Decentralized Low Rank Matrix Recovery from Column-Wise Projections by Alternating GD and Minimization
ICASSP 2024 - 2024 IEEE International Conference on Acoustics, Speech and Signal Processing (ICASSP)(2024)
摘要
This work studies our recently developed algorithm, decentralized alternating projected gradient descent algorithm (Dec-AltGDmin), for recovering a low rank (LR) matrix from independent column-wise linear projections in a decentralized setting. This means that the observed data is spread across L agents and there is no central coordinating node. Since this problem is non-convex and since it involves a subspace recovery step, most existing literature from decentralized optimization is not useful. We demonstrate using extensive numerical simulations and communication, time, and sample complexity comparisons that (i) existing decentralized gradient descent (GD) approaches fail, and (ii) other common solution approaches on LR recovery literature – projected GD, alternating GD and alternating minimization (AltMin) – either have a higher communication (and time) complexity or a higher sample complexity. Communication complexity is often the most important concern in decentralized learning.
更多查看译文
关键词
Low Rank matrix recovery,compressed sensing,decentralized algorithms
AI 理解论文
溯源树
样例
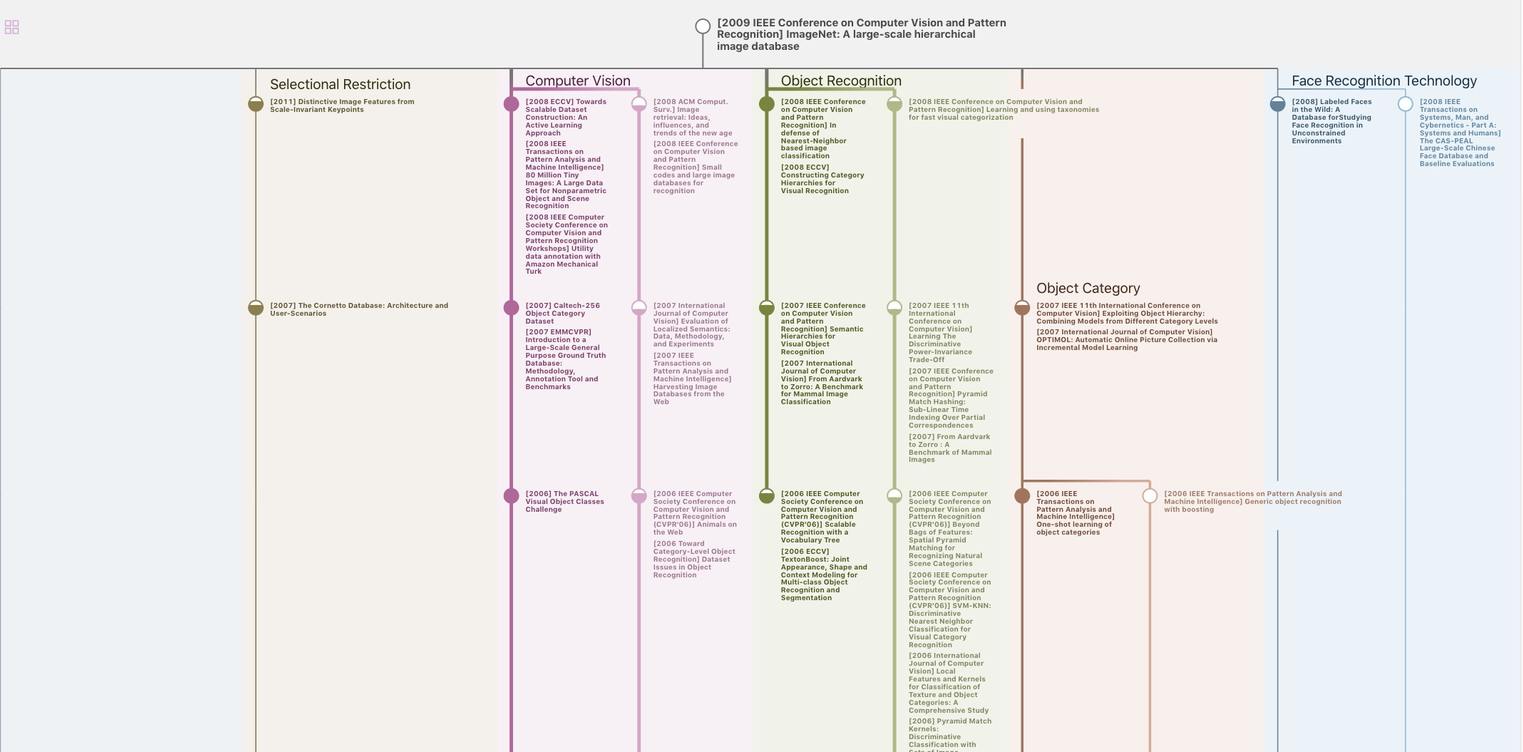
生成溯源树,研究论文发展脉络
Chat Paper
正在生成论文摘要