Flexible margins and multiple samples learning to enhance lexical semantic similarity
Engineering Applications of Artificial Intelligence(2024)
摘要
The advancement of deep learning and neural networks has led to the widespread adoption of neural word embeddings as a prominent lexical representation method in natural language processing. With the help of the neural language model trained by the contextual information of large scale text, the neural word embedding obtained by the neural language model captures more semantic correlation in the semantic space, while ignoring the semantic similarity. It will incur high computational cost and time costs during the training process of the model. To better inject semantic similarity into the distribution space and reduce time cost, we perform post processing learning of neural word embeddings using deep metric learning. This paper proposes a lexical enhancement method based on flexible margins and multiple samples learning. In this method, we embed the lexical entailment constraint relations into neural word embeddings. By categorizing the set of lexical constraints and penalizing the negative samples to different degrees according to the gap between categories, and allowing the positive and negative samples to learn from each other in the distributed space. The method we propose significantly improves neural word embeddings. By evaluating neural word embedded vocabulary similarity, the benchmark accuracy is improved to 75%. The method shows great competitiveness in text similarity tasks and text categorization tasks. These findings summarize research results and provide strong support for further applications.
更多查看译文
关键词
Semantic similarity,Deep metric learning,Post-processing method,Enhanced word embedding
AI 理解论文
溯源树
样例
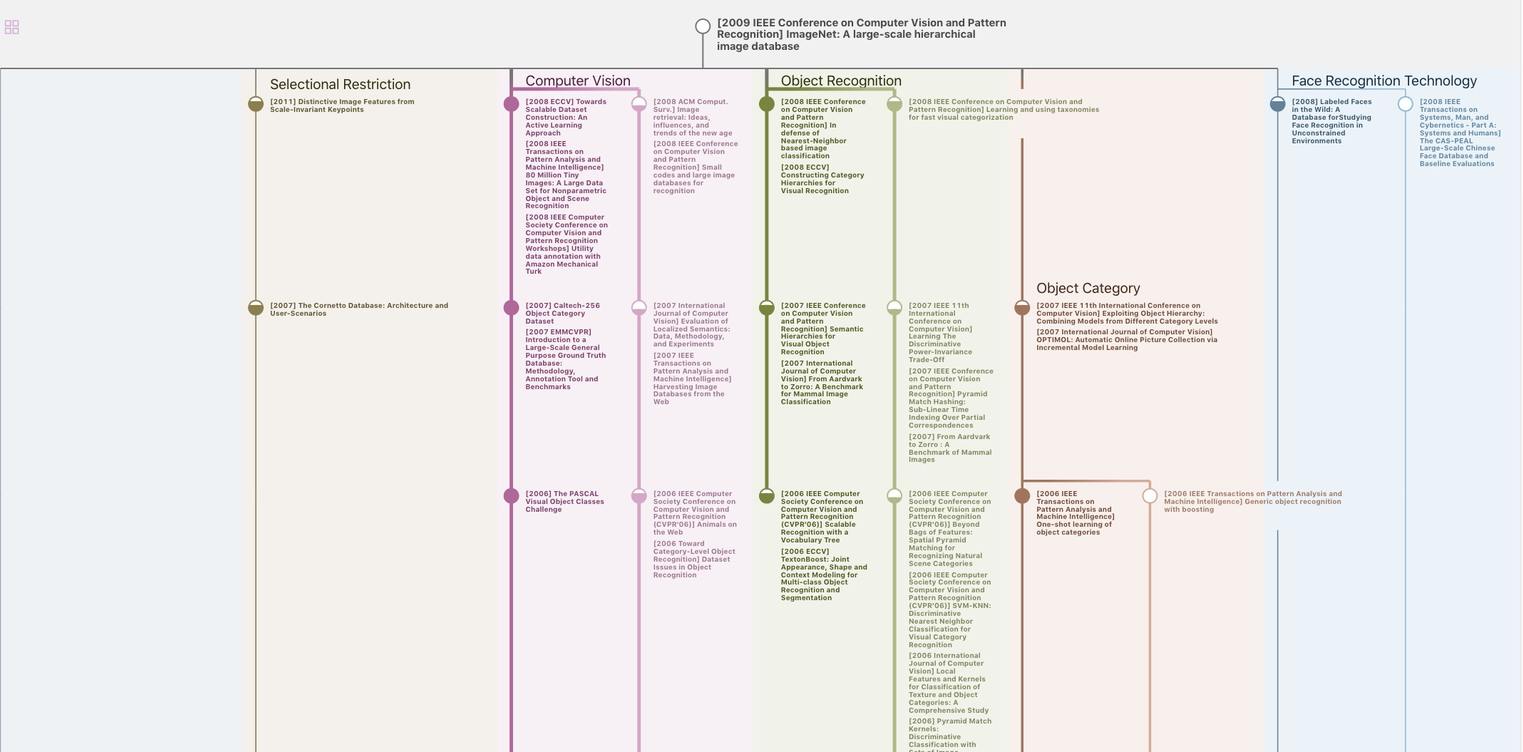
生成溯源树,研究论文发展脉络
Chat Paper
正在生成论文摘要