Data-Based Filters for Non-Gaussian Dynamic Systems with Unknown Output Noise Covariance
IEEE-CAA JOURNAL OF AUTOMATICA SINICA(2024)
摘要
This paper proposes linear and nonlinear filters for a non-Gaussian dynamic system with an unknown nominal covariance of the output noise. The challenge of designing a suitable filter in the presence of an unknown covariance matrix is addressed by focusing on the output data set of the system. Considering that data generated from a Gaussian distribution exhibit ellipsoidal scattering, we first propose the weighted sum of norms (SON) clustering method that prioritizes nearby points, reduces distant point influence, and lowers computational cost. Then, by introducing the weighted maximum likelihood, we propose a semi-definite program (SDP) to detect outliers and reduce their impacts on each cluster. Detecting these weights paves the way to obtain an appropriate covariance of the output noise. Next, two filtering approaches are presented: a cluster-based robust linear filter using the maximum a posterior (MAP) estimation and a cluster-based robust nonlinear filter assuming that output noise distribution stems from some Gaussian noise resources according to the ellipsoidal clusters. At last, simulation results demonstrate the effectiveness of our proposed filtering approaches.
更多查看译文
关键词
Data-based filter,maximum likelihood estimation,unknown covariance,weighted maximum likelihood estimation,weighted sum-of-norms clustering
AI 理解论文
溯源树
样例
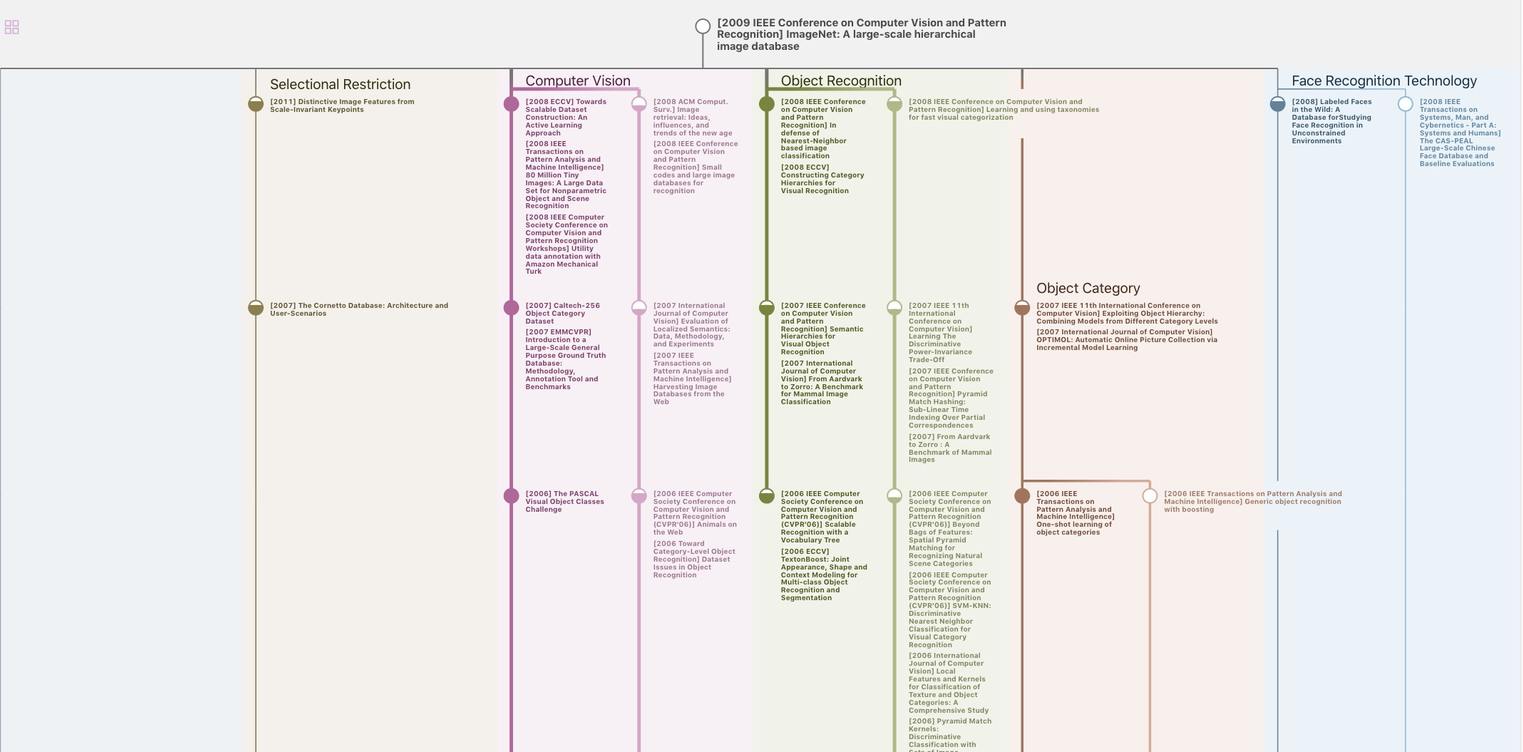
生成溯源树,研究论文发展脉络
Chat Paper
正在生成论文摘要