Generative Motion Stylization within Canonical Motion Space
arxiv(2024)
摘要
Stylized motion breathes life into characters. However, the fixed skeleton
structure and style representation hinder existing data-driven motion synthesis
methods from generating stylized motion for various characters. In this work,
we propose a generative motion stylization pipeline, named MotionS, for
synthesizing diverse and stylized motion on cross-structure characters using
cross-modality style prompts. Our key insight is to embed motion style into a
cross-modality latent space and perceive the cross-structure skeleton
topologies, allowing for motion stylization within a canonical motion space.
Specifically, the large-scale Contrastive-Language-Image-Pre-training (CLIP)
model is leveraged to construct the cross-modality latent space, enabling
flexible style representation within this space. Additionally, two
topology-encoded tokens are learned to capture the canonical and specific
skeleton topologies, facilitating cross-structure topology shifting.
Subsequently, the topology-shifted stylization diffusion is designed to
generate motion content for the specific skeleton and stylize it in the shifted
canonical motion space using multi-modality style descriptions. Through an
extensive set of examples, we demonstrate the flexibility and generalizability
of our pipeline across various characters and style descriptions. Qualitative
and quantitative experiments underscore the superiority of our pipeline over
state-of-the-art methods, consistently delivering high-quality stylized motion
across a broad spectrum of skeletal structures.
更多查看译文
AI 理解论文
溯源树
样例
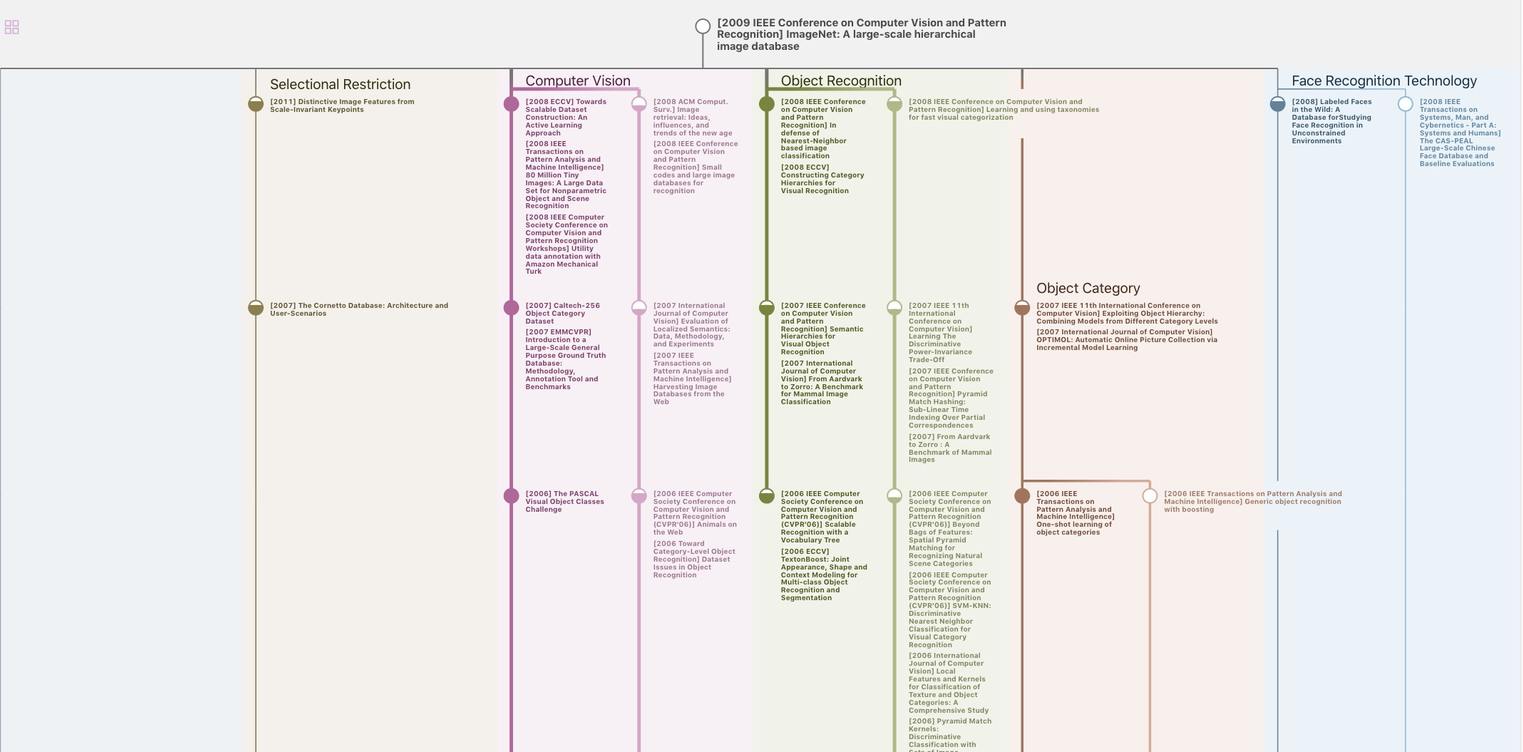
生成溯源树,研究论文发展脉络
Chat Paper
正在生成论文摘要