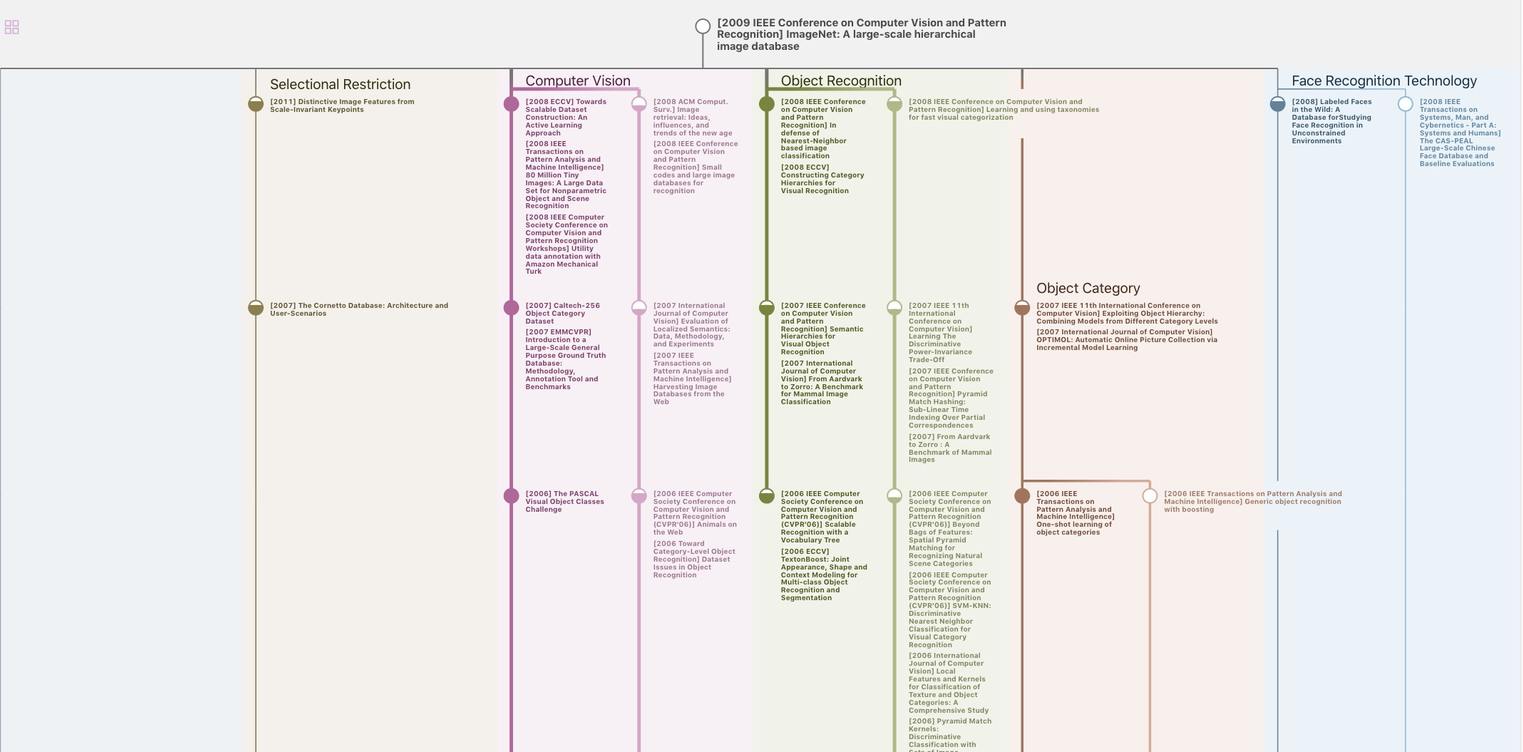
生成溯源树,研究论文发展脉络
Chat Paper
正在生成论文摘要