Assessment of a machine-learning software for classifying ground cover
SOUTHWESTERN NATURALIST(2023)
摘要
Estimating and classifying ground cover is a common component of many ecological field studies, but traditional methods are time-consuming and may introduce researcher bias. We assessed the use of a novel machine-learning software to estimate and classify ground cover in the semiarid landscape of the High Plains of Texas. We compared several types of software estimation to classification of ground cover in the field by a trained ecologist. We found high correlations between two out of three classification models. On-site category training had the highest correlation in the litter-dead vegetation ground cover category. Micro image training had the highest correlation in the bare ground cover category. Traditional training produced a model that was highly uncorrelated in all ground cover categories compared with the trained ecologist classification. We found ImageJ plus WEKA segmentation to be a valuable tool for research projects that require standardized ground cover classification across multiple samples at a low cost, compared with manually classifying ground cover, especially when time in the field is at a premium.
更多查看译文
AI 理解论文
溯源树
样例
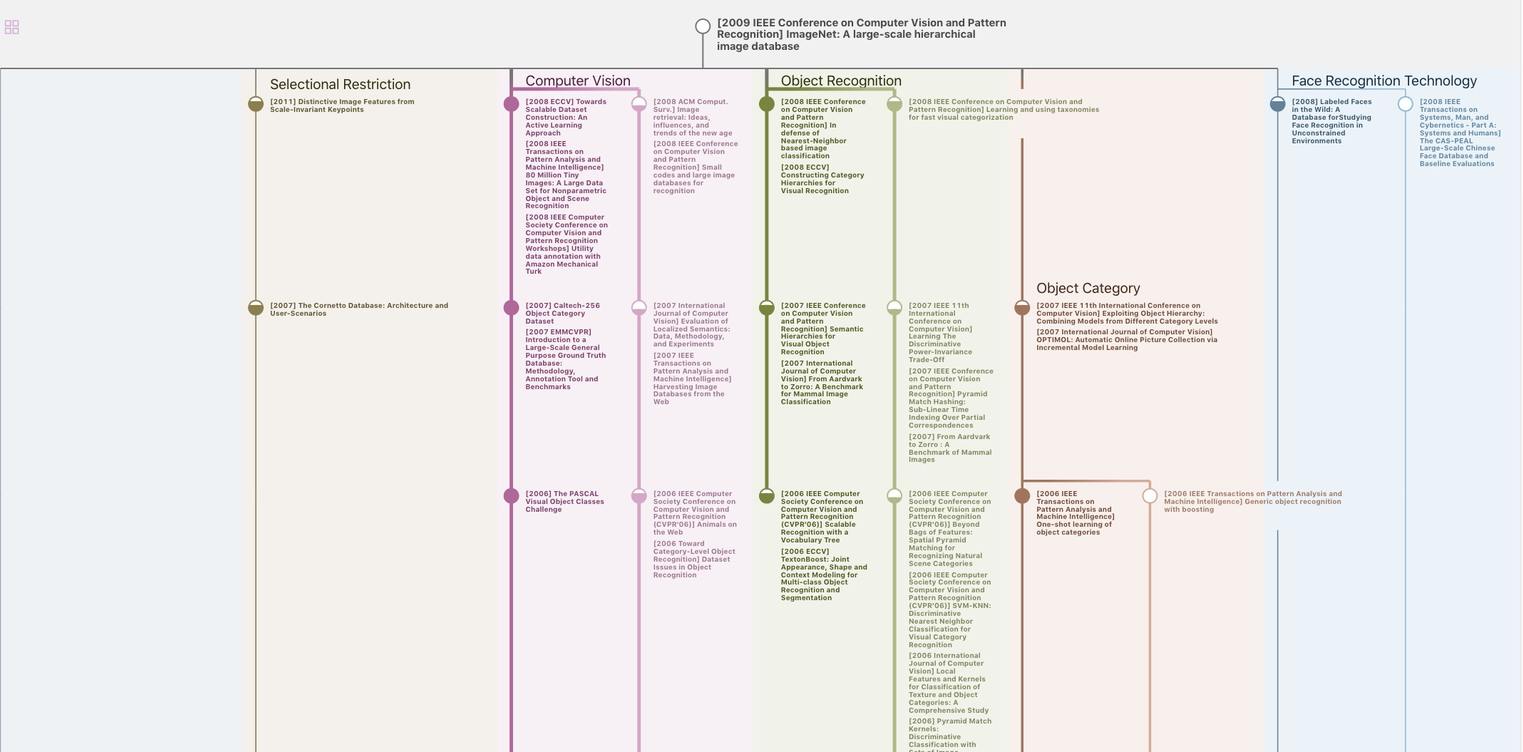
生成溯源树,研究论文发展脉络
Chat Paper
正在生成论文摘要