Learning of Nash Equilibria in Risk-Averse Games
arxiv(2024)
摘要
This paper considers risk-averse learning in convex games involving multiple
agents that aim to minimize their individual risk of incurring significantly
high costs. Specifically, the agents adopt the conditional value at risk (CVaR)
as a risk measure with possibly different risk levels. To solve this problem,
we propose a first-order risk-averse leaning algorithm, in which the CVaR
gradient estimate depends on an estimate of the Value at Risk (VaR) value
combined with the gradient of the stochastic cost function. Although estimation
of the CVaR gradients using finitely many samples is generally biased, we show
that the accumulated error of the CVaR gradient estimates is bounded with high
probability. Moreover, assuming that the risk-averse game is strongly monotone,
we show that the proposed algorithm converges to the risk-averse Nash
equilibrium. We present numerical experiments on a Cournot game example to
illustrate the performance of the proposed method.
更多查看译文
AI 理解论文
溯源树
样例
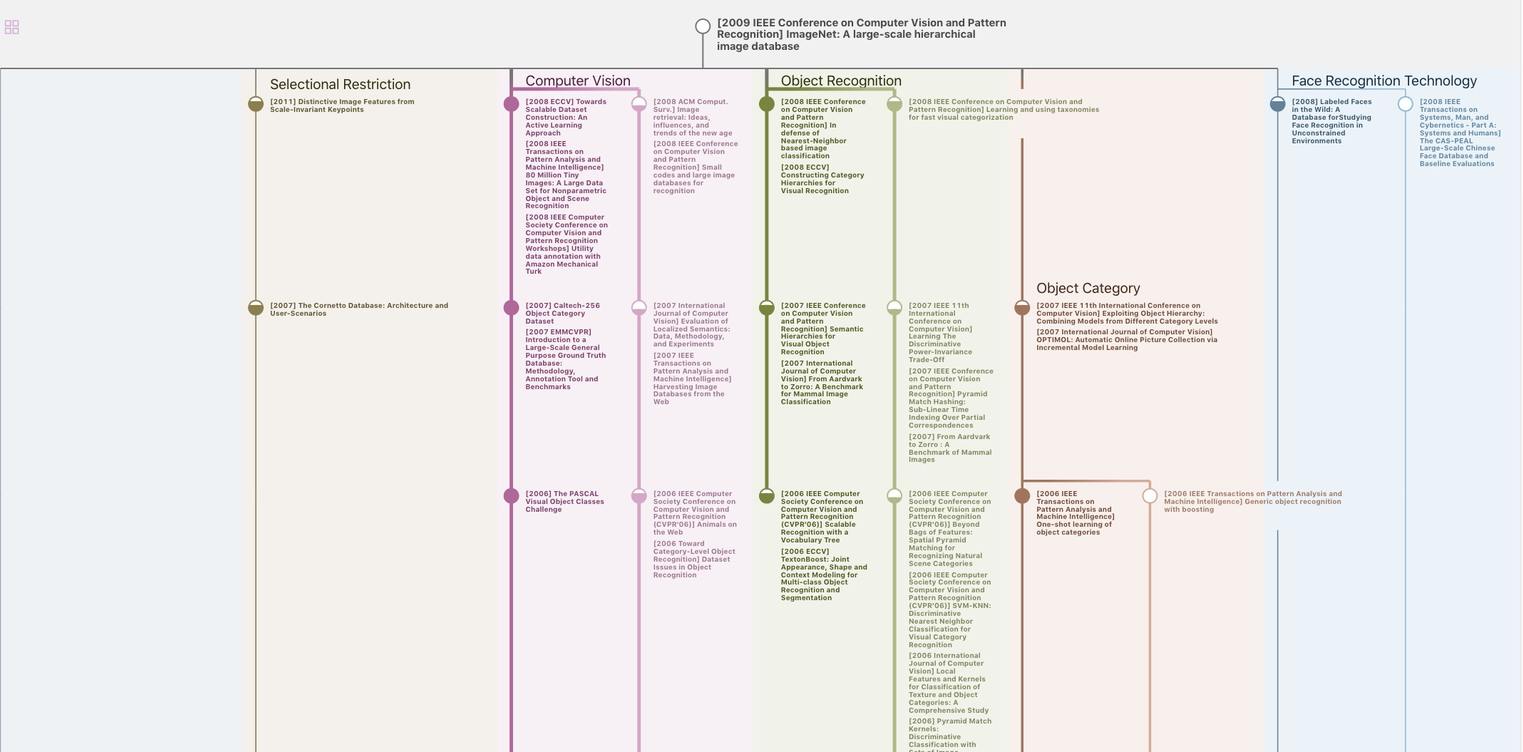
生成溯源树,研究论文发展脉络
Chat Paper
正在生成论文摘要