DEEPOREDNET: Contrastive Learning-Based Attention-Weighted Dual Channel Residual Network for Ocular Redness Assessment
ICASSP 2024 - 2024 IEEE International Conference on Acoustics, Speech and Signal Processing (ICASSP)(2024)
Abstract
Ocular redness is highly prevalent worldwide and often accompanied by pain, discomfort, and vision problems, making it an essential signal for monitoring disease development and prognosis. Understanding the category of ocular redness is crucial for health. However, the intricate vascular structure of the ocular surface poses challenges in extracting meaningful features through conventional methods. Moreover, the subtle variations in the signs contribute to the difficulty in achieving accurate discrimination. In this paper, we propose a novel approach named contrastive learning-based attention-weighted dual channel residual network (DeepORedNet) to address the challenging problem. The effectiveness of the proposed network architecture has been validated through detailed experiments. Our proposed DeepORedNet achieves superior performance compared to the baseline models across all evaluation metrics. We hope the proposed framework can effectively facilitate the clinic assessment of ocular redness.
MoreTranslated text
Key words
Ocular Redness,Contrastive Learning,Attention,Residual Network
AI Read Science
Must-Reading Tree
Example
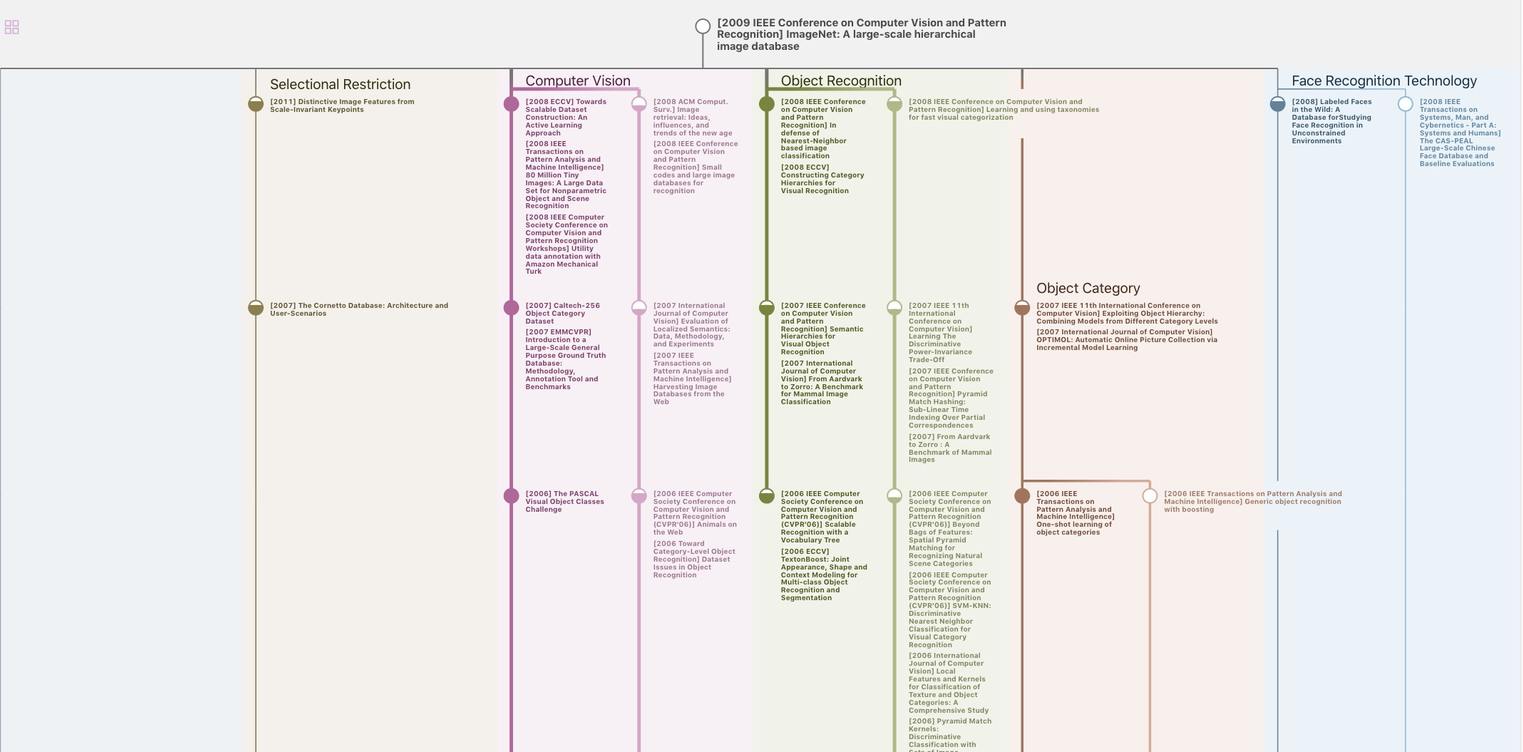
Generate MRT to find the research sequence of this paper
Chat Paper
Summary is being generated by the instructions you defined