Learning Fine-Grained Information Alignment for Calibrated Cross-Modal Retrieval
ICASSP 2024 - 2024 IEEE International Conference on Acoustics, Speech and Signal Processing (ICASSP)(2024)
摘要
Masked Language Modeling (MLM) and Image-Text Matching (ITM) are always used in fusion encoder to learn the joint representation of images and text. In existing methods, the masking strategy of MLM leads to the neglect of image details during the modeling process. Meanwhile, the sampling strategy of ITM struggles to consistently select high-difficulty hard negative instances, reducing the effectiveness of constraints. This leads to challenges in aligning fine-grained information in cross-modal retrieval. In response to this challenge, a fine-grained information alignment-based visual language model (FAM) is proposed in this paper. On one hand, the attribute-based masking strategy is employed in MLM, helping the model focus on the details of objects in images during modeling. On the other hand, the robust hard negative sample generation strategy provides challenging negative samples for ITM by altering the relationships between objects. This enables the model to align relationships between objects in different modalities and thus calibrates cross-modal retrieval. Extensive experiments demonstrate the effectiveness of the model in cross-modal retrieval tasks.
更多查看译文
关键词
Cross-modal retrieval,fine-grained information,attribute-based masking,hard negative sample
AI 理解论文
溯源树
样例
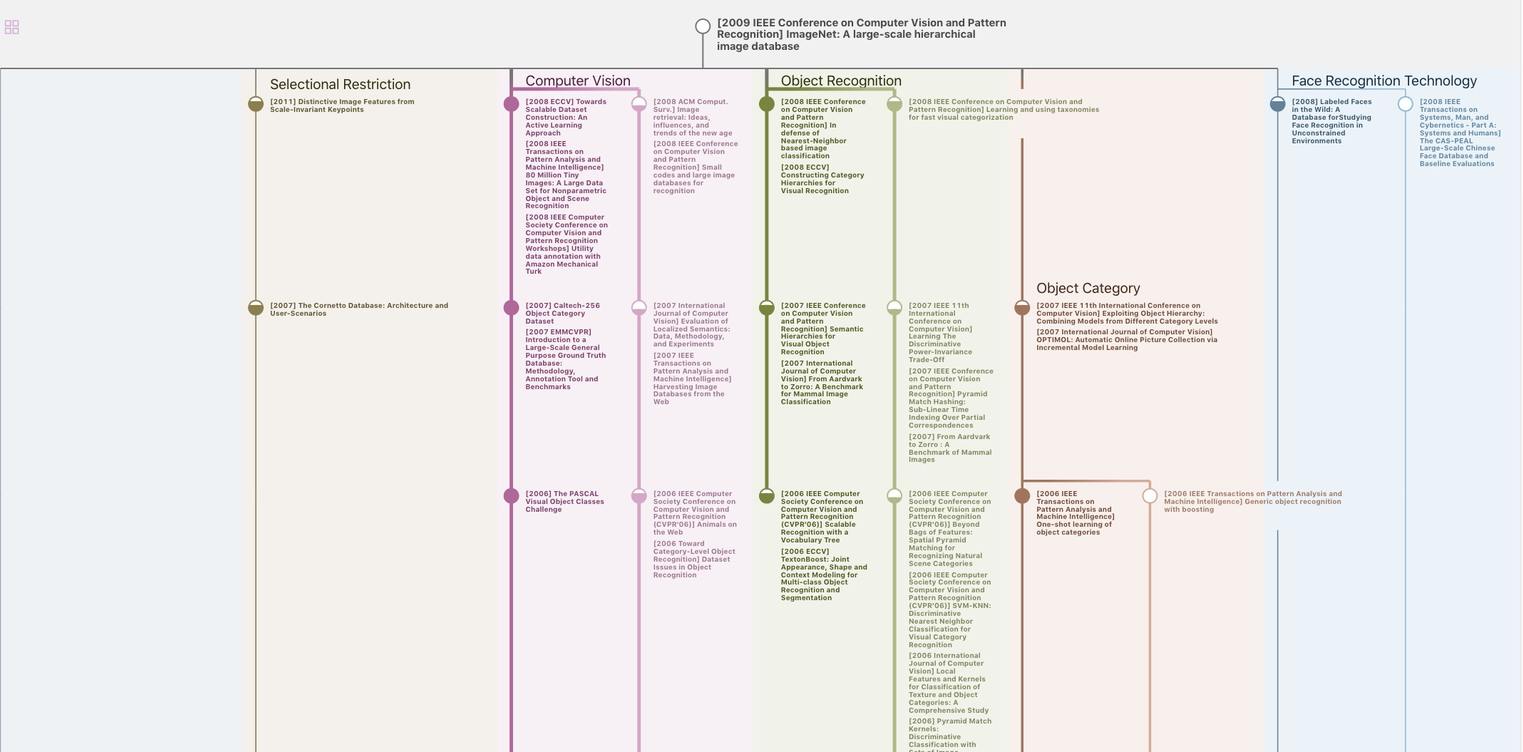
生成溯源树,研究论文发展脉络
Chat Paper
正在生成论文摘要