Cross-view multi-layer perceptron for incomplete multi-view learning
Applied Soft Computing(2024)
摘要
Incomplete multi-view learning (IML) is an important and challenging issue. The recent popular matrix factorization methods learn the representation matrix that contains as much complete information as possible from incomplete data. However, these works focus more on mining intrinsic information from the remaining views but fail to exploit the latent and connotative consistency, complementarity, and diversity information across views simultaneously. Meanwhile, the commonly used mean completer or deleting incomplete views strategy generates high uncertainty samples. To overcome these limits, this paper presents a Cross-View Multi-Layer Perceptron (CVMLP). CVMLP integrates an auto-encoder module, cross-view classification loss, masked contrastive learning, and variance loss into a unified framework to learn IML problems. The auto-encoder and cross-view modules efficiently express consistency and diversity across views, mining structural information from within views to between views. Masked contrastive loss makes the model robust to missing views by establishing a contrastive relationship between the input and random masked data. The variance loss can reduce the uncertainty of the classification hyperplane. Extensive experiments demonstrate that CVMLP achieves superior performance.
更多查看译文
关键词
Incomplete multi-view learning,Cross-view multi-layer perceptron,Contrastive learning
AI 理解论文
溯源树
样例
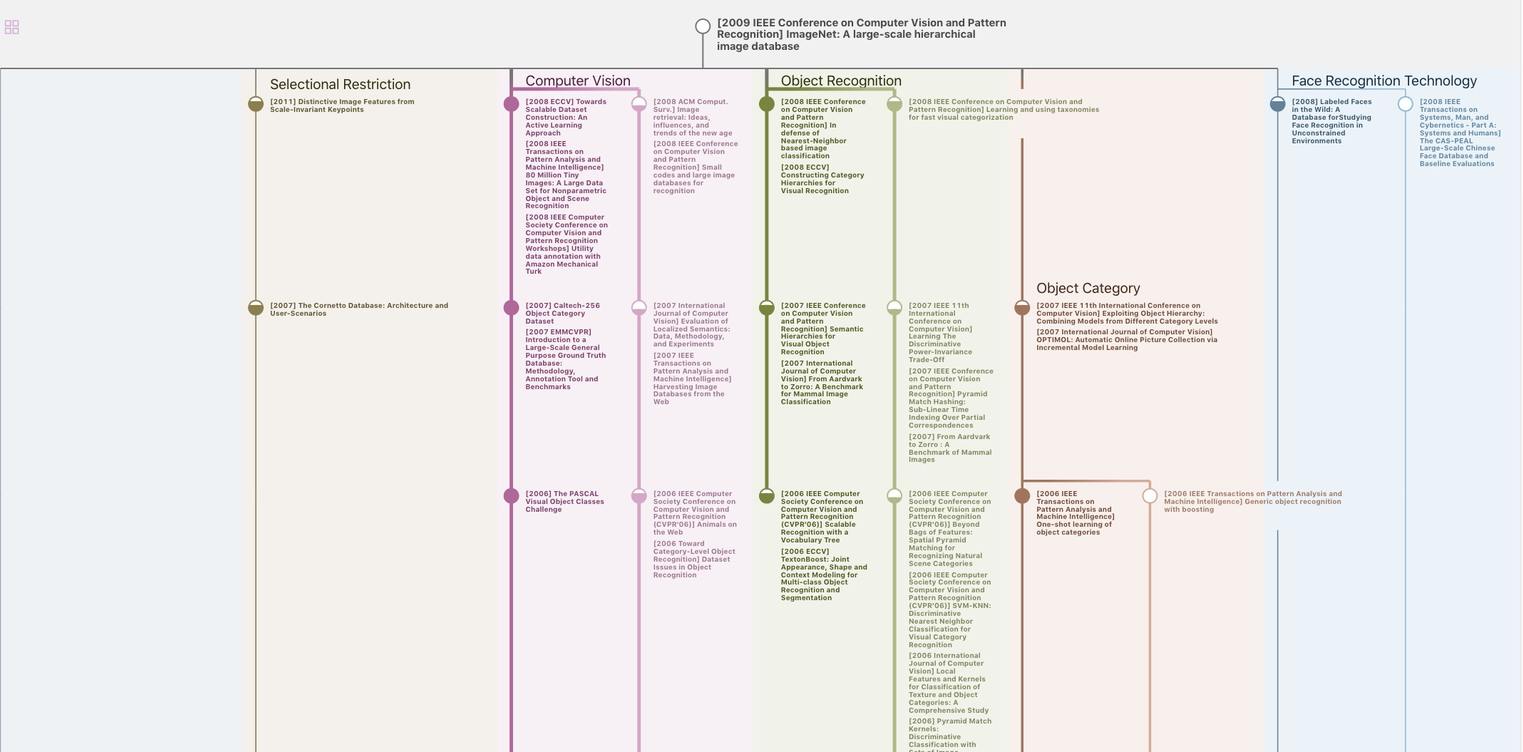
生成溯源树,研究论文发展脉络
Chat Paper
正在生成论文摘要