Fair Consensus in Blockchain with Heterogeneous Miners using Reinforcement Learning aided Adaptive Proof-of-Work
2024 IEEE 21ST CONSUMER COMMUNICATIONS & NETWORKING CONFERENCE, CCNC(2024)
摘要
Blockchain technology relies on Peer-to-Peer (P2P) ledgers that require a mechanism to maintain security and ensure consensus. The Proof of Work (PoW) consensus algorithm requires participants to dedicate significant computation to solve a cryptography puzzle. The Proof of Work Difficulty (PoW-D) in large public blockchains, such as Ethereum, is adjusted based on the time taken by the winning miner to mine the last block since the system is unaware of the computation power in the blockchain. The consortium and private blockchains provide greater visibility of the nodes involved, which may have variable compute power over time. Such heterogeneity in miners makes it challenging to maintain fairness leading to super linear profits. To address these issues, we propose two novel Reinforcement Learning (RL)-based techniques to intelligently select miners and adaptively update the PoW-D based on the variable compute power of the heterogeneous miners - RL based Miner Selection (RL-MS) and RL based Miner and Difficulty Selection (RL-MDS). Compared to the existing PoW techniques, the proposed approach lowered the winning percentage of the miners below a fairness factor threshold. Furthermore, the block mining time constraint violation is significantly reduced by up to 97% and up to 85% in RL-MS and RL-MDS, respectively.
更多查看译文
关键词
Blockchain,Proof ofWork,Consensus,Fairness,Federated Learning,Reinforcement Learning
AI 理解论文
溯源树
样例
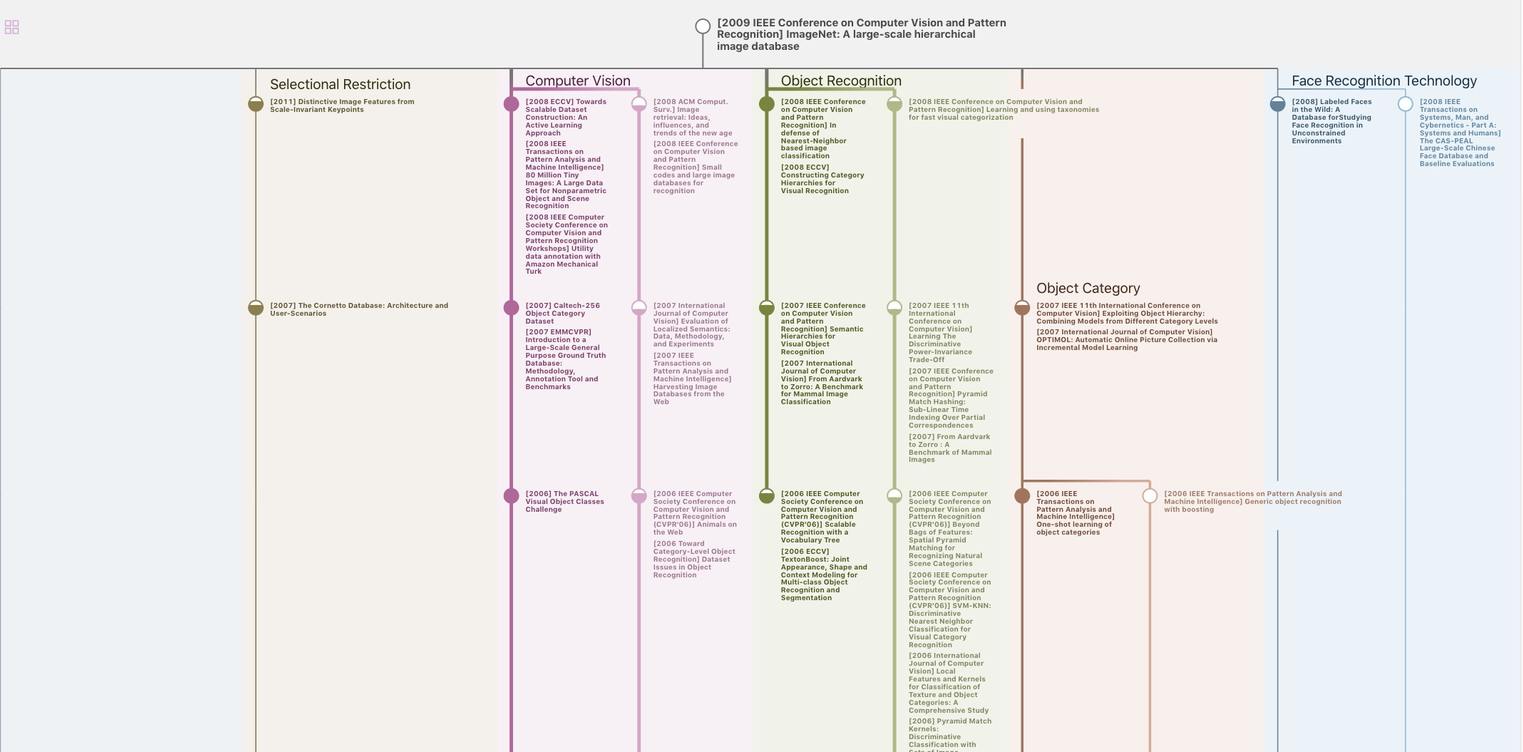
生成溯源树,研究论文发展脉络
Chat Paper
正在生成论文摘要