Information-guided signal multi-granularity contrastive feature learning for fault diagnosis with few labeled data
Advanced Engineering Informatics(2024)
摘要
Fault diagnosis is important for isolating the root causes and supporting preventive maintenance of an equipment. However, the lack of labeled fault samples often limits the performance of fault diagnosis in practical situations. In this paper, we propose an information-guided signal multi-granularity contrastive feature learning (IMCFL) method with few labeled data to identify fault types. Unlike manual selection of data augmentation in traditional contrastive learning, IMCFL adaptively generates task-relevant augmented views for contrastive learning by differentiable automatic augmentation guided by information from few labeled (no more than 5%) data. Furthermore, a multi-granularity contrastive loss is constructed for the feature extractor to generate discriminative feature representation considering the characteristics of the signals. By exploring instance-level relationships and temporal relationships within the signal in multiple successive down-sampling operations, features of different granularities can be adequately extracted. After completing the contrastive learning, fault diagnosis is accomplished by a simple classifier without fine-tuning. Experiments on bearing and gear datasets demonstrate the diagnostic accuracy of the proposed method compared to other methods. Further transfer learning experiments show that the proposed method has the ability to be applied to different devices for fault diagnosis through knowledge transfer of its pre-trained powerful feature extractors.
更多查看译文
关键词
Contrastive learning,Mechanical equipment,Few labeled data,Intelligent fault diagnosis
AI 理解论文
溯源树
样例
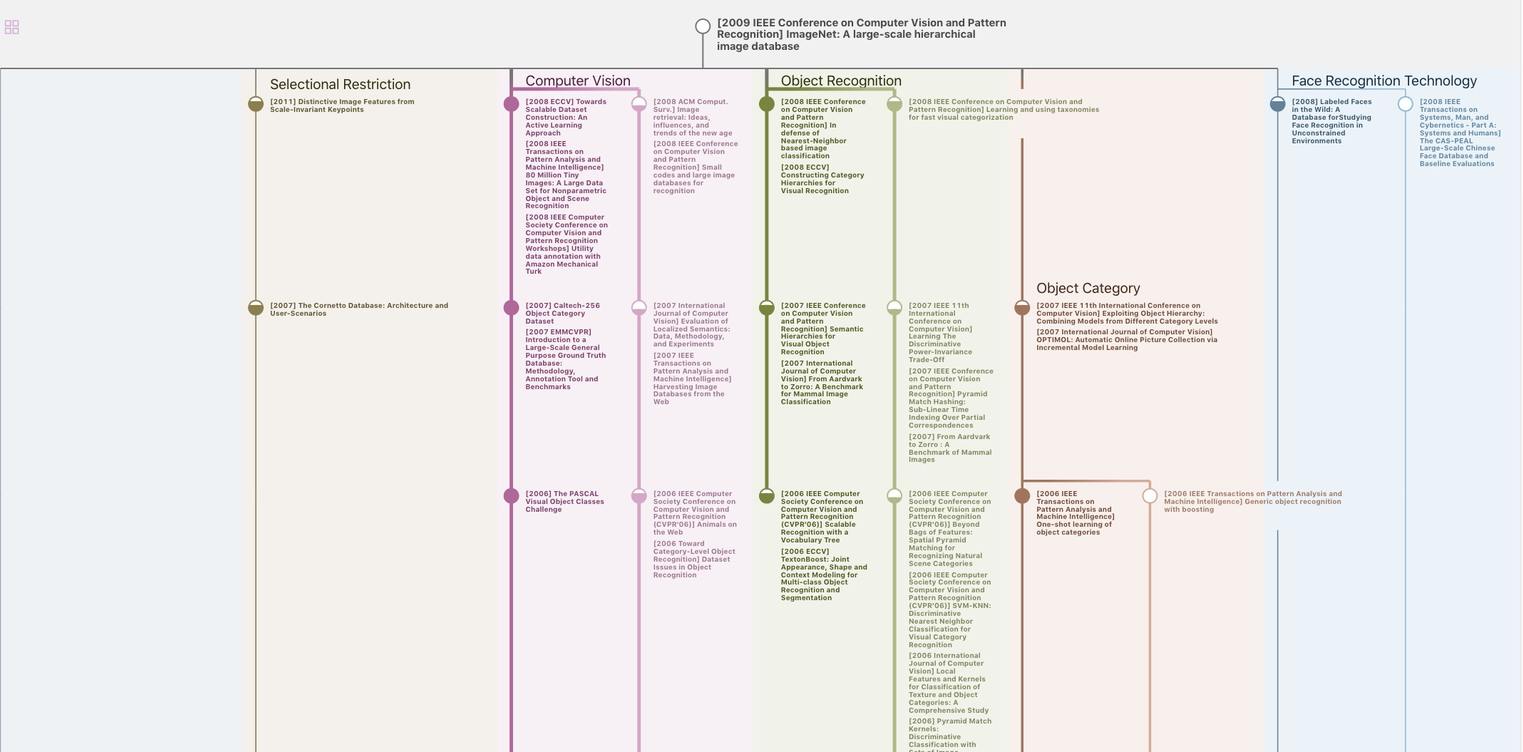
生成溯源树,研究论文发展脉络
Chat Paper
正在生成论文摘要