A Multi-Task Learning Framework Using Graph Attention Network for User Stance and Rumor Veracity Prediction
ASONAM '23 Proceedings of the International Conference on Advances in Social Networks Analysis and Mining(2024)
摘要
In this paper, we present a multi-task learning framework consisting of two interrelated components for the joint modeling of stance classification and rumor veracity prediction on Twitter. The proposed hierarchical framework models a conversation sequence using a graph attention network, leveraging a BERT-based word representation augmented with user credibility information. The lower component of the framework models the conversation sequence of a claim in addition to the BERT-based user content representation to predict the stance of the underlying tweets. It employs a modified graph attention network, which models a conversation thread by finding tweets path-to-root conversation sequences. Further, the learned stance representation is augmented with the users' credibility information and content representation to predict rumor veracity. The experimental evaluation results over two benchmark datasets show that the proposed approach outperforms the state-of-the-art methods.
更多查看译文
关键词
Multitask Learning,Stance Detection,Rumor Veracity prediction,Joint Learning
AI 理解论文
溯源树
样例
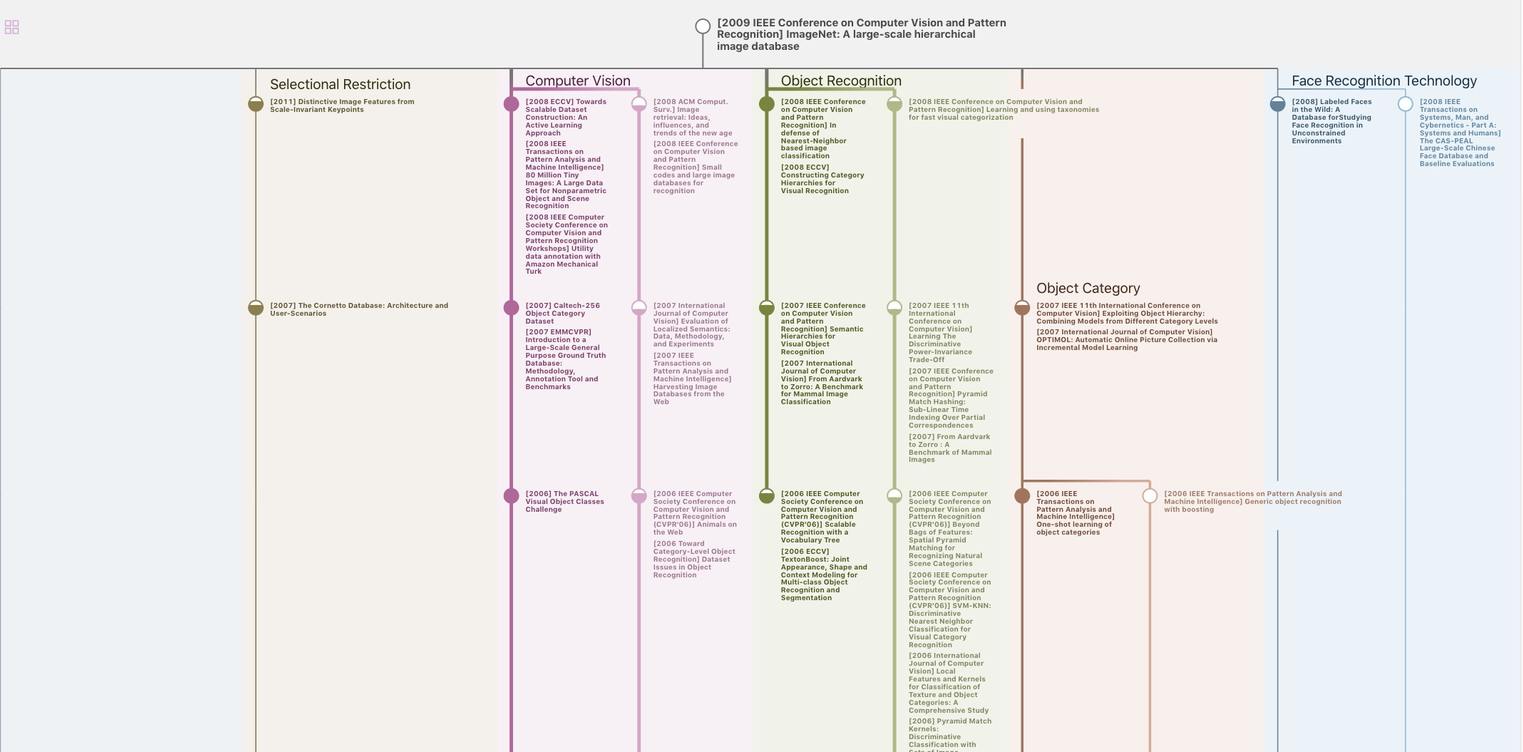
生成溯源树,研究论文发展脉络
Chat Paper
正在生成论文摘要