Non-iterative Pyramid Network for Unsupervised Deformable Medical Image Registration.
IEEE International Conference on Acoustics, Speech, and Signal Processing(2024)
摘要
Large deformation is a key issue for deformable medical image registration. Decomposing a large deformation into several small deformations is an efficient solution. Current decomposition methods can be classed into iteration-based and non-iterative-based approaches. However, compared to iteration-based methods, non-iterative-based methods have faster inference times but registration accuracy gaps. To alleviate this limitation, we design a novel Non-iterative Pyramid Network (NIPNet). Firstly, Our Dual-domain Feature Extraction Module (DFEM) extracts global and local features in the frequency and spatial domains, respectively. Hence, the model considers global and local deformations. Secondly, a Multi-scale Localization Information Fusion Module (MIFM) is applied to fuse the localization information of adjacent scales to assist the current level in obtaining a more accurate deformation field. Finally, a Pyramid Self-distillation Loss (PDL) is introduced to improve the registration accuracy by treating the final deformation field as a teacher to guide the intermediate deformation field. By conducting intensive experiments on two typical 3D brain MRI datasets, we verify that the proposed NIPNet outperforms SOTA iterative-based methods and requires only a similar runtime as non-iterative approaches. Code is available at https://github.com/JXT210/NIPNet.
更多查看译文
关键词
Medical Image Registration,Non-iterative,Pyramid Network
AI 理解论文
溯源树
样例
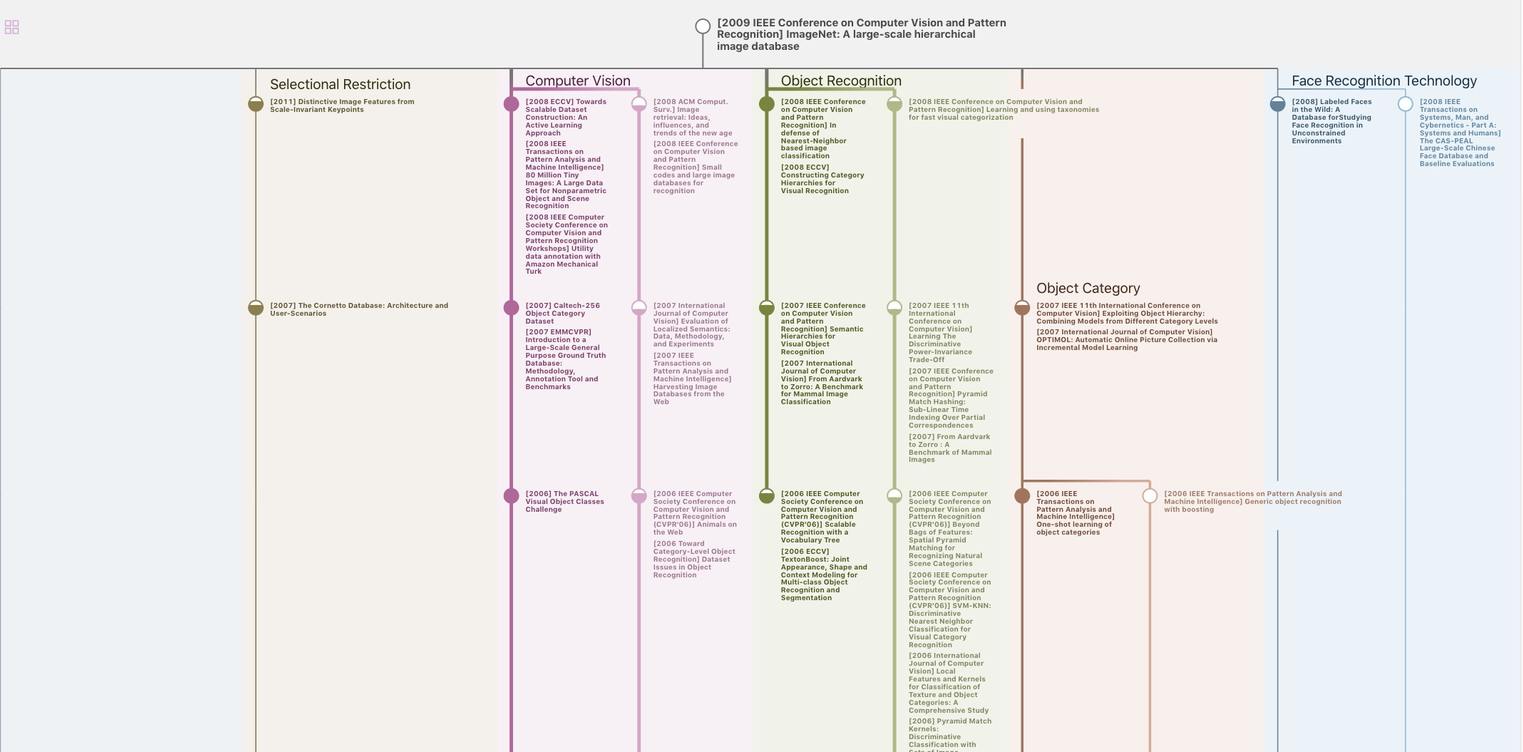
生成溯源树,研究论文发展脉络
Chat Paper
正在生成论文摘要