PolarDB: Formula-Driven Dataset for Pre-Training Trajectory Encoders
ICASSP 2024 - 2024 IEEE International Conference on Acoustics, Speech and Signal Processing (ICASSP)(2024)
摘要
Formula-driven supervised learning (FDSL) is a growing research topic for finding simple mathematical formulas that generate synthetic data and labels for pre-training neural networks. The main advantage of FDSL is that there is no risk of generating data with ethical implications such as gender bias and racial bias because it does not rely on real data as discussed in previous studies using fractals and polygons for pre-training image encoders. While FDSL has been proposed for pre-training image encoders, it has not been considered for temporal trajectory data. In this paper, we introduce PolarDB, the first formula-driven dataset for pre-training trajectory encoders with an application to fine-grained cutting-method recognition using hand trajectories. More specifically, we generate 270k trajectories for 432 categories on the basis of polar equations and use them to pre-train a Transformer-based trajectory encoder in an FDSL manner. In the experiments, we show that pre-training on PolarDB improves the accuracy of fine-grained cutting-method recognition on cooking videos of EPIC-KITCHEN and Ego4D datasets, where the pre-trained trajectory encoder is used as a plug-in module for a video recognition network.
更多查看译文
关键词
Formula-driven supervised learning,Polar equations,Fine-grained action recognition,Cutting method recognition
AI 理解论文
溯源树
样例
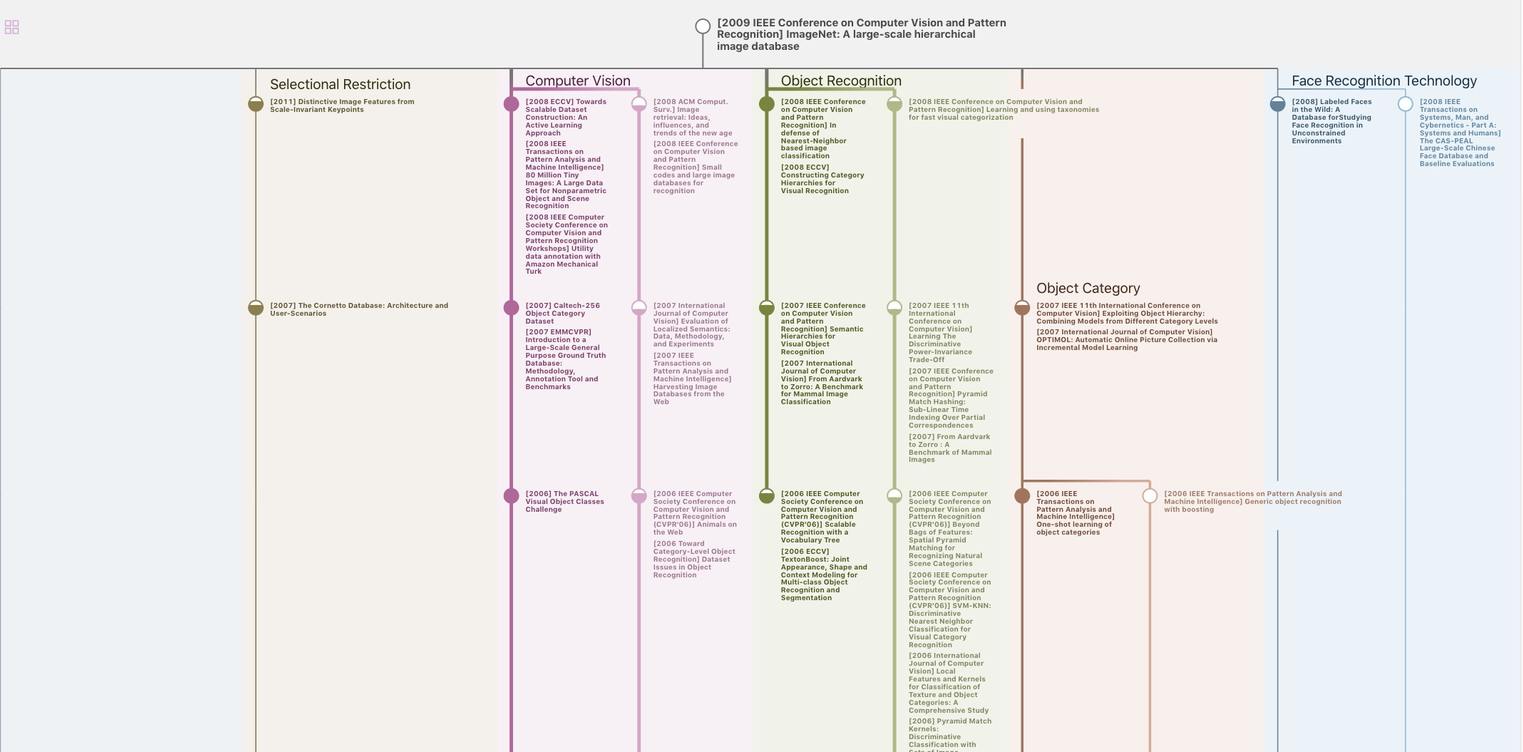
生成溯源树,研究论文发展脉络
Chat Paper
正在生成论文摘要