Symmetric Consistency with Cross-Domain Mixup for Cross-Modality Cardiac Segmentation
ICASSP 2024 - 2024 IEEE International Conference on Acoustics, Speech and Signal Processing (ICASSP)(2024)
摘要
Accurate cardiac segmentation in cross-modality images plays an important role in the quantitative analysis of the heart to diagnose cardiovascular diseases. However, achieving high performance in cross-modality segmentation is hindered by the time-consuming annotation and modality gap. While some approaches employ Unsupervised Domain Adaptation (UDA) through adversarial learning to address the issue, it still remains challenging due to the instability of the adversarial generative models. In this work, we propose Symmetric Consistency with Cross-Domain Mixup (SCCDM), integrated with the teacher-student model for cross-modality cardiac segmentation. Specifically, we introduce symmetric consistency across the domains for two mixed data to diversify the data distribution from both the source domain and target domain. Extensive experiments on a public cardiac dataset demonstrate that SCCDM achieves superior domain adaptation performance for cardiac segmentation compared to state-of-the-art methods.
更多查看译文
关键词
Mixup,Cross-modality,Cardiac Segmentation,Unsupervised Domain Adaptation
AI 理解论文
溯源树
样例
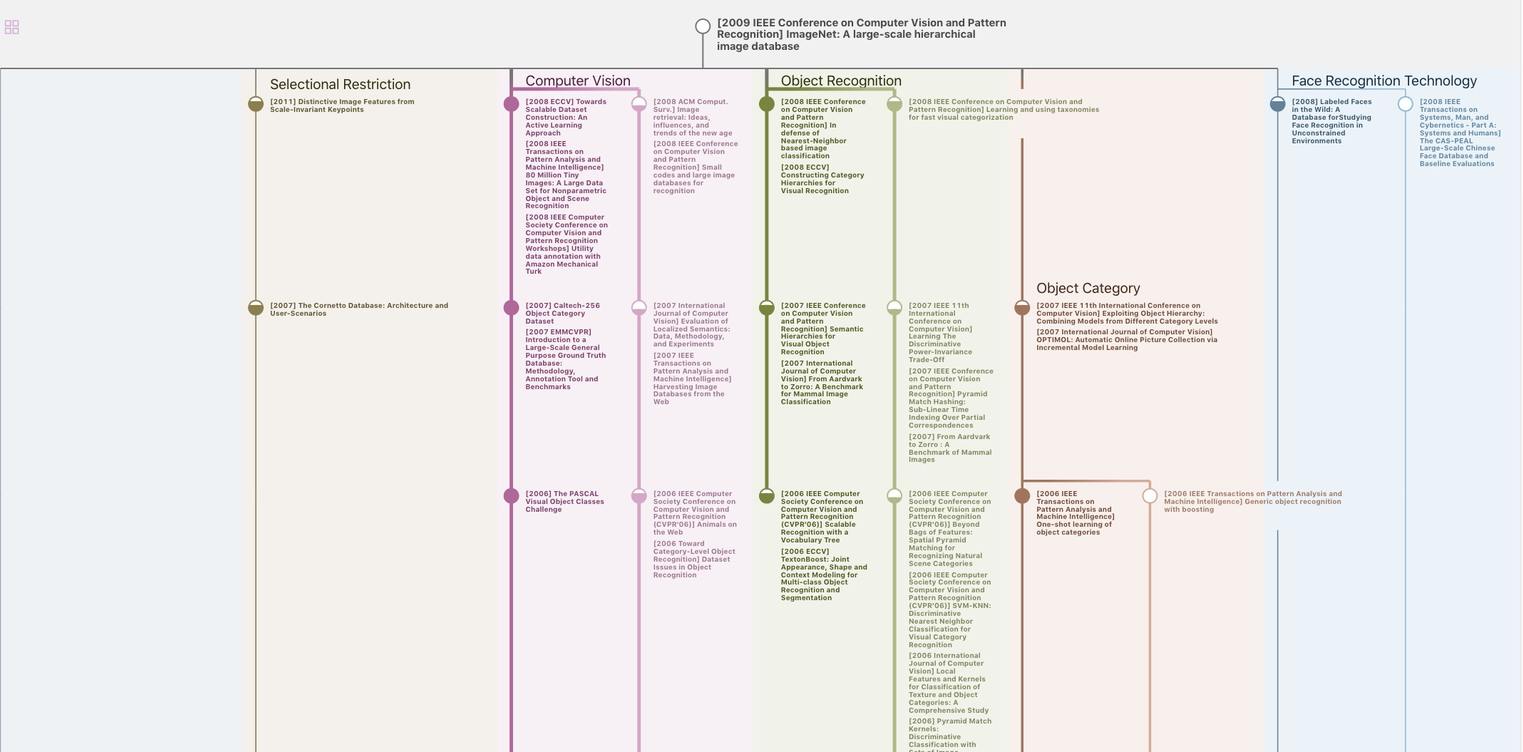
生成溯源树,研究论文发展脉络
Chat Paper
正在生成论文摘要