Grasp Anything: Combining Teacher-Augmented Policy Gradient Learning with Instance Segmentation to Grasp Arbitrary Objects
ICRA 2024(2024)
摘要
Interactive grasping from clutter, akin to human dexterity, is one of the longest-standing problems in robot learning. Challenges stem from the intricacies of visual perception, the demand for precise motor skills, and the complex interplay between the two. In this work, we present Teacher-Augmented Policy Gradient (TAPG), a novel two-stage learning framework that synergizes reinforcement learning and policy distillation. After training a teacher policy to master the motor control based on object pose information, TAPG facilitates guided, yet adaptive, learning of a sensorimotor policy, based on object segmentation. We zero-shot transfer from simulation to a real robot by using Segment Anything Model for promptable object segmentation. Our trained policies adeptly grasp a wide variety of objects from cluttered scenarios in simulation and the real world based on human-understandable prompts. Furthermore, we show robust zero-shot transfer to novel objects. Videos of our experiments are available at url{https://maltemosbach.github.io/grasp_anything}.
更多查看译文
关键词
Reinforcement Learning,Grasping,Sensorimotor Learning
AI 理解论文
溯源树
样例
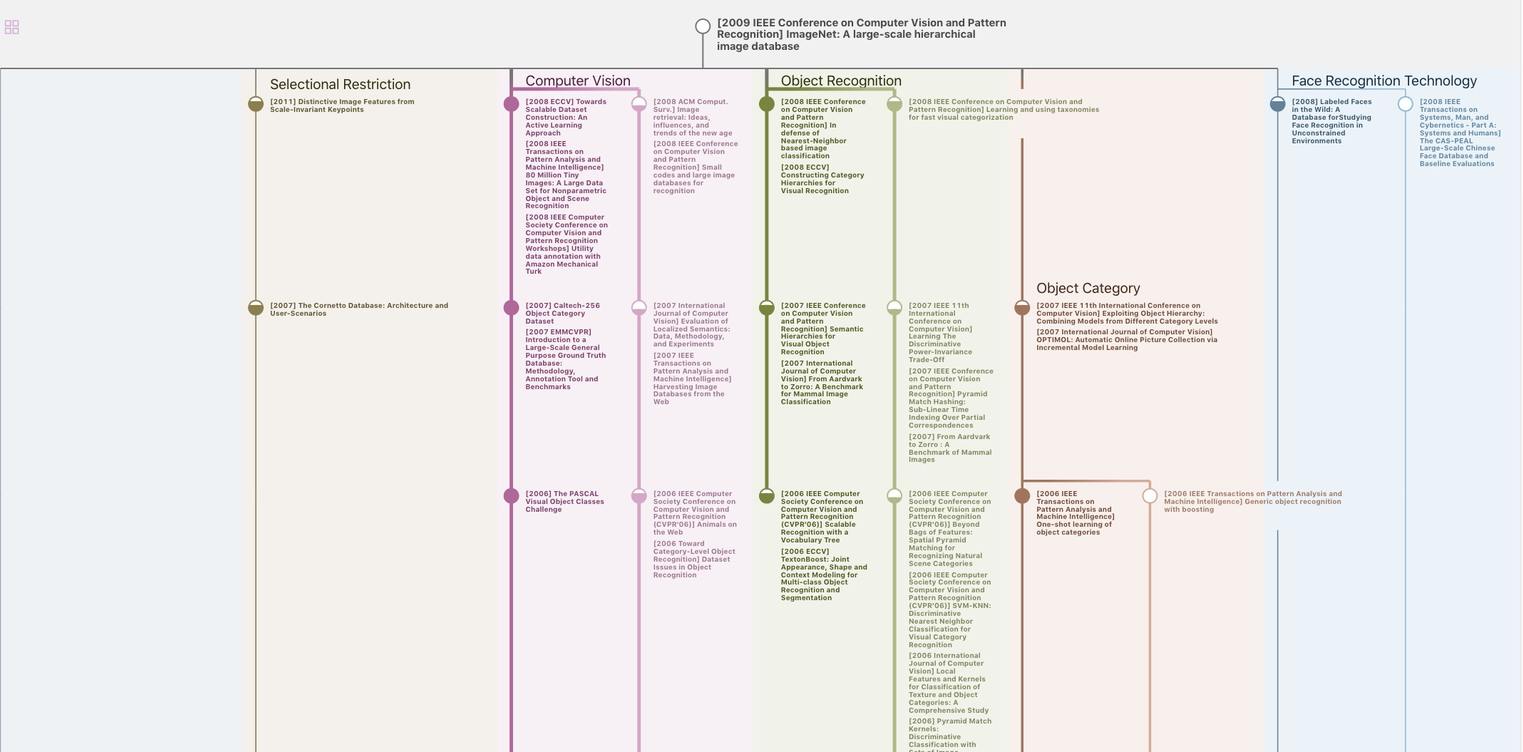
生成溯源树,研究论文发展脉络
Chat Paper
正在生成论文摘要