Gaussian universality for approximately polynomial functions of high-dimensional data
arxiv(2024)
摘要
We establish an invariance principle for polynomial functions of n
independent high-dimensional random vectors, and also show that the obtained
rates are nearly optimal. Both the dimension of the vectors and the degree of
the polynomial are permitted to grow with n. Specifically, we obtain a finite
sample upper bound for the error of approximation by a polynomial of Gaussians,
measured in Kolmogorov distance, and extend it to functions that are
approximately polynomial in a mean squared error sense. We give a corresponding
lower bound that shows the invariance principle holds up to polynomial degree
o(log n). The proof is constructive and adapts an asymmetrisation argument
due to V. V. Senatov. As applications, we obtain a higher-order delta method
with possibly non-Gaussian limits, and generalise a number of known results on
high-dimensional and infinite-order U-statistics, and on fluctuations of
subgraph counts.
更多查看译文
AI 理解论文
溯源树
样例
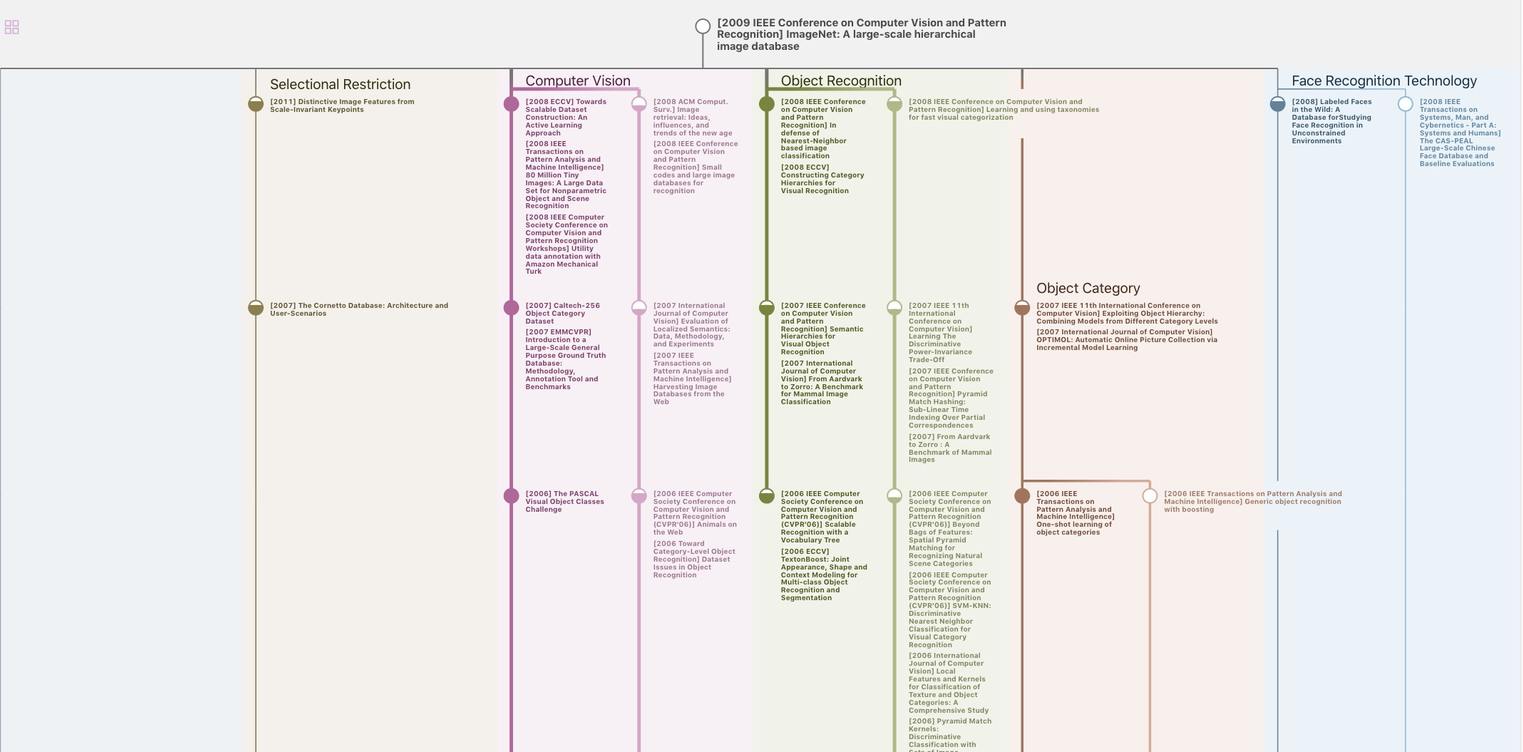
生成溯源树,研究论文发展脉络
Chat Paper
正在生成论文摘要