CLigopt: Controllable Ligand Design Through Target-Specific Optimisation
Bioinformatics (Oxford, England)(2024)
摘要
Motivation Key challenge in deep generative models for molecular design is to navigate random sampling of the vast molecular space, and produce promising molecules that compromise property controls across multiple chemical criteria. Fragment-based drug design (FBDD), using fragments as starting points, is an effective way to constrain chemical space and improve generation of biologically active molecules. Furthermore, optimisation approaches are often implemented with generative models to search through chemical space, and identify promising samples which satisfy specific properties. Controllable FBDD has promising potential in efficient target-specific ligand design. Results We propose a controllable FBDD model, CLigOpt, which can generate molecules with desired properties from a given fragment pair. CLigOpt is a Variational AutoEncoder-based model which utilises co-embeddings of node and edge features to fully mine information from molecular graphs, as well as a multi-objective Controllable Generation Module to generate molecules under property controls. CLigOpt achieves consistently strong performance in generating structurally and chemically valid molecules, as evaluated across six metrics. Applicability is illustrated through ligand candidates for hDHFR and it is shown that the proportion of feasible active molecules from the generated set is increased by 10%. Molecular docking and synthesisability prediction tasks are conducted to prioritise generated molecules to derive potential lead compounds. Availability and Implementation The source code is available via . ### Competing Interest Statement The authors have declared no competing interest.
更多查看译文
关键词
Molecular Docking
AI 理解论文
溯源树
样例
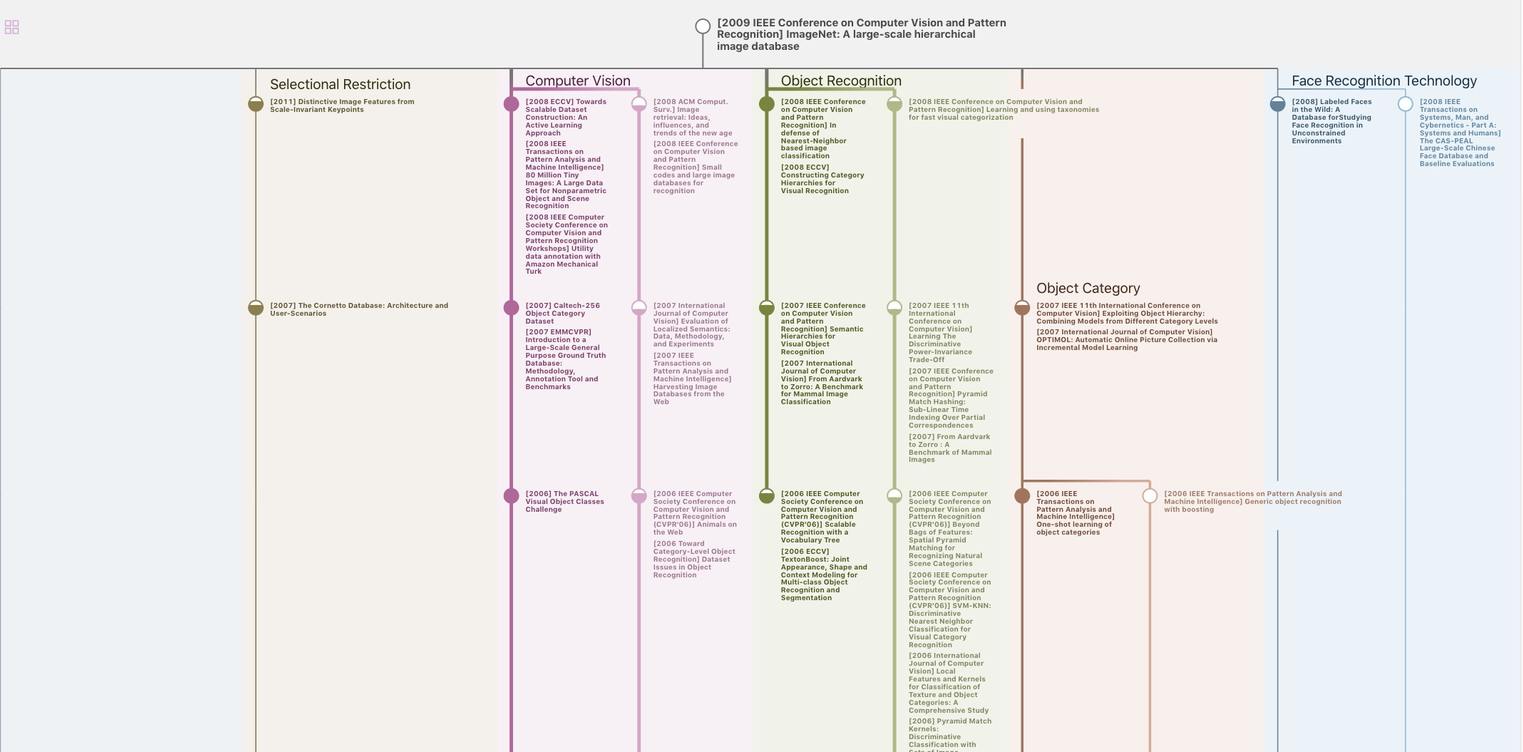
生成溯源树,研究论文发展脉络
Chat Paper
正在生成论文摘要