DHyper: A Recurrent Dual Hypergraph Neural Network for Event Prediction in Temporal Knowledge Graphs
ACM Transactions on Information Systems(2024)
摘要
Event prediction is a vital and challenging task in temporal knowledge graphs (TKGs), which have played crucial roles in various applications. Recently, many graph neural networks based approaches are proposed to model the graph structure information in TKGs. However, these approaches only construct graphs based on quadruplets and model the pairwise correlation between entities, which fail to capture the high-order correlations among entities. To this end, we propose DHyper, a recurrent Dual Hypergraph neural network for event prediction in TKGs, which simultaneously models the influences of the high-order correlations among both entities and relations. Specifically, a dual hypergraph learning module is proposed to discover the high-order correlations among entities and among relations in a parameterized way. A dual hypergraph message passing network is introduced to perform the information aggregation and representation fusion on the entity hypergraph and the relation hypergraph. Extensive experiments on six real-world datasets demonstrate that DHyper achieves the state-of-the-art performances, outperforming the best baseline by an average of 13.09%, 4.26%, 17.60%, and 18.03% in MRR, Hits@1, Hits@3, and Hits@10, respectively.
更多查看译文
关键词
Event prediction,temporal knowledge graphs,hypergraph,graph neural networks
AI 理解论文
溯源树
样例
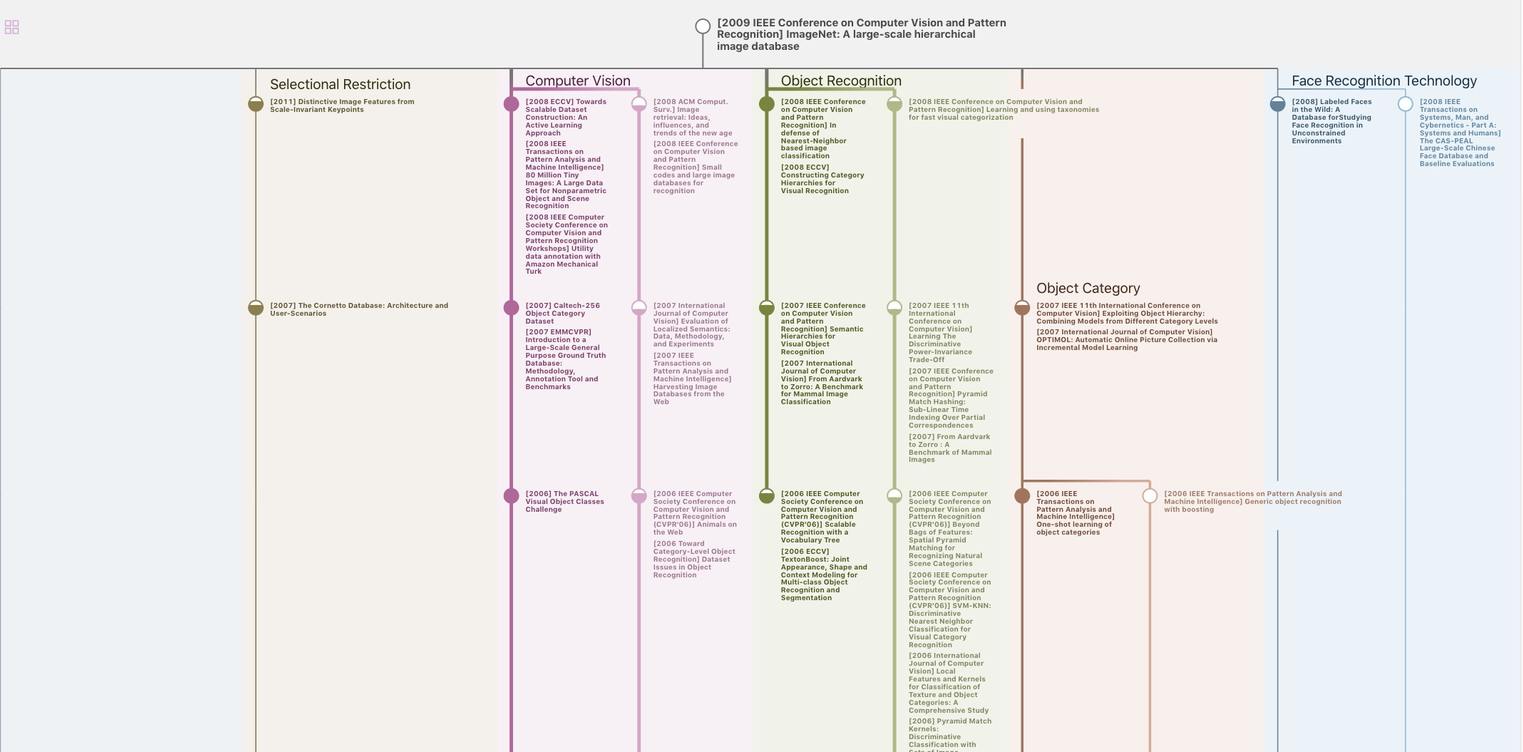
生成溯源树,研究论文发展脉络
Chat Paper
正在生成论文摘要