A deep transfer learning approach for sleep stage classification and sleep apnea detection using wrist-worn consumer sleep technologies.
IEEE transactions on bio-medical engineering(2024)
摘要
Obstructive sleep apnea (OSA) is a common, underdiagnosed sleep-related breathing disorder with serious health implications Objective - We propose a deep transfer learning approach for sleep stage classification and sleep apnea (SA) detection using wrist-worn consumer sleep technologies (CST). Methods - Our model is based on a deep convolutional neural network (DNN) utilizing accelerometers and photo-plethysmography signals from nocturnal recordings. The DNN was trained and tested on internal datasets that include raw data from clinical and wrist-worn devices; external validation was performed on a hold-out test dataset containing raw data from a wrist-worn CST. Results - Training on clinical data improves performance significantly, and feature enrichment through a sleep stage stream gives only minor improvements. Raw data input outperforms feature-based input in CST datasets. The system generalizes well but performs slightly worse on wearable device data compared to clinical data. However, it excels in detecting events during REM sleep and is associated with arousal and oxygen desaturation. We found; cases that were significantly underestimated were characterized by fewer of such event associations. Conclusion - This study showcases the potential of using CSTs as alternate screening solution for undiagnosed cases of OSA. Significance - This work is significant for its development of a deep transfer learning approach using wrist-worn consumer sleep technologies, offering comprehensive validation for data utilization, and learning techniques, ultimately improving sleep apnea detection across diverse devices.
更多查看译文
关键词
deep learning,sleep stage classification,consumer sleep technologies,sleep disordered breathing
AI 理解论文
溯源树
样例
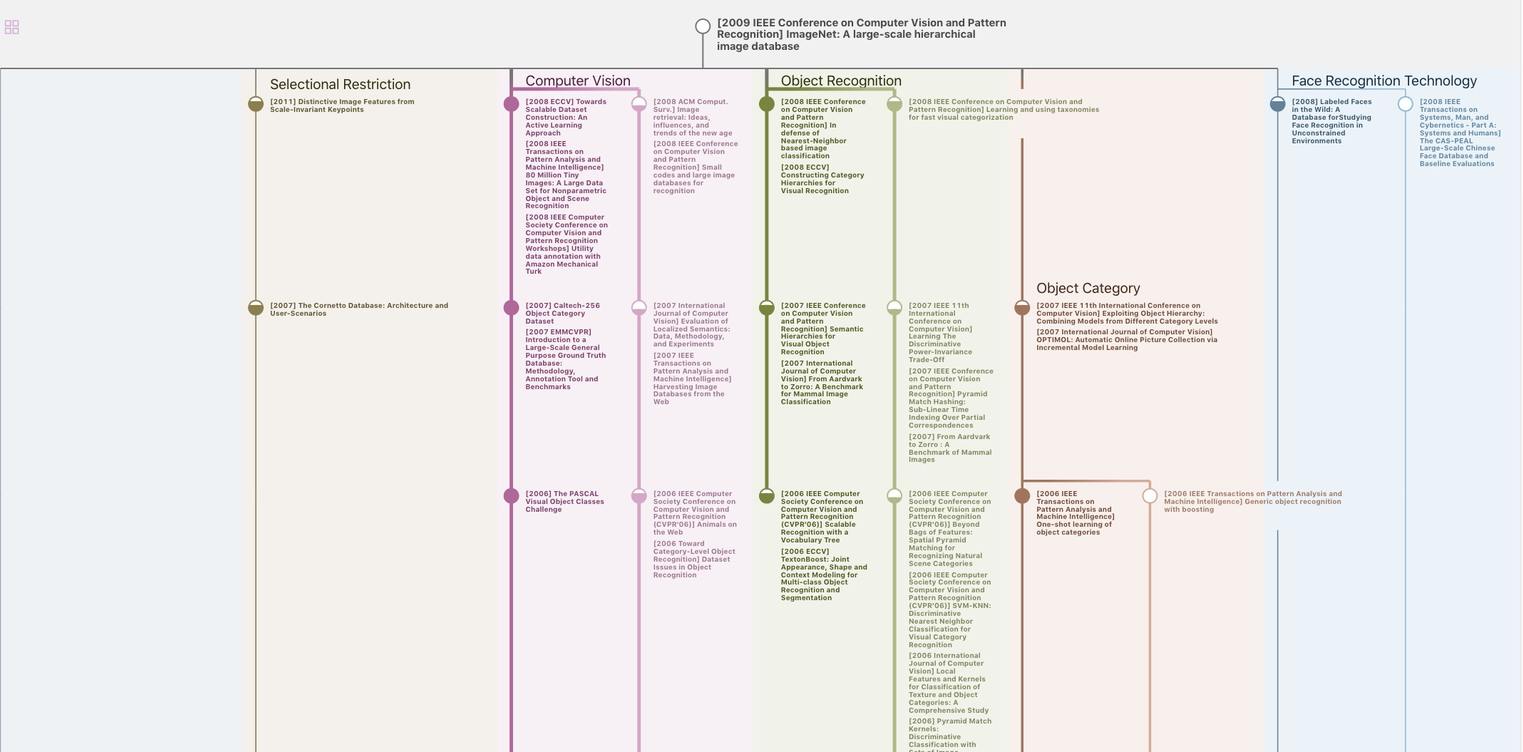
生成溯源树,研究论文发展脉络
Chat Paper
正在生成论文摘要