Integrating Real-Time Location Systems with Electronic Medical Records: A Machine Learning Approach for In-Hospital Fall Risk Prediction
medrxiv(2024)
摘要
Hospital falls are the most prevalent adverse event in healthcare, posing significant risks to patient health outcomes and institutional care quality. The effectiveness of several fall prediction models currently in use is limited by various clinical factors. This study explored the efficacy of merging real-time location system (RTLS) data with clinical information to enhance the accuracy of in-hospital fall predictions. The model performances were compared based on the clinical data, RTLS data, and a hybrid approach using various evaluation metrics. The RTLS and integrated clinical data were obtained from 22,201 patients between March 2020 and June 2022. From the initial cohort, 118 patients with falls and 443 patients without falls were included. Predictive models were developed using the XGBoost algorithm across three distinct frameworks: clinical model, RTLS model, and clinical + RTLS model. The model performance was evaluated using metrics, such as AUROC, AUPRC, accuracy, PPV, sensitivity, specificity, and F1 score. Shapley additive explanation values were used to enhance the model interpretability. The clinical model yielded an AUROC of 0.813 and AUPRC of 0.407. The RTLS model demonstrated superior fall prediction capabilities, with an AUROC of 0.842 and AUPRC of 0.480. The clinical + RTLS model excelled further, achieving an AUROC of 0.853 and AUPRC of 0.497. Feature importance analysis revealed that movement patterns of patients on the last day of their stay were significantly associated with falls, together with elevated RDW levels, sedative administration, age. This study underscored the advantages of combining RTLS data with clinical information to predict in-hospital falls more accurately. This innovative technology-driven approach may enhance early fall risk detection during hospitalization, potentially preventing falls, improving patient safety, and contributing to more efficient healthcare delivery.
### Competing Interest Statement
The authors have declared no competing interest.
### Funding Statement
This research was supported by a grant of the Korea Health Technology R&D Project through the Korea Health Industry Development Institute (KHIDI), funded by the Ministry of Health and Welfare, Republic of Korea (grant number: HI22C0452). And this work was supported by the National Research Foundation of Korea (NRF) grant funded by the Korean government (MSIT) (RS-2023-00253897) and grand funded by the Ministry of Education (2021R1I1A1A01060333).
### Author Declarations
I confirm all relevant ethical guidelines have been followed, and any necessary IRB and/or ethics committee approvals have been obtained.
Yes
The details of the IRB/oversight body that provided approval or exemption for the research described are given below:
This study was approved by the Institutional Review Board of Yonsei University Severance Hospital (No. 9-2021-0037).
I confirm that all necessary patient/participant consent has been obtained and the appropriate institutional forms have been archived, and that any patient/participant/sample identifiers included were not known to anyone (e.g., hospital staff, patients or participants themselves) outside the research group so cannot be used to identify individuals.
Yes
I understand that all clinical trials and any other prospective interventional studies must be registered with an ICMJE-approved registry, such as ClinicalTrials.gov. I confirm that any such study reported in the manuscript has been registered and the trial registration ID is provided (note: if posting a prospective study registered retrospectively, please provide a statement in the trial ID field explaining why the study was not registered in advance).
Yes
I have followed all appropriate research reporting guidelines, such as any relevant EQUATOR Network research reporting checklist(s) and other pertinent material, if applicable.
Yes
The datasets used and/or analyzed in the current study are available from the corresponding author upon reasonable request.
更多查看译文
AI 理解论文
溯源树
样例
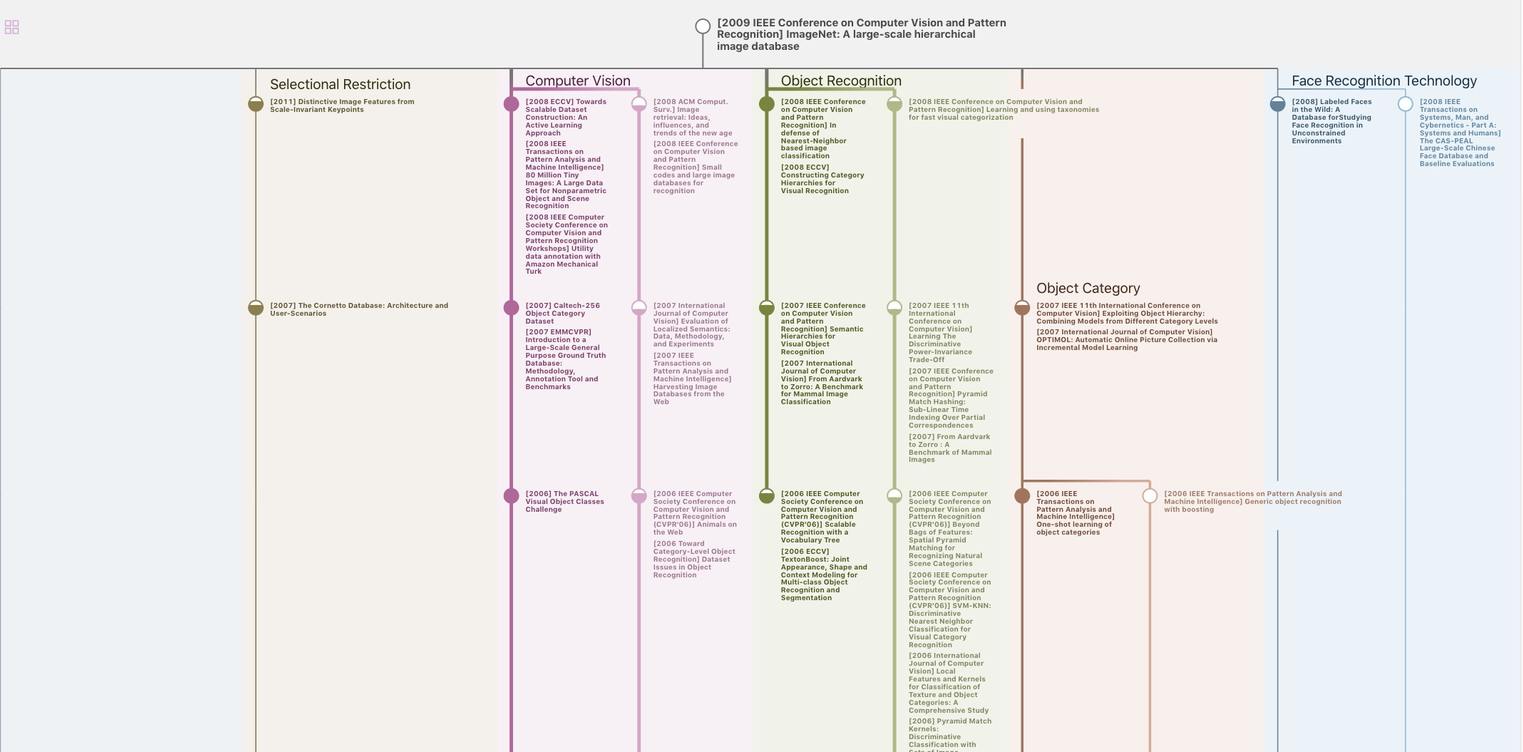
生成溯源树,研究论文发展脉络
Chat Paper
正在生成论文摘要