Diversifying Sequential Recommendation with Retrospective and Prospective Transformers.
ACM Trans. Inf. Syst.(2024)
Abstract
Previous studies on sequential recommendation (SR) have predominantly concentrated on optimizing recommendation accuracy. However, there remains a significant gap in enhancing recommendation diversity, particularly for short interaction sequences. The limited availability of interaction information in short sequences hampers the recommender’s ability to comprehensively model users’ intents, consequently affecting both the diversity and accuracy of recommendation. In light of the above challenge, we propose reTrospective and pRospective Transformers for dIversified sEquential Recommendation (TRIER) . The TRIER addresses the issue of insufficient information in short interaction sequences by first retrospectively learning to predict users’ potential historical interactions, thereby introducing additional information and expanding short interaction sequences, and then capturing users’ potential intents from multiple augmented sequences. Finally, the TRIER learns to generate diverse recommendation lists by covering as many potential intents as possible. To evaluate the effectiveness of TRIER, we conduct extensive experiments on three benchmark datasets. The experimental results demonstrate that TRIER significantly outperforms state-of-the-art methods, exhibiting diversity improvement of up to 11.36% in terms of intra-list distance (ILD@5) on the Steam dataset, 3.43% ILD@5 on the Yelp dataset and 3.77% in terms of category coverage (CC@5) on the Beauty dataset. As for accuracy, on the Yelp dataset, we observe notable improvement of 7.62% and 8.63% in HR@5 and NDCG@5, respectively. Moreover, we found that TRIER reveals more significant accuracy and diversity improvement for short interaction sequences.
MoreTranslated text
Key words
Retrospective transformer,prospective transformer,diverse,sequential recommendation
AI Read Science
Must-Reading Tree
Example
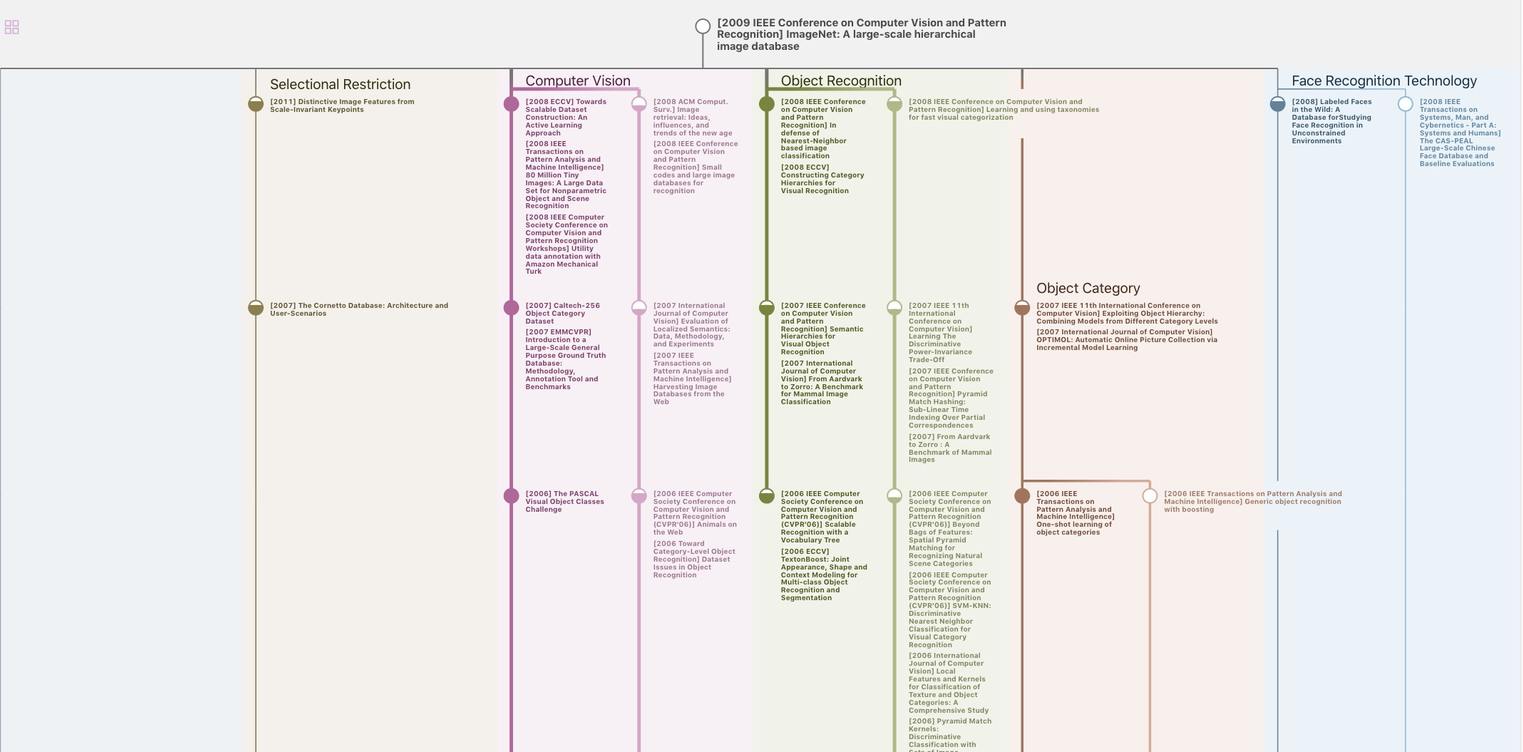
Generate MRT to find the research sequence of this paper
Chat Paper
Summary is being generated by the instructions you defined