Unsupervised knowledge discovery framework: From AIS data processing to maritime traffic networks generating
Applied Ocean Research(2024)
摘要
With the continued growth in global maritime transportation, maritime situational awareness and traffic management have become increasingly crucial. To extract maritime traffic patterns from vast, low-quality Automatic Identification System (AIS) data, an unsupervised knowledge mining framework is proposed for generating a maritime traffic network. First, the framework incorporates an AIS data pre-processing algorithm that can generate high-quality, spatiotemporally continuous maritime trajectory data from low-quality inputs. Second, it extracts and categorizes anchoring and berthing trajectories, leveraging the spatial distribution of the trajectories to generate maritime stopping areas with functional semantic distributions. Furthermore, a genetic algorithm based on quadtree spatial dissection is used to extract turning areas in large maritime regions. Finally, a maritime traffic network comprising feature regions and typical routes is established. An experiment was conducted on the 2019 AIS data released by the National Oceanic and Atmospheric Administration of the United States to validate the effectiveness of this method. The results confirm that the proposed approach efficiently extracts high-quality maritime traffic networks from large-scale, low-quality AIS data.
更多查看译文
关键词
Maritime traffic network,Knowledge mining framework,Maritime stopping area extraction,Maritime turning area extraction,Typical route generation
AI 理解论文
溯源树
样例
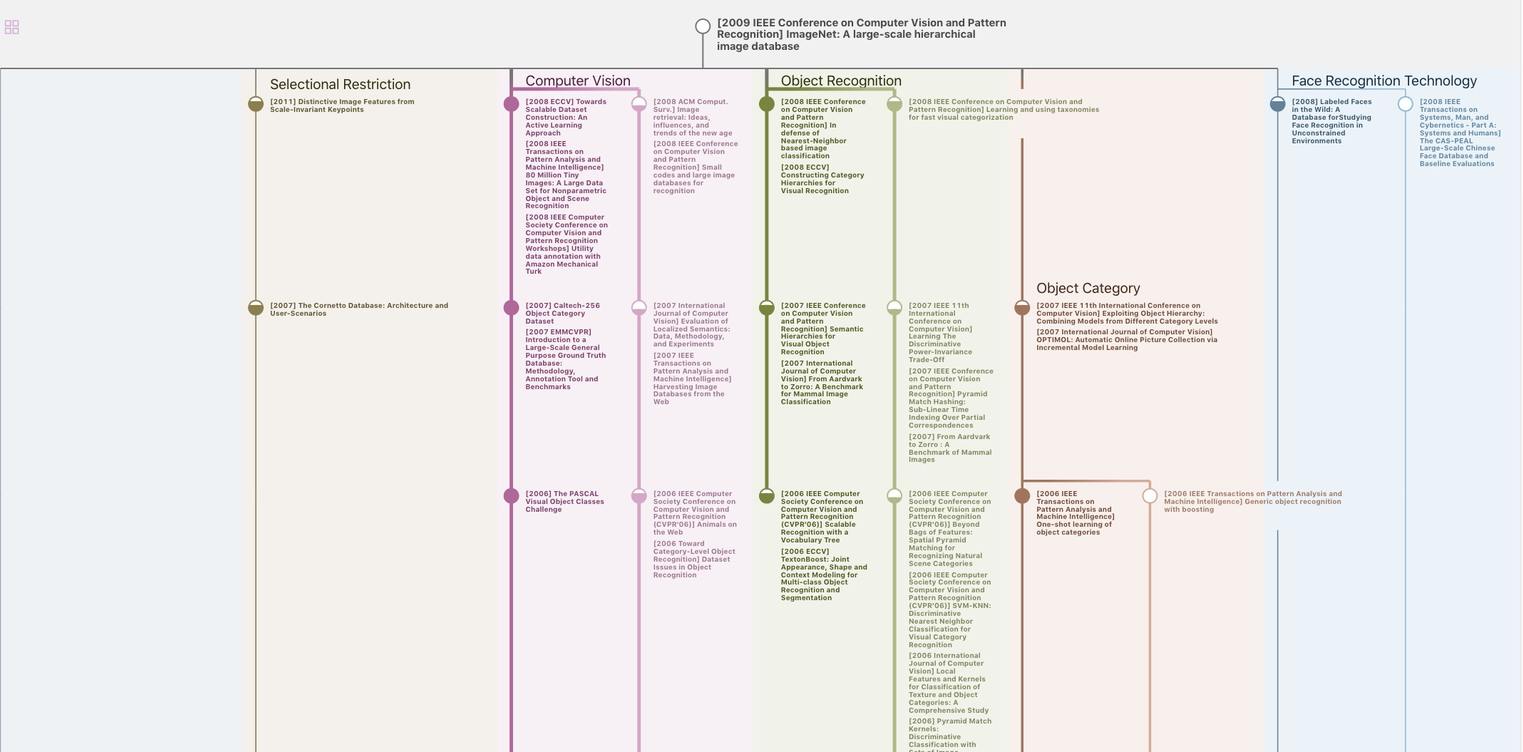
生成溯源树,研究论文发展脉络
Chat Paper
正在生成论文摘要