HOICS: Zero-Shot Hoi Detection via Compatibility Self-Learning
ICASSP 2024 - 2024 IEEE International Conference on Acoustics, Speech and Signal Processing (ICASSP)(2024)
摘要
In recent years, the computer vision community has increasingly focused on detecting and recognizing human-object interactions (HOIs), which are crucial for tasks such as action recognition and scene understanding. However, the imbalanced distribution of interaction categories presents challenges, especially in scenarios with limited data. To address this issue, we propose an innovative end-to-end parallel HOI detection framework that incorporates compatibility self-learning (HOICS) for zero-shot HOI detection. Specifically, our approach introduces a compatibility self-learning strategy that allows the model to extract insights from HOI compatibility data and refine the interaction prediction head. This method involves utilizing combination probabilities and compatibility scores during the self-learning process, which significantly enhances zero-shot detection performance. Additionally, HOICS employs mosaic augmentation to broaden the model’s capabilities. Our extensive experiments on benchmark datasets demonstrate HOICS’ superiority over state-of-the-art methods, achieving a noteworthy 2.16 percent improvement in zero-shot HOI detection for previously unseen categories.
更多查看译文
关键词
human-object interaction,zero-shot detection,self-learning
AI 理解论文
溯源树
样例
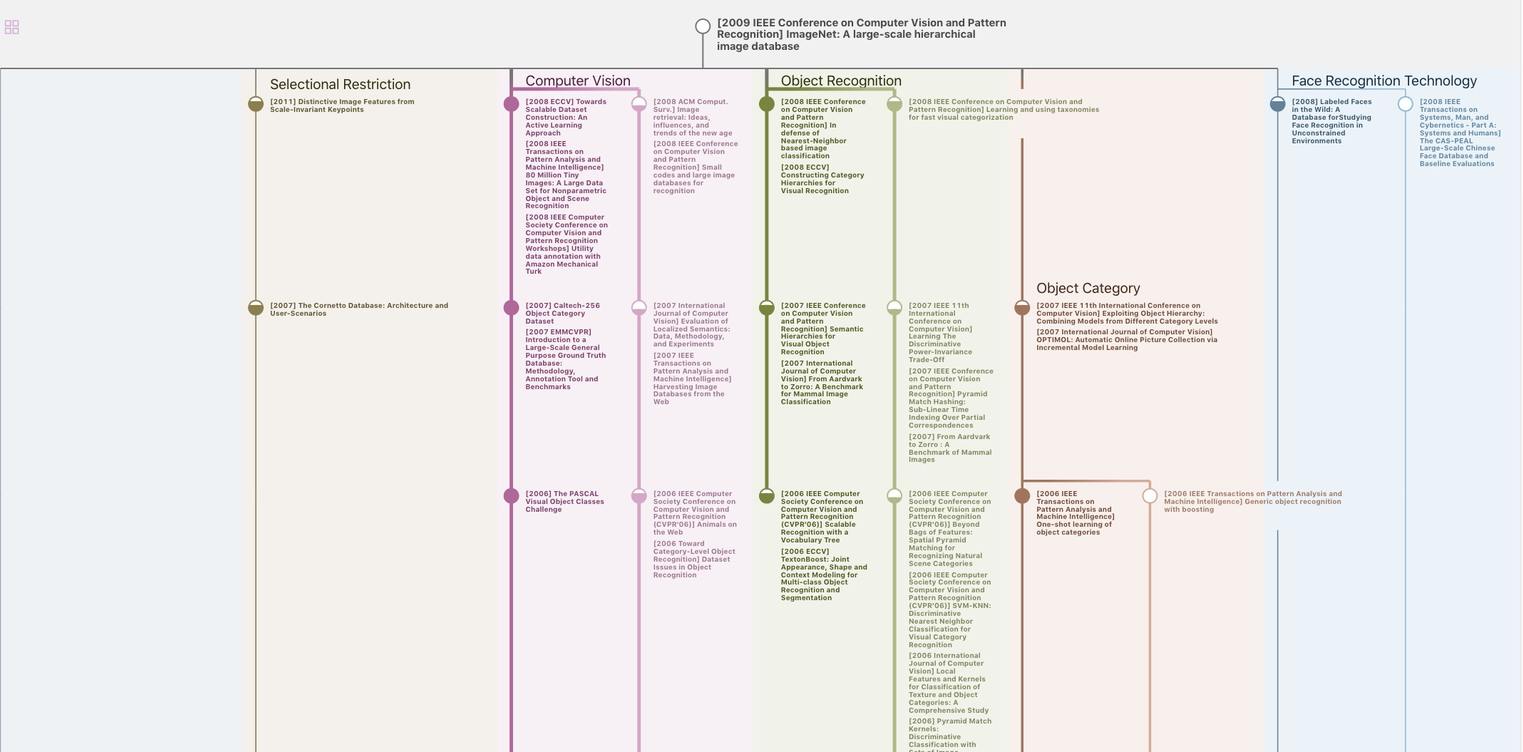
生成溯源树,研究论文发展脉络
Chat Paper
正在生成论文摘要