When Training-Free Nas Meets Vision Transformers: A Neural Tangent Kernel Perspective
ICASSP 2024 - 2024 IEEE International Conference on Acoustics, Speech and Signal Processing (ICASSP)(2024)
摘要
This paper investigates the Neural Tangent Kernel (NTK) to search vision transformers without training. In contrast with the previous observation that NTK-based metrics can effectively predict CNN’s performance at initialization, we empirically show their inefficacy in the ViT search space. We hypothesize that the fundamental feature learning preference within ViT contributes to the ineffectiveness of applying NTK to NAS for ViT. We both theoretically and empirically validate that NTK essentially estimates the ability of neural networks that learn low-frequency signals, completely ignoring the impact of high-frequency signals in feature learning. To address this limitation, we propose a new method called ViNTK that generalizes the standard NTK to the high-frequency domain by integrating the Fourier features from inputs. Experiments with multiple ViT search spaces on image classification and semantic segmentation tasks show that our method can significantly speed up search costs over prior state-of-the-art NAS for ViT while maintaining similar performance on searched architectures.
更多查看译文
关键词
Neural Architecture Search,Vision Transformer
AI 理解论文
溯源树
样例
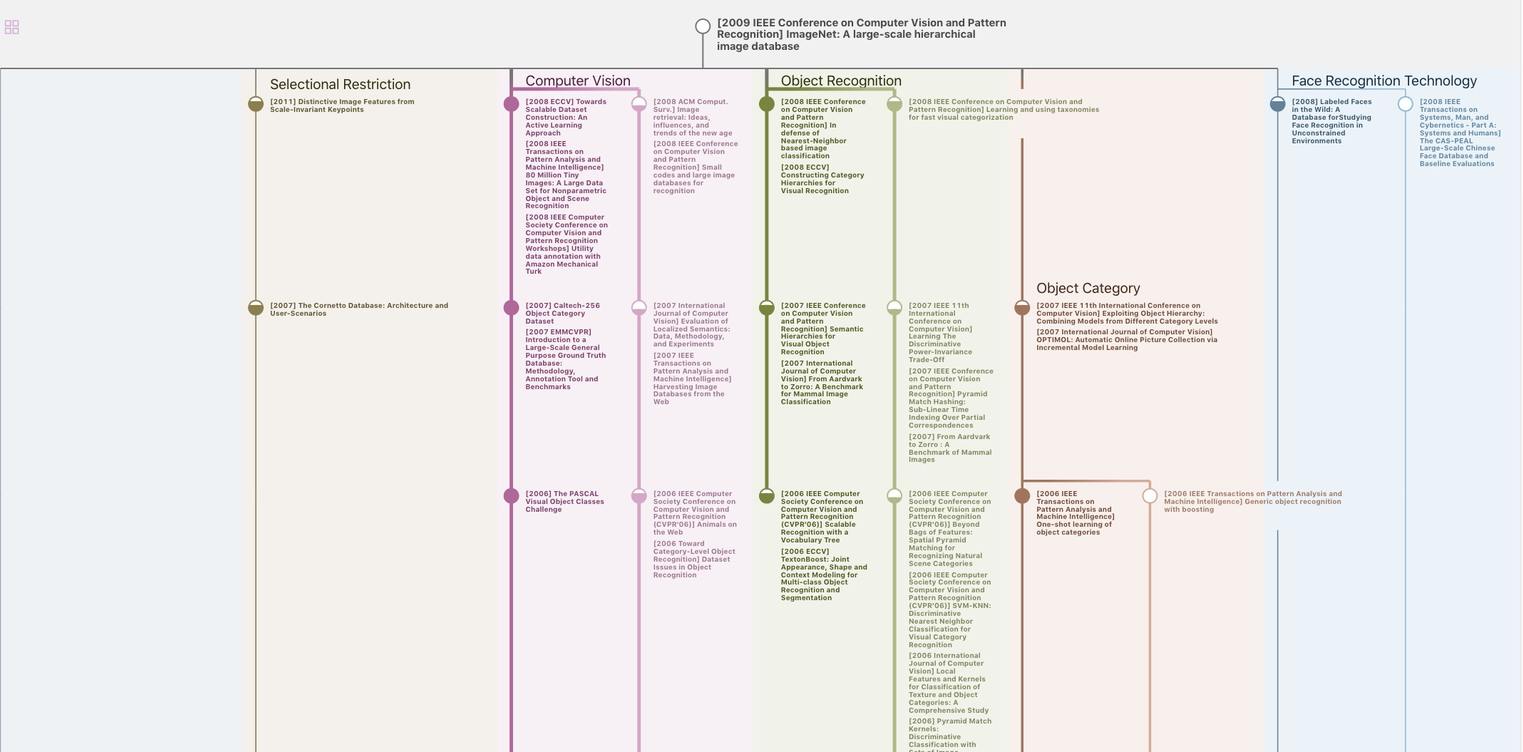
生成溯源树,研究论文发展脉络
Chat Paper
正在生成论文摘要