Asymptotically Tight Misspecified Bayesian Cramér-Rao Bound
ICASSP 2024 - 2024 IEEE International Conference on Acoustics, Speech and Signal Processing (ICASSP)(2024)
摘要
In many applications of estimation theory, the true data model is not perfectly known, leading to mismatch between the assumed model used for parameter estimation and the actual model. The non-Bayesian misspecified Cramér-Rao bound (MCRB) allows considering the effect of model misspecification on the estimator performance, and it has been extended to the Bayesian framework. Unlike the non-Bayesian MCRB, the corresponding Bayesian bound is asymptotically unattainable. In this paper, we derive an asymptotically tight misspecified Bayesian Cramér-Rao bound. We demonstrate that under some mild and common regularity conditions, this bound is asymptotically achieved by the maximum a-posteriori probability (MAP) estimator. The proposed bound is applied to the problems of variance estimation and direction-of-arrival estimation under model misspecification, illustrating its asymptotic attainability by the MAP estimator.
更多查看译文
关键词
Bayesian bounds,mean-squared-error,model misspecification,misspecified Cramér-Rao bound (MCRB)
AI 理解论文
溯源树
样例
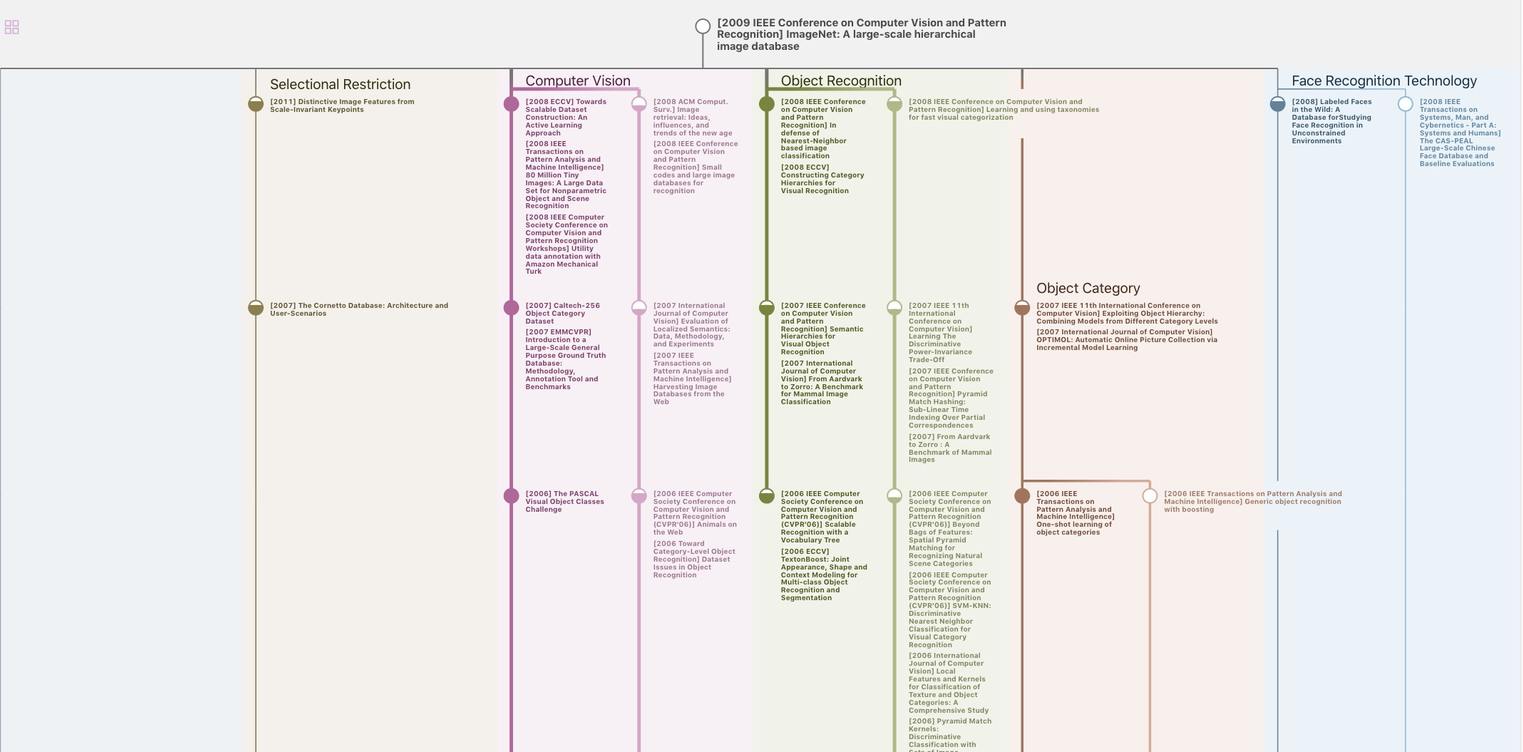
生成溯源树,研究论文发展脉络
Chat Paper
正在生成论文摘要