Tracking User Sentiment Changes on Social Networks
PROCEEDINGS OF THE 2023 IEEE/ACM INTERNATIONAL CONFERENCE ON ADVANCES IN SOCIAL NETWORKS ANALYSIS AND MINING, ASONAM 2023(2023)
摘要
We present a time-dependent approach for learning temporal co-variates explaining the subsequent user sentiments in social networks. In most of the existing approaches, we note that the underlying text classification setting, generally used to model user sentiments, is designed to ingest a user comment generated at time t to predict his corresponding sentiment at the same time. Under such constraint, user sentiments can only be given whenever she or he has generated a comment. Furthermore, the evolving historical sentiments are omitted and no anticipation of subsequent sentiments could be made. To alleviate this limitation, we propose a time-dependent approach that takes advantage of historical user comments to learn temporal co-variates that explain their evolving sentiments. We demonstrate that our approach could be used to predict user sentiments at subsequent times ahead. Experimental results on Tweets data, during the Covid-19 pandemic, illustrate the suitability of our approach.
更多查看译文
关键词
Graph representation learning,Time series,Prediction
AI 理解论文
溯源树
样例
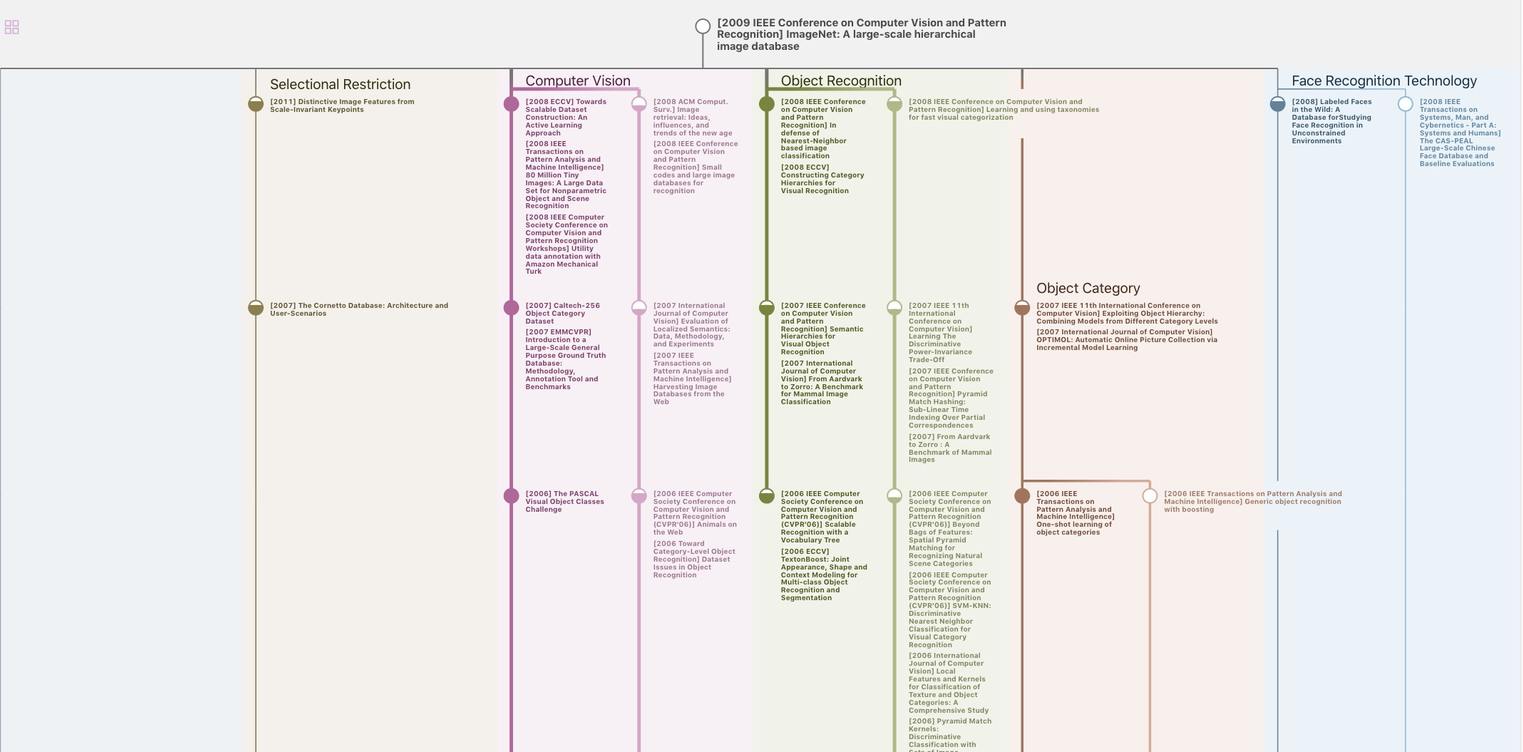
生成溯源树,研究论文发展脉络
Chat Paper
正在生成论文摘要