FedComLoc: Communication-Efficient Distributed Training of Sparse and Quantized Models
CoRR(2024)
摘要
Federated Learning (FL) has garnered increasing attention due to its unique
characteristic of allowing heterogeneous clients to process their private data
locally and interact with a central server, while being respectful of privacy.
A critical bottleneck in FL is the communication cost. A pivotal strategy to
mitigate this burden is Local Training, which involves running multiple
local stochastic gradient descent iterations between communication phases. Our
work is inspired by the innovative Scaffnew algorithm, which has
considerably advanced the reduction of communication complexity in FL. We
introduce FedComLoc (Federated Compressed and Local Training), integrating
practical and effective compression into Scaffnew to further enhance
communication efficiency. Extensive experiments, using the popular TopK
compressor and quantization, demonstrate its prowess in substantially reducing
communication overheads in heterogeneous settings.
更多查看译文
AI 理解论文
溯源树
样例
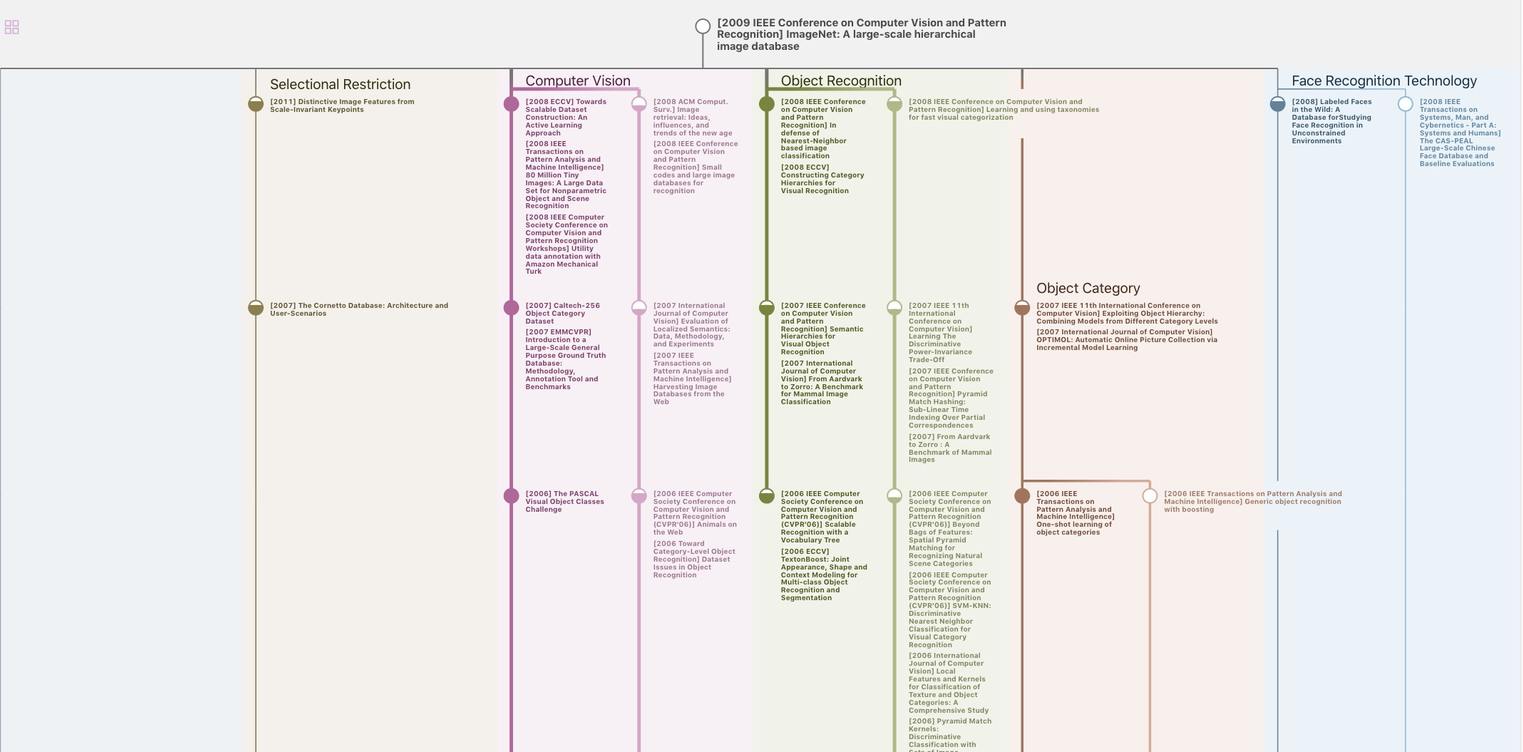
生成溯源树,研究论文发展脉络
Chat Paper
正在生成论文摘要