A Deep Learning Method for Beat-Level Risk Analysis and Interpretation of Atrial Fibrillation Patients during Sinus Rhythm
arxiv(2024)
摘要
Atrial Fibrillation (AF) is a common cardiac arrhythmia. Many AF patients
experience complications such as stroke and other cardiovascular issues. Early
detection of AF is crucial. Existing algorithms can only distinguish “AF
rhythm in AF patients” from “sinus rhythm in normal individuals” . However,
AF patients do not always exhibit AF rhythm, posing a challenge for diagnosis
when the AF rhythm is absent. To address this, this paper proposes a novel
artificial intelligence (AI) algorithm to distinguish “sinus rhythm in AF
patients” and “sinus rhythm in normal individuals” in beat-level. We
introduce beat-level risk interpreters, trend risk interpreters, addressing the
interpretability issues of deep learning models and the difficulty in
explaining AF risk trends. Additionally, the beat-level information fusion
decision is presented to enhance model accuracy. The experimental results
demonstrate that the average AUC for single beats used as testing data from
CPSC 2021 dataset is 0.7314. By employing 150 beats for information fusion
decision algorithm, the average AUC can reach 0.7591. Compared to previous
segment-level algorithms, we utilized beats as input, reducing data
dimensionality and making the model more lightweight, facilitating deployment
on portable medical devices. Furthermore, we draw new and interesting findings
through average beat analysis and subgroup analysis, considering varying risk
levels.
更多查看译文
AI 理解论文
溯源树
样例
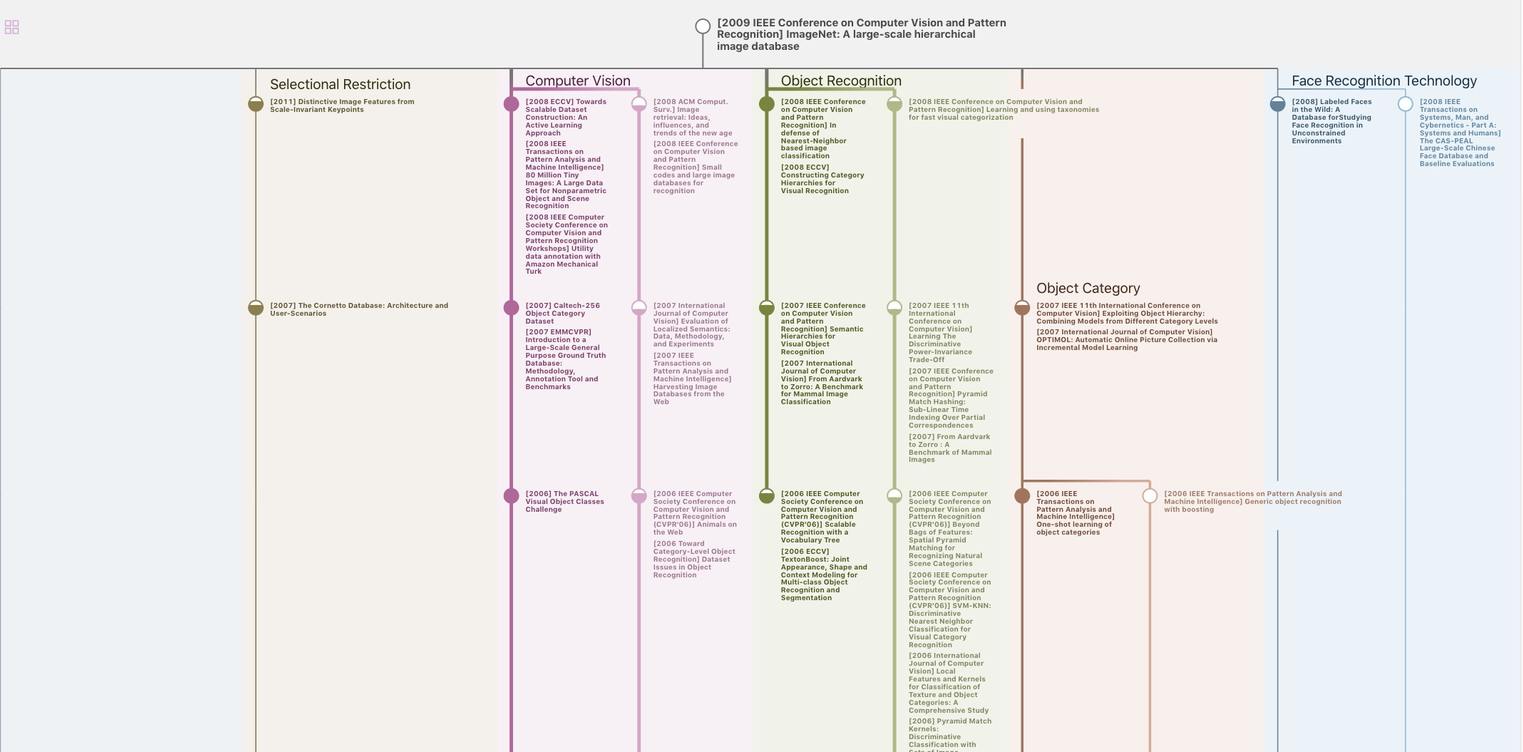
生成溯源树,研究论文发展脉络
Chat Paper
正在生成论文摘要