Low-Rank Completion Based Normal Guided Lidar Point Cloud Up-Sampling.
IEEE International Conference on Acoustics, Speech, and Signal Processing(2024)
摘要
Commercial inexpensive LiDAR sensor generally suffers low vertical resolutions, whose point cloud is sparse and may not be able to satisfy future metaverse applications. LiDAR point cloud up-sampling is a task to increase the vertical resolution while preserving the structural details. Scene representation is the central pillar of point cloud up-sampling. However, the sparsity of point cloud hinders the extraction of scene representation. In this paper, we find that low-rank representation can describe the primary scene structure approximately, and convert up-sampling as low-rank tensor completion problem. To decrease problem complexity, we leverage range view projection to convert the problem as low-rank depth completion, and present a low-rank normal guided up-sampling approach. It uses normal as guidance to smooth range depth. Extensive experiments show that our method outperforms current methods. In 2× up-sampling task, it achieves as low as 41cm of mean absolute error (MAE), which is 282% and 32% smaller than interpolation and traditional matrix completion methods, respectively. Hence, we believe the proposed method benefits to the field of metaverse.
更多查看译文
关键词
LiDAR sensor,point cloud up-sampling,low-rank representation,depth completion,metaverse
AI 理解论文
溯源树
样例
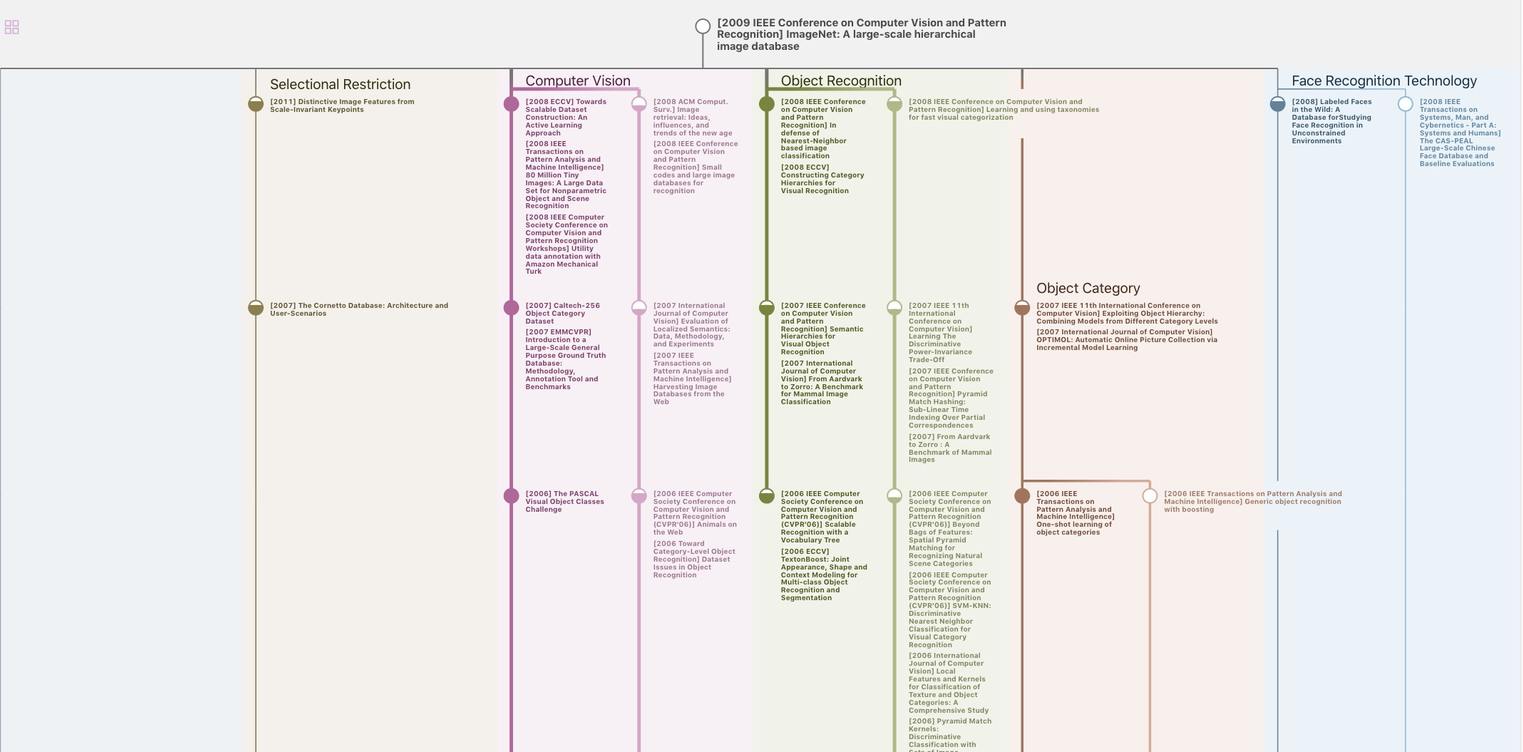
生成溯源树,研究论文发展脉络
Chat Paper
正在生成论文摘要