Probing the state of hydrogen in δ-AlOOH at mantle conditions with machine learning potential
Physical Review Research(2024)
摘要
Hydrous and nominally anhydrous minerals are a fundamental class of solids of enormous significance to geophysics. They are the water carriers in the deep geological water cycle and impact structural, elastic, plastic, and thermodynamic properties and phase relations in Earth's forming aggregates (rocks). They play a critical role in the geochemical and geophysical processes that shape the planet. Their complexity has prevented predictive calculations of their properties, but progress in materials simulations ushered by machine-learning potentials is transforming this state of affairs. Here, we adopt a hybrid approach that combines deep learning potentials (DPs) with the strongly constrained and appropriately normed meta-generalized gradient approximation functional to simulate a prototypical hydrous system. We illustrate the success of this approach to simulate δ-AlOOH (δ), a phase capable of transporting water down to near the core-mantle boundary of the Earth (∼2900km depth and ∼135GPa) in subducting slabs. A high-throughput sampling of phase space using molecular dynamics simulations with DPs sheds light on the hydrogen-bond behavior and proton diffusion at geophysical conditions. These simulations provide a pathway for a deeper understanding of these crucial components that shape Earth's internal state.
更多查看译文
AI 理解论文
溯源树
样例
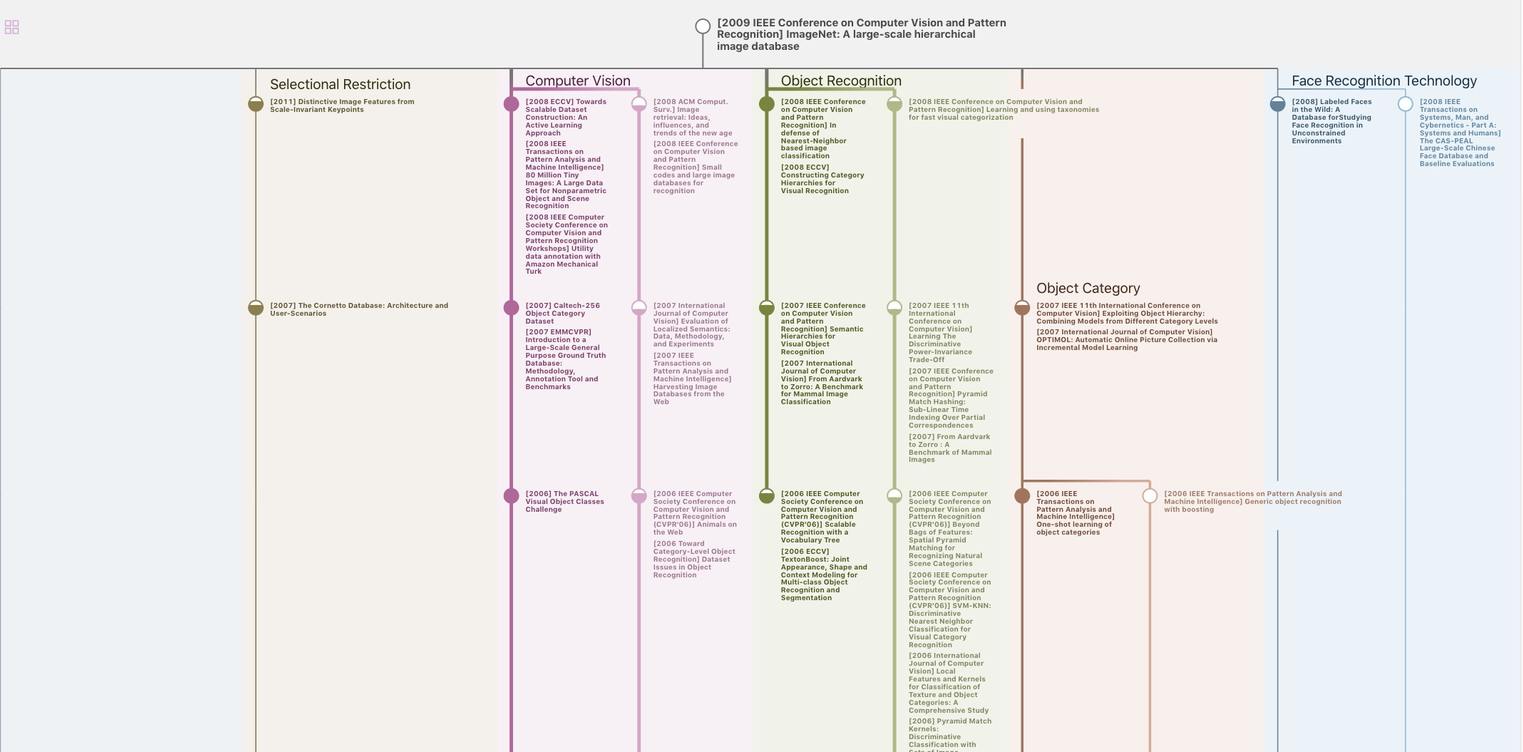
生成溯源树,研究论文发展脉络
Chat Paper
正在生成论文摘要