A Multimodal Approach to Device-Directed Speech Detection with Large Language Models
ICASSP 2024 - 2024 IEEE International Conference on Acoustics, Speech and Signal Processing (ICASSP)(2024)
摘要
Interactions with virtual assistants typically start with a predefined
trigger phrase followed by the user command. To make interactions with the
assistant more intuitive, we explore whether it is feasible to drop the
requirement that users must begin each command with a trigger phrase. We
explore this task in three ways: First, we train classifiers using only
acoustic information obtained from the audio waveform. Second, we take the
decoder outputs of an automatic speech recognition (ASR) system, such as 1-best
hypotheses, as input features to a large language model (LLM). Finally, we
explore a multimodal system that combines acoustic and lexical features, as
well as ASR decoder signals in an LLM. Using multimodal information yields
relative equal-error-rate improvements over text-only and audio-only models of
up to 39
adaption leads to further relative EER reductions of up to 18
更多查看译文
关键词
device-directed speech detection,large language model,multimodal,conditional generation
AI 理解论文
溯源树
样例
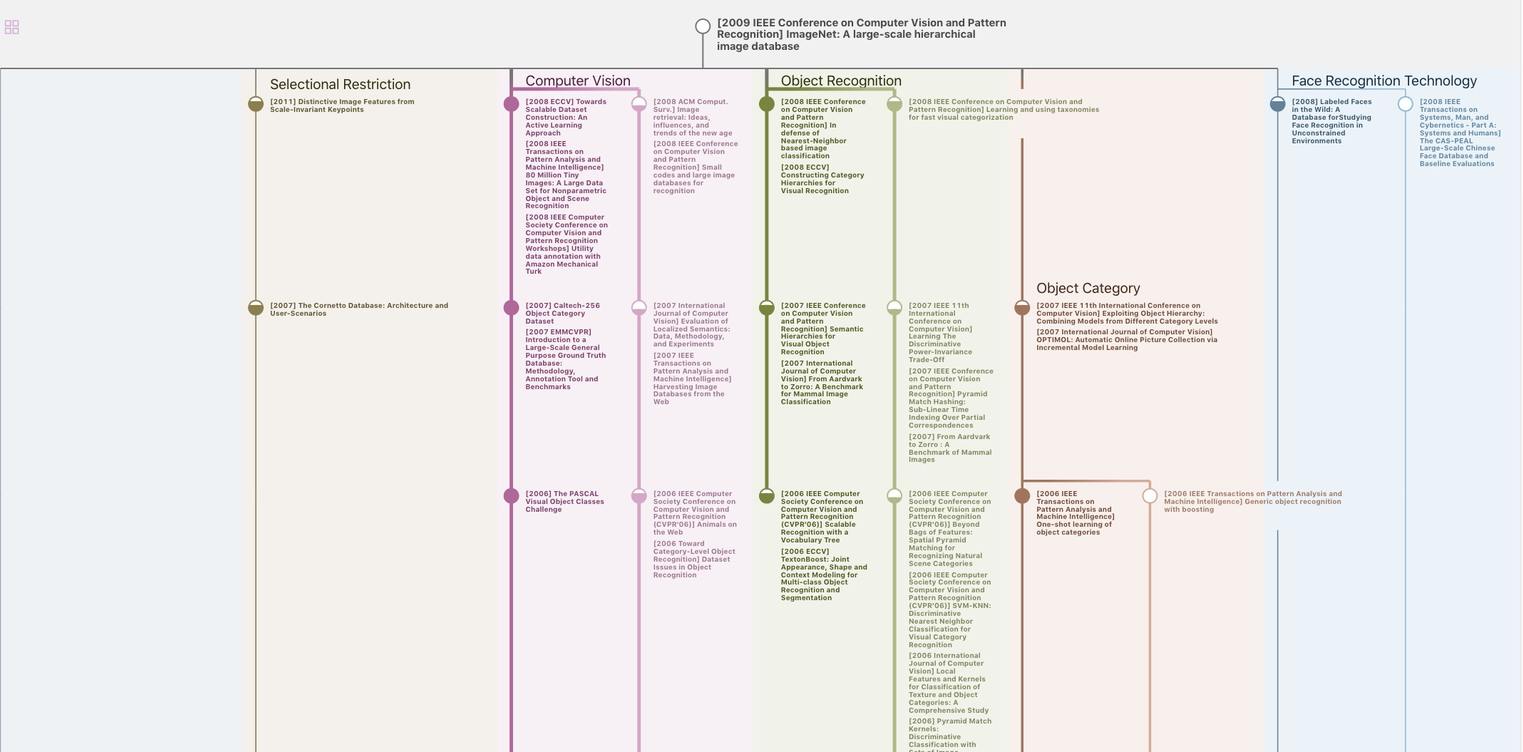
生成溯源树,研究论文发展脉络
Chat Paper
正在生成论文摘要