Fine-grained image classification based on TinyVit object location and graph convolution network
Journal of Visual Communication and Image Representation(2024)
摘要
Fine-grained image classification is a branch of image classification. Recently, vision transformer has made excellent progress in the field of image recognition. Its self-attention mechanism can extract very effective image feature information. However, feeding fixed-size image blocks into the network introduces additional noise, which is detrimental to extract discriminative features for fine-grained images. The vision transformer's network model is large, making it difficult to utilize in practice. Moreover, many of today's fine-grained image classification methods focus on mining discriminative features while ignoring the connections within the image. To address these problems, we propose a novel method based on the lightweight TinyVit backbone network. Our approach utilizes the self-attention weight values of TinyVit as a guide to construct an effective object location (OL) module that cuts and enlarges the object area, providing the network with the opportunity to concentrate on the local object. Additionally, we employ the graph convolutional network (GCN) to create a spatial relationship feature learning (SRFL) module that captures spatial context information between image blocks in TinyVit with the help of the transformer's self-attention weights. OL and SRFL collaborate to jointly guide the classification task. The experimental results show that the proposed method achieved competitive performance, with the second-highest classification faccuracy on both the CUB-200–2011 and NABirds datasets. When tested on the Stanford Dogs dataset, our approach outperformed many popular methods. Our code is uploaded on https://github.com/hhhj1999/SRFL_OL.
更多查看译文
关键词
Fine-grained image classification,TinyVit,Object location,Spatial relationship feature learning,Graph convolution network
AI 理解论文
溯源树
样例
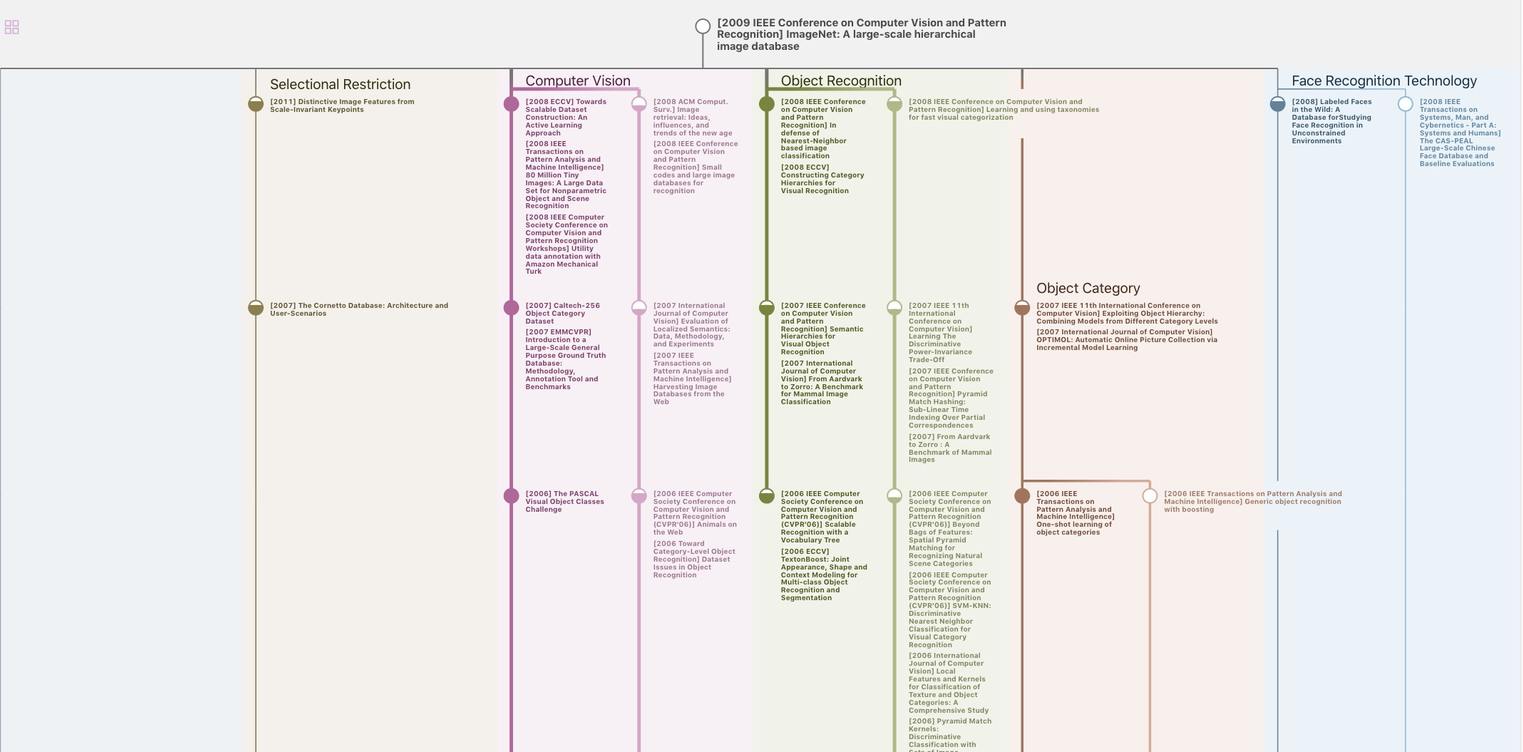
生成溯源树,研究论文发展脉络
Chat Paper
正在生成论文摘要