Patch-Wise Augmentation for Anomaly Detection and Localization
ICASSP 2024 - 2024 IEEE International Conference on Acoustics, Speech and Signal Processing (ICASSP)(2024)
摘要
Existing visual anomaly detection (AD) methods typically train reconstruction models by relying on normal images, while anomalous regions would not be well-recovered and hence can be localized with complicated post-processing steps during inference. In this paper, we formulate unsupervised AD as a supervised object detection task. To create supervision signals, we build a patch-wise data augmentation strategy called PatchAnomaly, to synthesize anomaly-like images based on self-supervised learning. Then, we propose a reconstruction-detection model to directly localize anomalous regions under supervision signals derived from PatchAnomaly. Experiments on the MVTecAD and BTAD datasets demonstrate competitive performance, achieving image-level AUROC scores of 98.4% and 95.5% respectively.
更多查看译文
关键词
Anomaly detection,Self-supervised learning,Data augmentation,Object detection,Feature learning
AI 理解论文
溯源树
样例
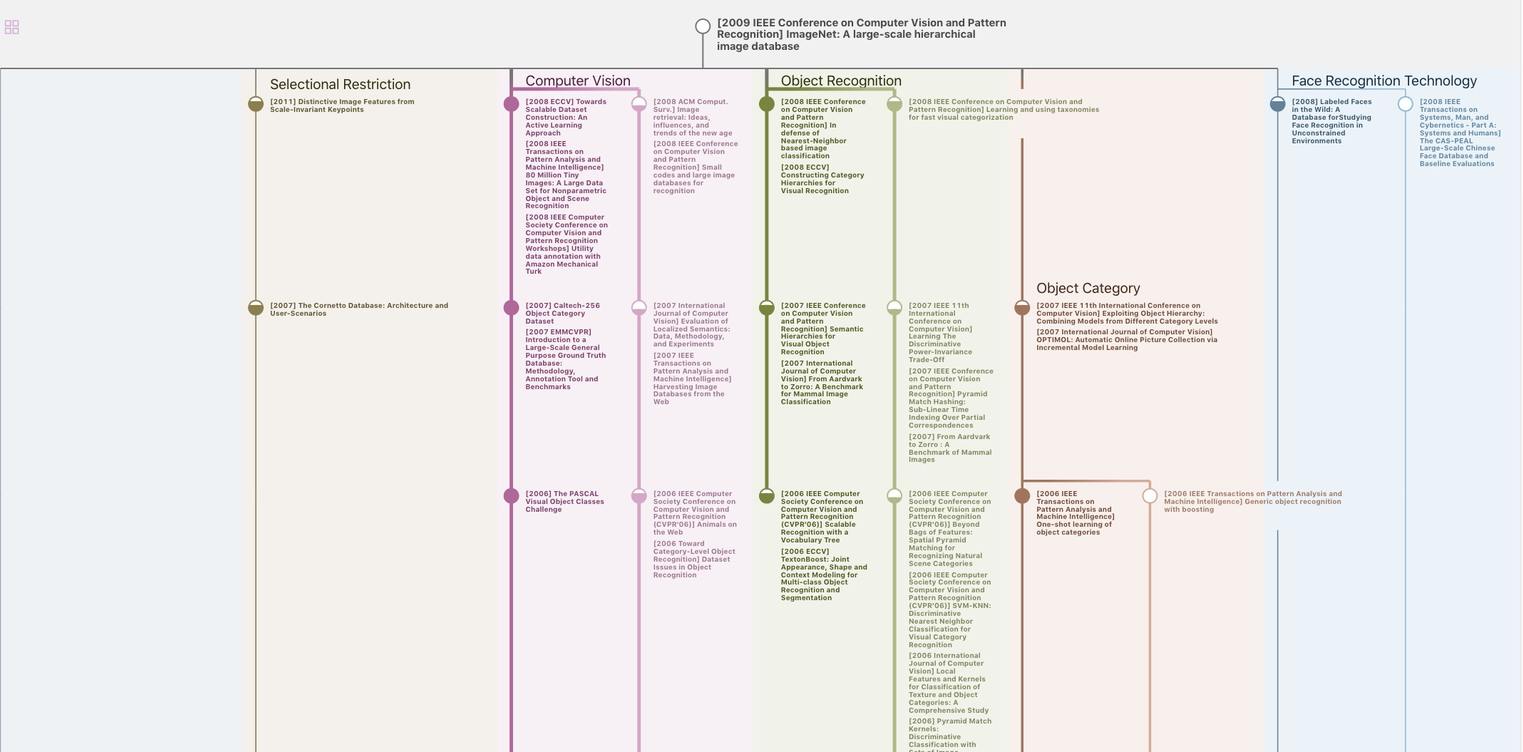
生成溯源树,研究论文发展脉络
Chat Paper
正在生成论文摘要