Learning Discriminative Style Representations for Unsupervised and Few-Shot Artistic Portrait Drawing Generation
ICASSP 2024 - 2024 IEEE International Conference on Acoustics, Speech and Signal Processing (ICASSP)(2024)
摘要
In this paper, we propose an unsupervised artistic portrait drawing generation method for few-shot datasets based on contrastive learning of style features. Firstly, we construct a discriminative style encoder with contrastive learning, improving the ability of the encoder to separate style features. Secondly, based on the dynamic codebook and momentum network, we used historical average features instead of batch instance features to prevent the problem of style bias in few-shot datasets. Finally, a conditional projection discriminator with filter response normalization is utilized to improve the discriminative ability of the discriminator and the stability of the generative adversarial network, which motivates the generator to synthesize more realistic image details. Quantitative and qualitative analysis show that the method proposed in this paper significantly improves the quality of artistic portrait drawing generation, and outperforms existing benchmarks in terms of visual effect and metrics evaluation. Our code and results are avilable at https://github.com/AiArt-HDU/Co-GAN.
更多查看译文
关键词
artistic portrait drawing generation,face sketch synthesis,few-shot learning,image-to-image translation,contrastive learning
AI 理解论文
溯源树
样例
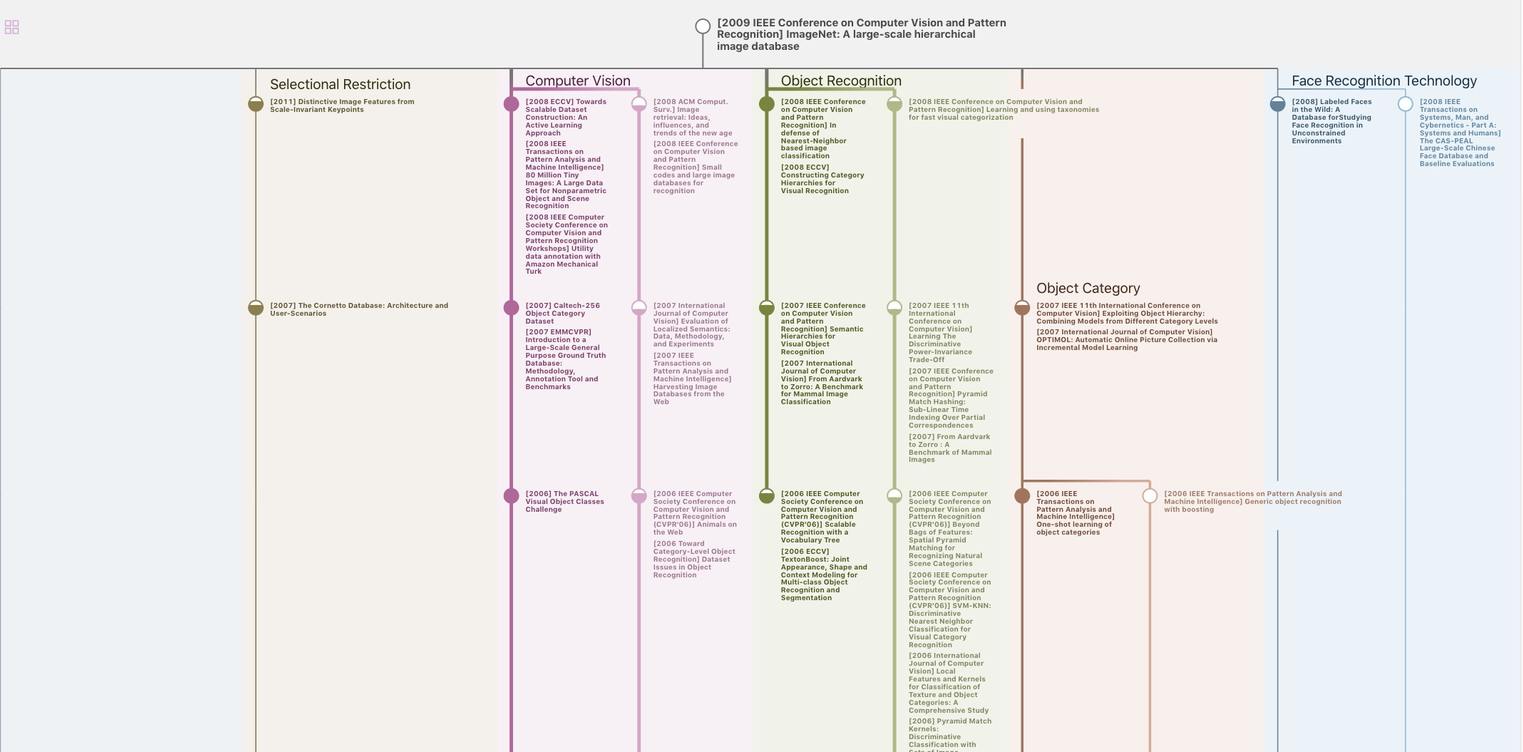
生成溯源树,研究论文发展脉络
Chat Paper
正在生成论文摘要