TA2P: Task-Aware Adaptive Pruning Method for Image Classification on Edge Devices
IEEE International Conference on Acoustics, Speech, and Signal Processing(2024)
摘要
Convolutional neural networks (CNNs) have been extensively used in image classification. However, it is still challenging to directly deploy them on edge devices because of high computation requirements. To solve this problem, many pruning schemes for neural networks have been proposed. However, these methods ignore the fact that most edge devices only need to handle some instead of the whole classes. If the classification of several classes is defined as one task, then task-level information could be utilized to further lighten CNNs. To this end, we propose such a task-aware adaptive model pruning method in this paper. Specifically, we first employ control gates to learn weights of each convolutional channel of each class, and then merge class-level control gates through linear combinations to obtain the pruned model for an adaptive task on edge devices. Experiments demonstrate that our proposed method could significantly reduce the number of parameters up to 33.3% and 13.5% on CIFAR-10 and CIFAR-100, while achieving almost the same accuracy compared with baseline methods.
更多查看译文
关键词
Model compression,channel pruning,edge computing,task aware
AI 理解论文
溯源树
样例
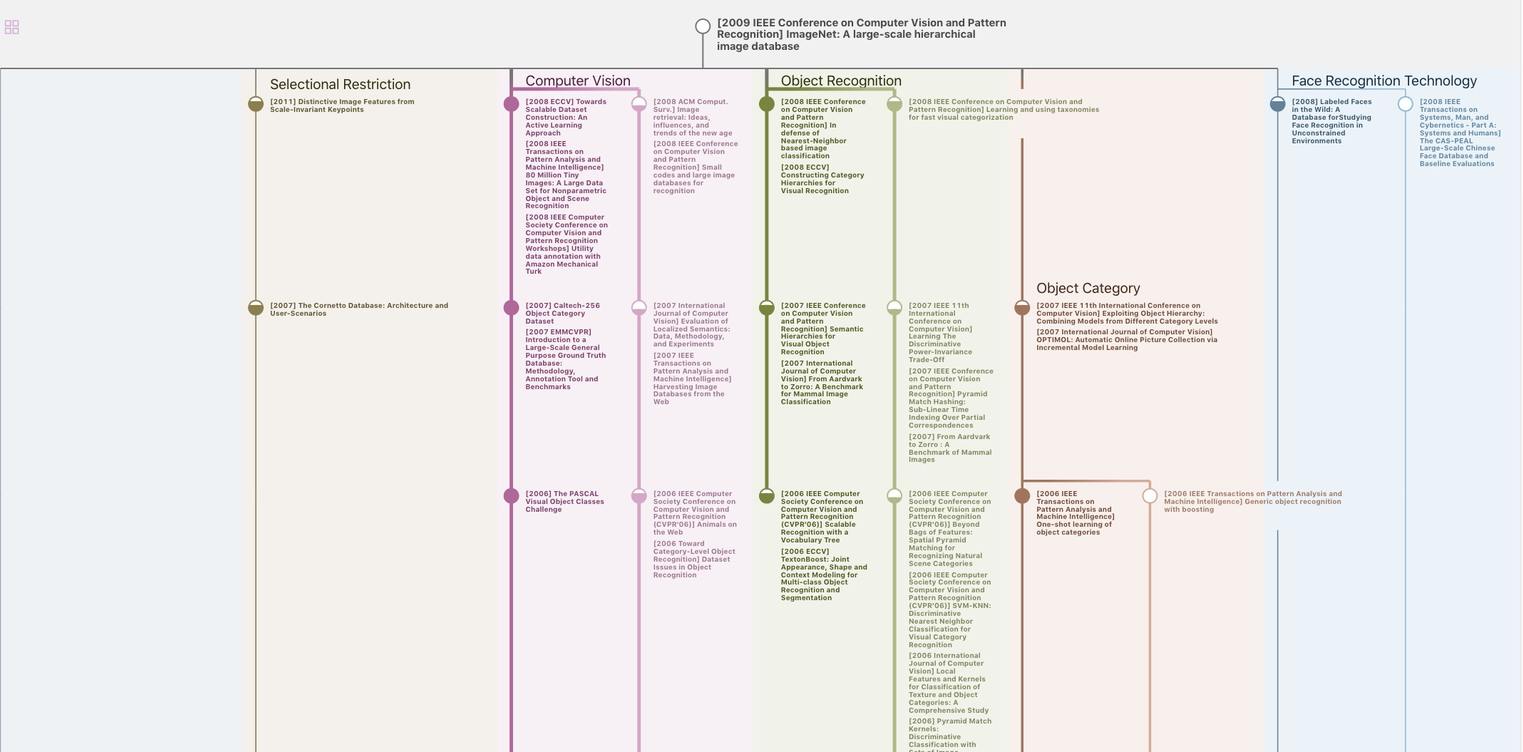
生成溯源树,研究论文发展脉络
Chat Paper
正在生成论文摘要