Driver Scanpath Prediction Based On Inverse Reinforcement Learning
ICASSP 2024 - 2024 IEEE International Conference on Acoustics, Speech and Signal Processing (ICASSP)(2024)
摘要
Modeling driver attention allocation by scanpath prediction plays a crucial role in advancing autonomous driving capabilities and enhancing accident anticipation. Existing studies primarily predict human scanpath for visual search, visual question answering, and free-viewing. Few studies have focused on predicting scanpath in driving scenarios. To address these limitations, we propose a novel inverse reinforcement learning-based approach through adversarial learning to effectively anticipate human-like scanpaths within different driving tasks. Particularly, we introduce a Transformer-based architecture to construct the generator and discriminator models, while integrating top-down, bottom-up, and historical information for dynamic state updated through State-Encode-with-Attention (SEA). Inspired by the human visual system, SEA adopts a fovea-like movement strategy. Experimental results on the benchmark dataset of BDD-X-diverse validate the effectiveness of our proposed method.
更多查看译文
关键词
Scanpath prediction,visual attention,inverse reinforcement learning,autonomous driving
AI 理解论文
溯源树
样例
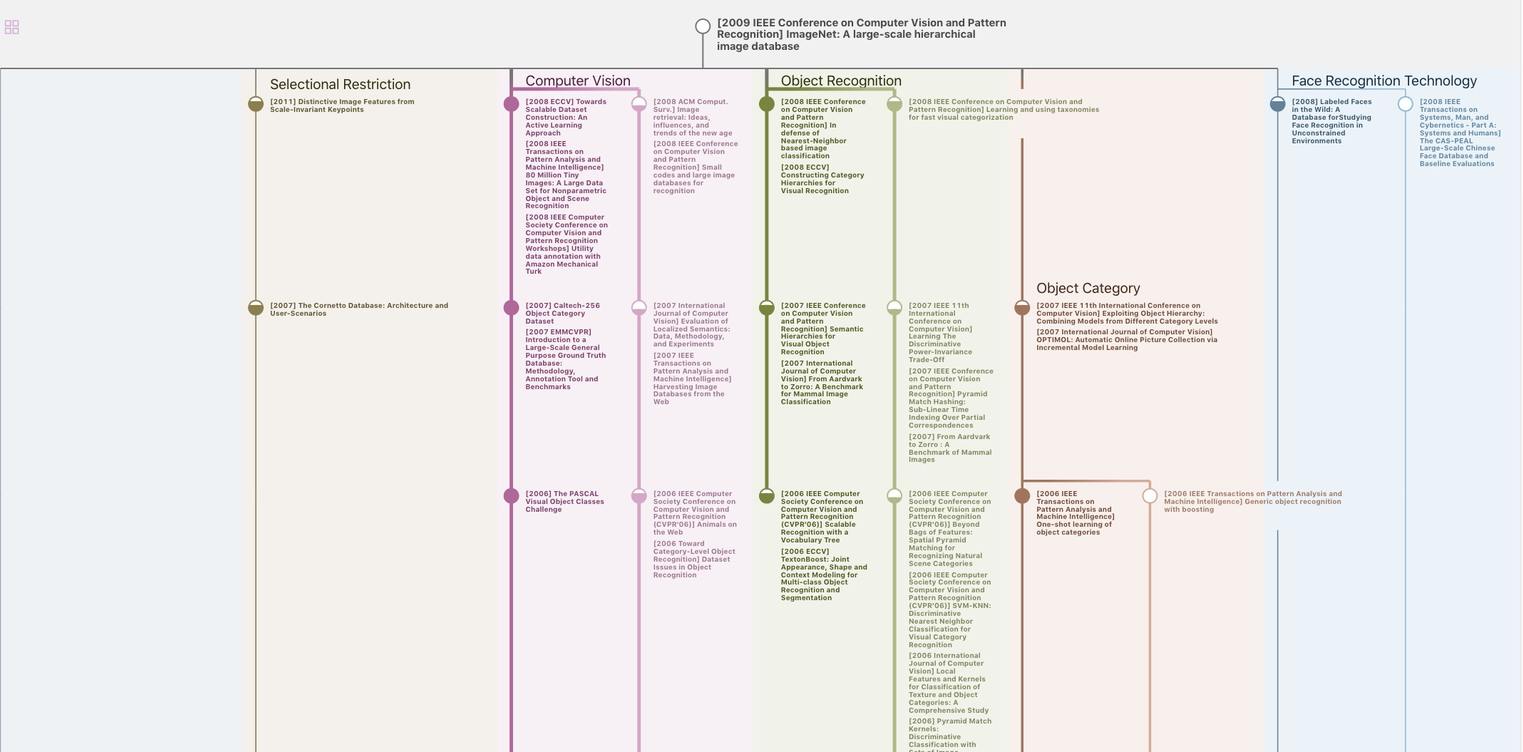
生成溯源树,研究论文发展脉络
Chat Paper
正在生成论文摘要